Histopathological Gastric Cancer Detection Using Transfer Learning
2023 11th International Conference on Bioinformatics and Computational Biology (ICBCB)(2023)
摘要
Gastric cancer is a leading cause of cancer-related deaths worldwide, underscoring the need for early detection to improve patient survival rates. Histopathological image analysis (HIA) is the gold standard for this purpose but it is time-consuming and laborious, leading to interest in computer-aided diagnosis to assist pathologists. Deep learning has shown promise in various HIA tasks, but the limited amount of training data in medical imaging has posed a significant obstacle. In this paper, we propose transfer learning-based CNNs for binary classification of gastric cancer patches, overcoming this limitation. We validate our approach on the publicly available GasHisSDB dataset, which includes three sub-databases with patch sizes of 80 x 80 pixels, 120 x 120 pixels, and 160 x 160 pixels. Our experimental results show that the DenseNet121 model achieved the highest accuracy of 98.68 % and AUC of 98.58% on the 160-pixels sub-database. These results suggest that our proposed work can assist pathologists in the detection of gastric cancer through histopathological image analysis.
更多查看译文
关键词
Gastric cancer,deep learning,transfer learning,histopathology,convolutional neural network,classification
AI 理解论文
溯源树
样例
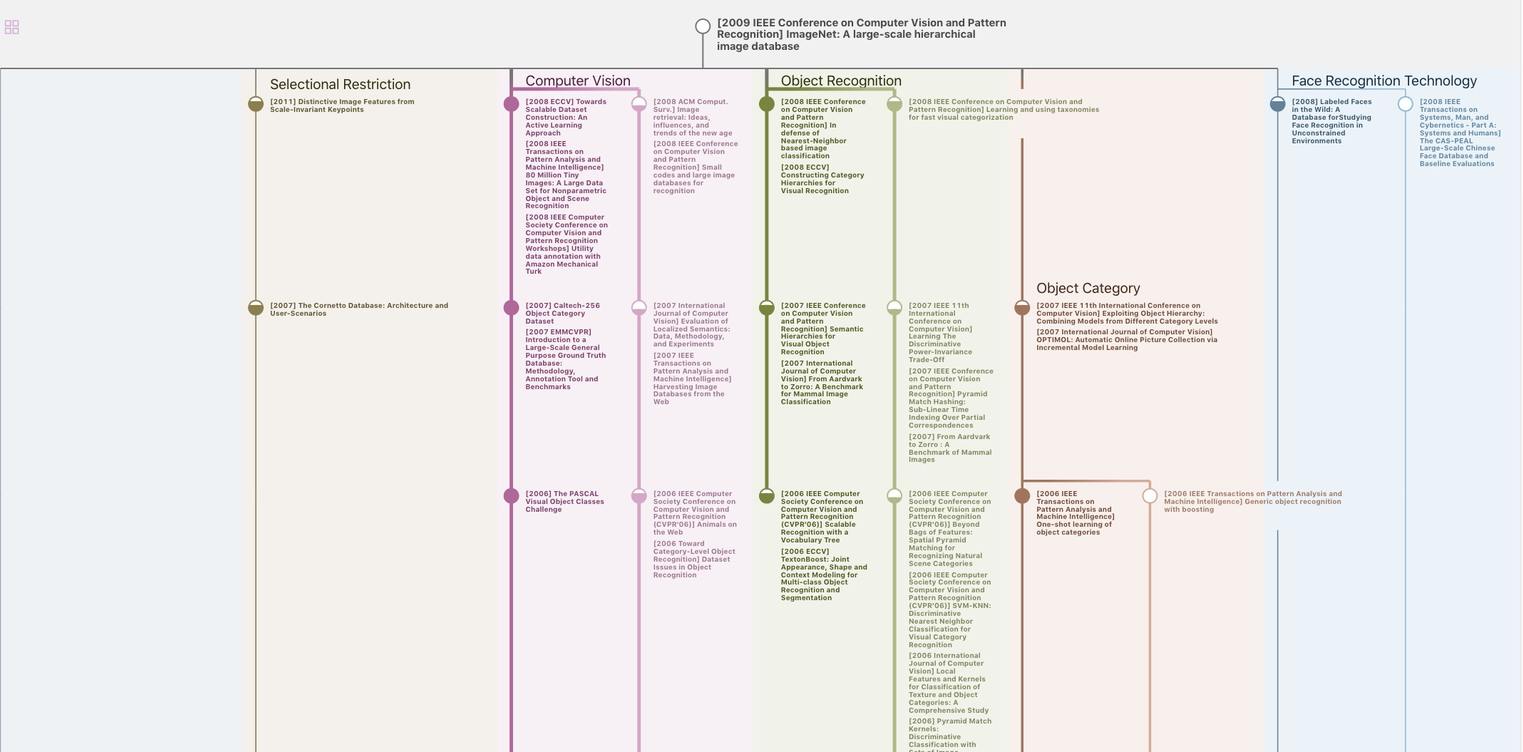
生成溯源树,研究论文发展脉络
Chat Paper
正在生成论文摘要