Identifying concerns when specifying machine learning-enabled systems: A perspective-based approach
Journal of Systems and Software(2024)
摘要
Engineering successful machine learning (ML)-enabled systems poses various challenges from both a theoretical and a practical side. Among those challenges are how to effectively address unrealistic expectations of ML capabilities from customers, managers and even other team members, and how to connect business value to engineering and data science activities composed by interdisciplinary teams. In this paper, we present PerSpecML, a perspective-based approach for specifying ML-enabled systems that helps practitioners identify which attributes, including ML and non-ML components, are important to contribute to the overall system’s quality. The approach involves analyzing 60 concerns related to typical tasks that practitioners face in ML projects, grouping them into five perspectives: system objectives, user experience, infrastructure, model, and data. Together, these perspectives serve to mediate the communication between business owners, domain experts, designers, software and ML engineers, and data scientists. The creation of PerSpecML involved a series of formative evaluations conducted in different contexts: (i) in academia, (ii) with industry representatives, and (iii) in two real industrial case studies. As a result of the diverse validations and continuous improvements, PerSpecML stands as a promising approach, poised to positively impact the specification of ML-enabled systems, particularly helping to reveal key components that would have been otherwise missed without using PerSpecML.Editor’s note: Open Science material was validated by the Journal of Systems and Software Open Science Board.
更多查看译文
关键词
Requirements engineering,Machine learning-enabled systems,Technology transfer,Case study
AI 理解论文
溯源树
样例
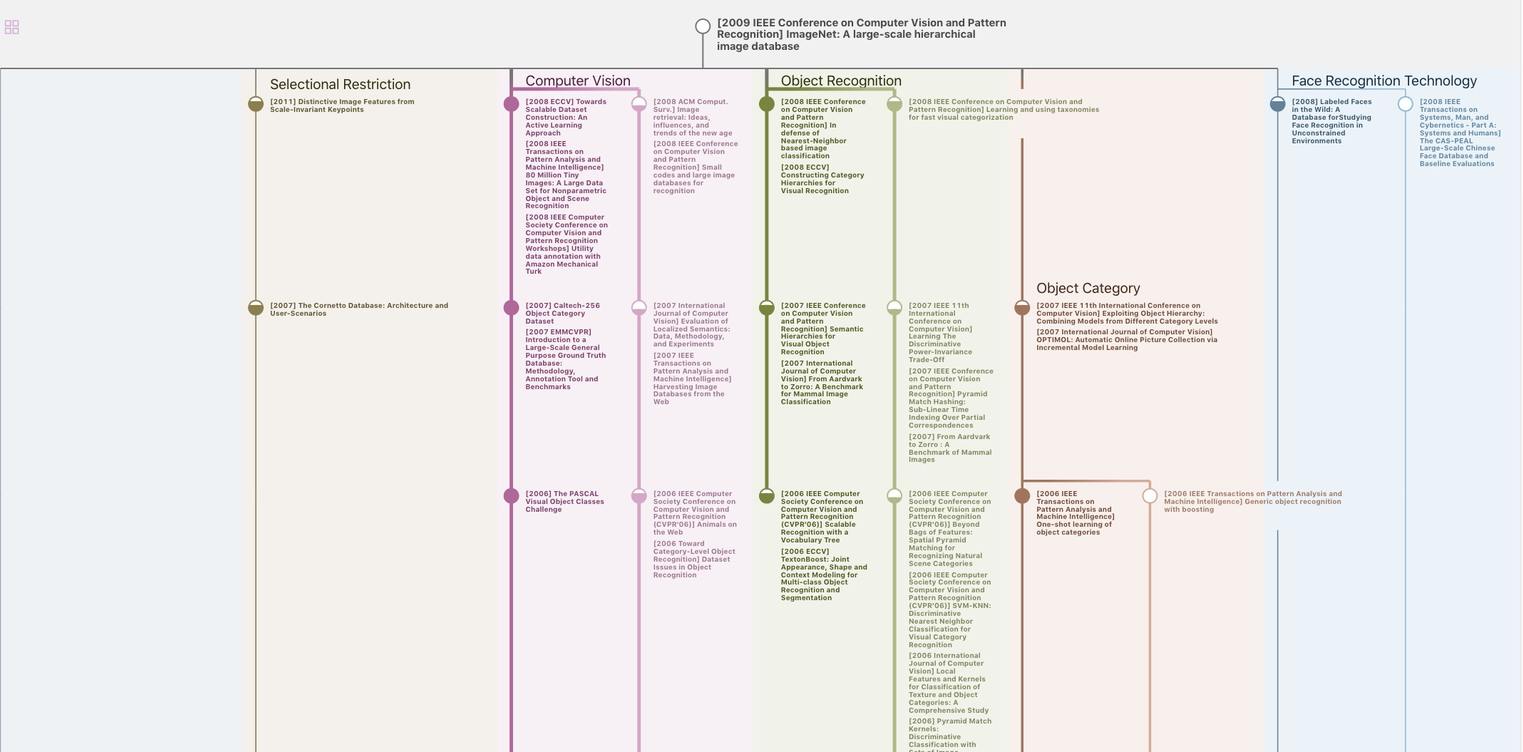
生成溯源树,研究论文发展脉络
Chat Paper
正在生成论文摘要