Restructure-Tolerant Timing Prediction via Multimodal Fusion
2023 60th ACM/IEEE Design Automation Conference (DAC)(2023)
摘要
Fast and accurate pre-routing timing prediction is crucial in the very-large-scale integration (VLSI) design flow. Existing machine learning (ML)-assisted pre-routing timing evaluators neglect the impact of timing optimization, which may render their approaches impractical in real circuit design flows. To model the impact of timing optimization, we propose an endpoint embedding framework that integrates netlist-layout information via multimodal fusion. An end-to-end flow is further developed for pre-routing restructure-tolerant prediction on global timing metrics. Comprehensive experiments on large-scale RISC-V designs with advanced 7-nm technology node demonstrate the superiority of our model compared to the SOTA pre-routing timing evaluators.
更多查看译文
AI 理解论文
溯源树
样例
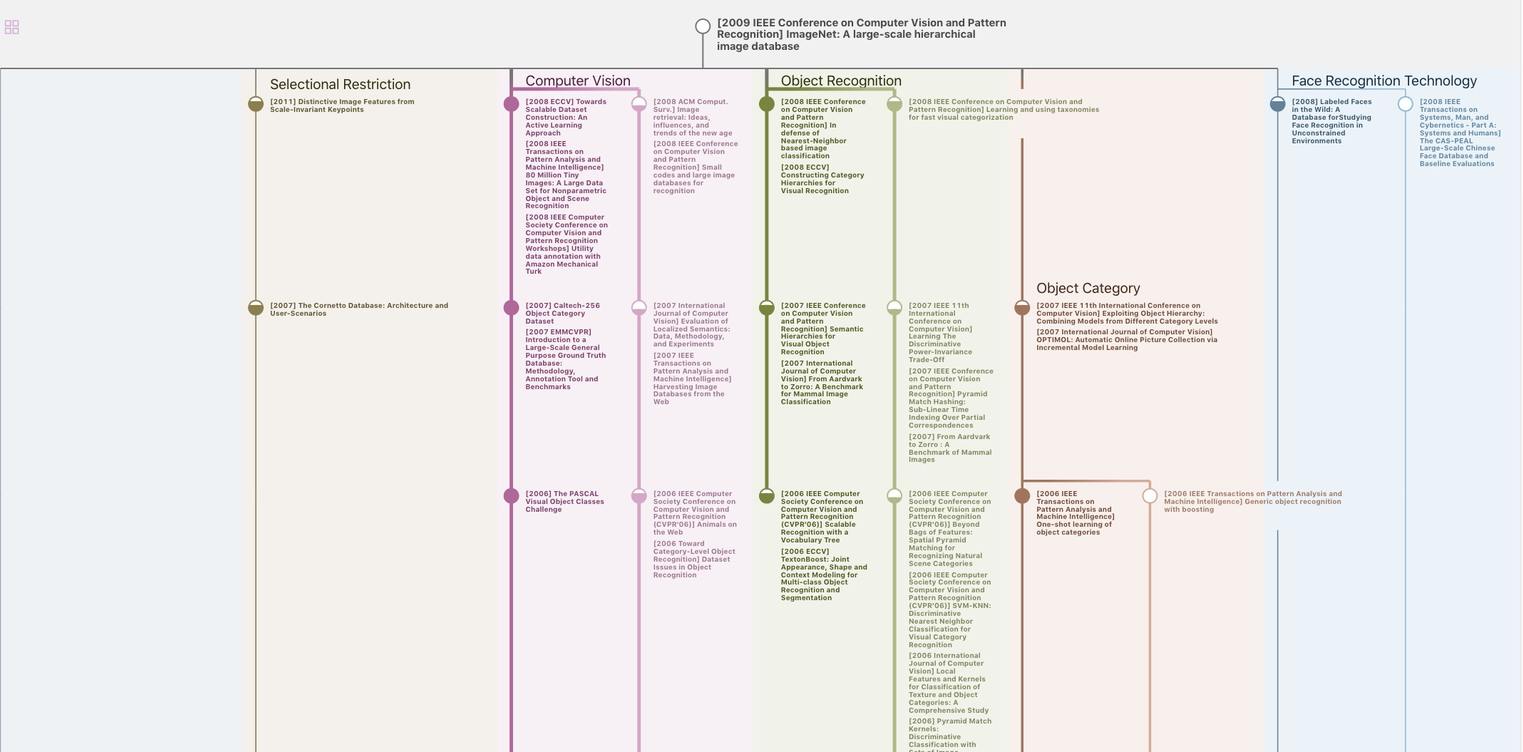
生成溯源树,研究论文发展脉络
Chat Paper
正在生成论文摘要