Are Large Language Model-based Evaluators the Solution to Scaling Up Multilingual Evaluation?
Conference of the European Chapter of the Association for Computational Linguistics(2023)
摘要
Large Language Models (LLMs) excel in various Natural Language Processing
(NLP) tasks, yet their evaluation, particularly in languages beyond the top
20, remains inadequate due to existing benchmarks and metrics limitations.
Employing LLMs as evaluators to rank or score other models' outputs emerges as
a viable solution, addressing the constraints tied to human annotators and
established benchmarks. In this study, we explore the potential of LLM-based
evaluators, specifically GPT-4 in enhancing multilingual evaluation by
calibrating them against 20K human judgments across three text-generation
tasks, five metrics, and eight languages. Our analysis reveals a bias in
GPT4-based evaluators towards higher scores, underscoring the necessity of
calibration with native speaker judgments, especially in low-resource and
non-Latin script languages, to ensure accurate evaluation of LLM performance
across diverse languages.
更多查看译文
关键词
multilingual evaluation,evaluators,language,model-based
AI 理解论文
溯源树
样例
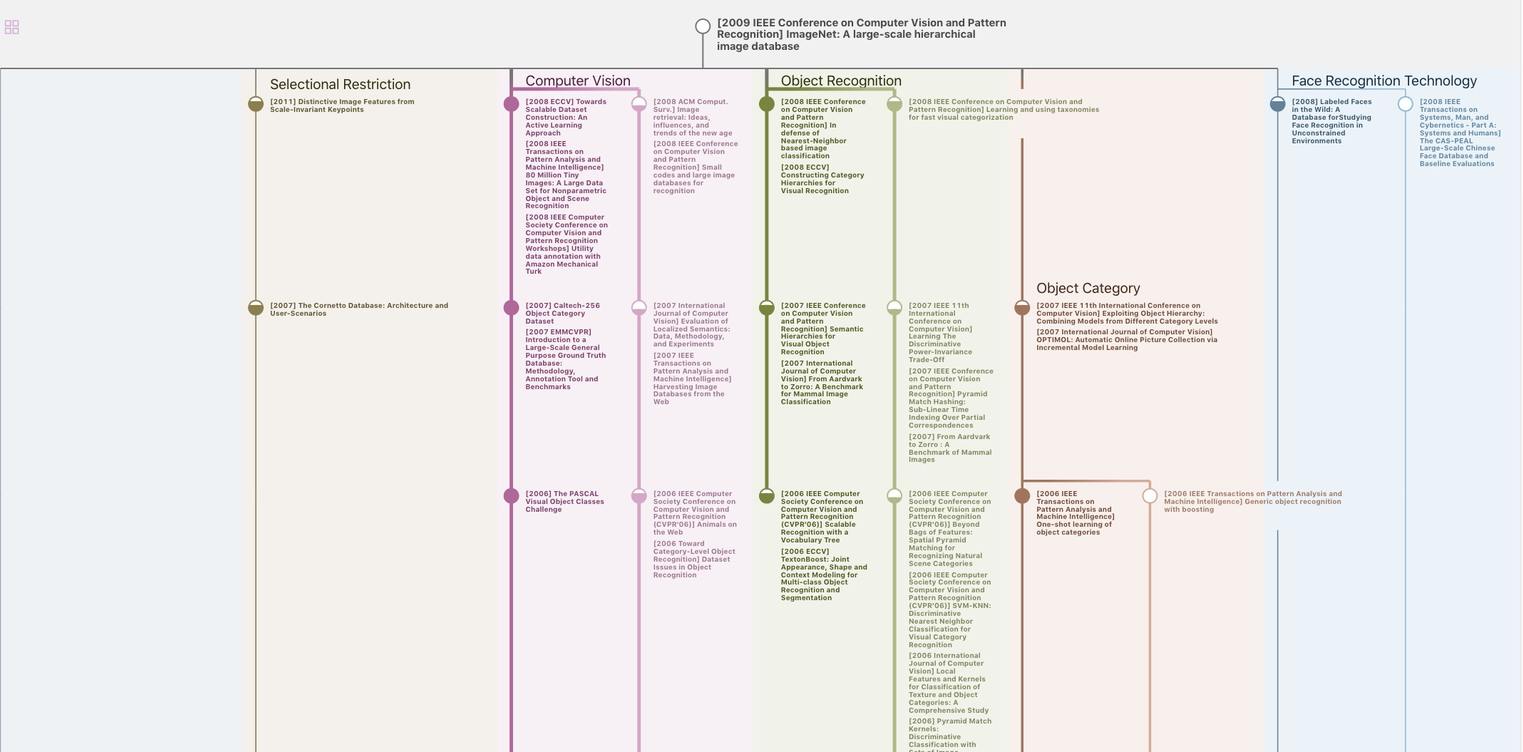
生成溯源树,研究论文发展脉络
Chat Paper
正在生成论文摘要