An Adaptive Divergence-Based Non-Negative Latent Factor Model
IEEE Transactions on Systems, Man, and Cybernetics: Systems(2023)
摘要
A High-dimensional and incomplete (HDI) matrix is regularly adopted to portray the inherent non-negativity of interactions among numerous nodes, which is involved in countless industrial applications driven by big data. An inherently non-negative latent factor (LF) model can take out the intrinsical features from such data conveniently and effectually due to its unimpeded training process. However, it constructs the learning objective relying on a standard Euclidean distance, thereby seriously restricting its representative ability to HDI data generated by different domains. To address this issue, this work proposes an adaptive divergence-based non-negative LF (ADNLF) model following: 1) constructing a generalized objective function based on
$\alpha - \beta $
-divergence to inflate its ability to represent various HDI data; 2) connecting the optimization variables with output LFs by a smooth and single LF-dependent bridging function to satisfy the non-negativity constraints constantly; and 3) facilitating adaptive divergence in the learning objective through particle swarm optimization for high scalability. Empirical studies on eight HDI matrices validate that an ADNLF model evidently outstrips state-of-the-art models in terms of estimation accuracy as well as computational efficiency for missing data of an HDI dataset.
更多查看译文
关键词
Adaptive divergence, big data, generalized divergence, high-dimensional and incomplete (HDI) data, intelligent system, latent factor (LF) model, non-negative LF (NLF) analysis
AI 理解论文
溯源树
样例
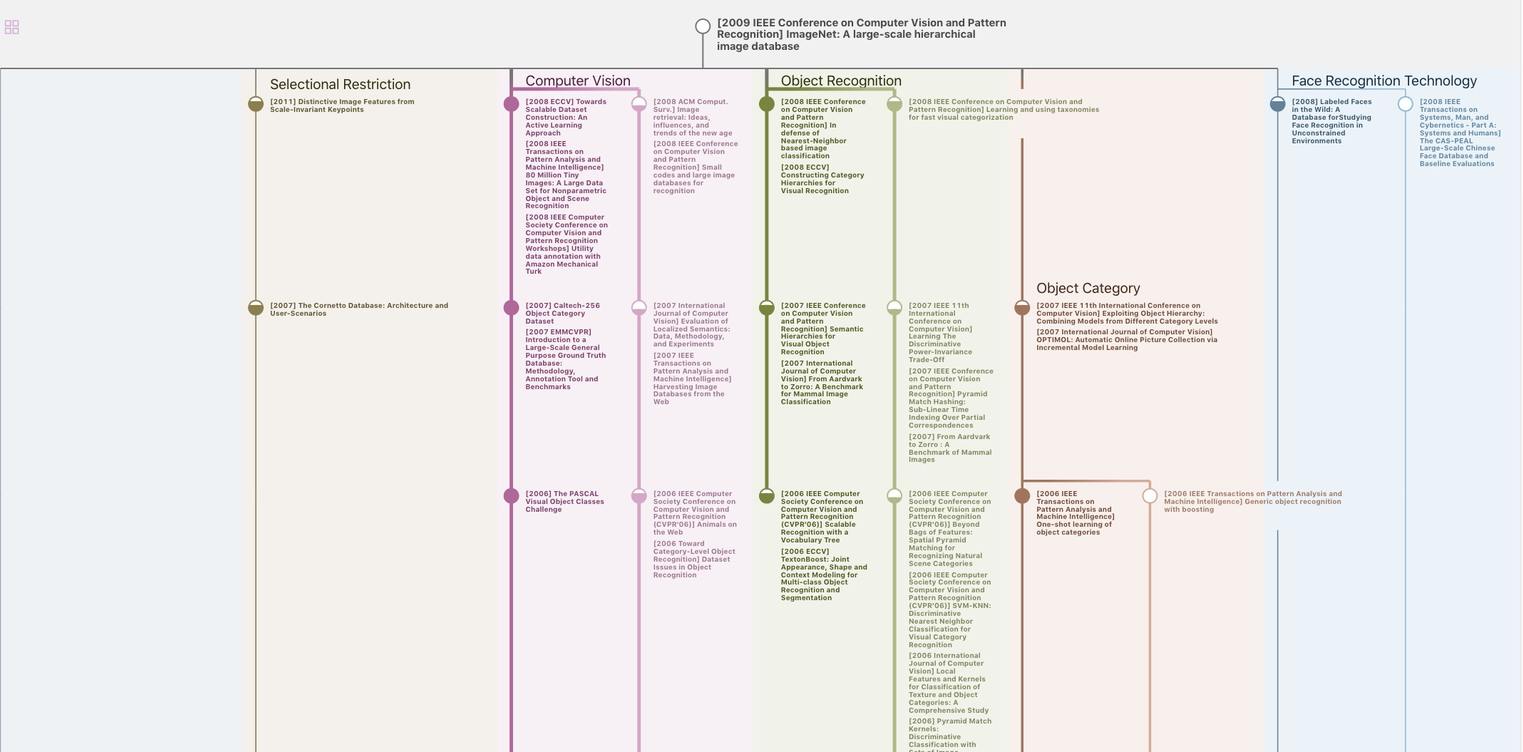
生成溯源树,研究论文发展脉络
Chat Paper
正在生成论文摘要