Movement tracking and action classification for human behaviour under threat in virtual reality
Gait & Posture(2023)
摘要
Understanding and characterising human movements is complex due to the diversity of human actions and their inherent inter, intra, and secular variability. Traditional marker-based, and more recently, some marker-less motion capture (MoCap) systems have demonstrated to be reliable tools for movement analysis. However, in complex experimental set ups involving virtual reality (VR) and free movements (as in [1]), accuracy and reliability tend to decrease due to occlusion, sensor blind spots, marker detachment, and other artifacts. Furthermore, when actions are less distinct, e.g., fast walk and slow run, current classification methods tend to fail when actions overlap, which is expected as even researchers struggle to manually label such actions. Can current marker-less MoCap systems, pose estimation (PE) algorithms, and advanced action classification (AC) methods: (1) accurately track participant movements in VR; (2) cluster participant actions. The experiment consisted of avoiding threats (Fig. 1A) whilst collecting fruit in VR environments (n=29 participants, 5x10m area), see [1]. The Unity® software [2], based on the Unity Experiment Framework [3], was used to create the VR experiment, which was streamed through an HTC vive pro (HTC Corporation) VR headset. Movements were recorded using 5 ELP cameras (1280×720 @120 Hz) synchronised with the Open Broadcaster Software® (OBS) [4]. Openpose [5] was employed for PE (Fig. 1B). Euclidean distances, and angular positions, velocities, and accelerations were derived from cartesian positions. Finally, Uniform Manifold Approximation and Projection (UMAP) was used to embed high-dimensional features into a low-dimensional space, and Hierarchical Density Based Spatial Clustering of Applications (HDBSCAN) was used for classification (see Fig. 1E), similar to B-SOiD [6]. Participants were virtually killed by the threat in 223 episodes, for which the participants’ last poses were estimated. After applying UMAP and HDBSCAN, 5 pose clusters were found (see Fig. 1C-D), which depict: (a) stand up, picking fruit with slow escape; (b) stand up, arms extended and slow escape; (c) long retreat at fast speed; (d) short retreat at medium speed; (e) crouching and picking fruit; (x) 4% unlabelled. Fig. 1. (A) VR-threat, (B) Participant estimated 3D-pose, (C) Pose clusters, (D) Cluster examples, (E) Methodology.Download : Download high-res image (176KB)Download : Download full-size image Marker-less MoCap and PE methods were mostly successful for participants’ last poses. However, in some cases, and during exploration, tracking was lost due to occlusion and sensor blind spots. The results from the AC methods are an indication of the potential use of unsupervised methods to find participant actions under threat in VR. Nevertheless, such clustering is rather general, and had some AC errors, which could not be quantified as further work is needed to understand and define where the threshold of overlapping actions occurs. The results are exciting and promising; however, further investigation is needed to validate the findings, and to improve the AC methods.
更多查看译文
关键词
action classification,human behaviour,virtual reality,movement
AI 理解论文
溯源树
样例
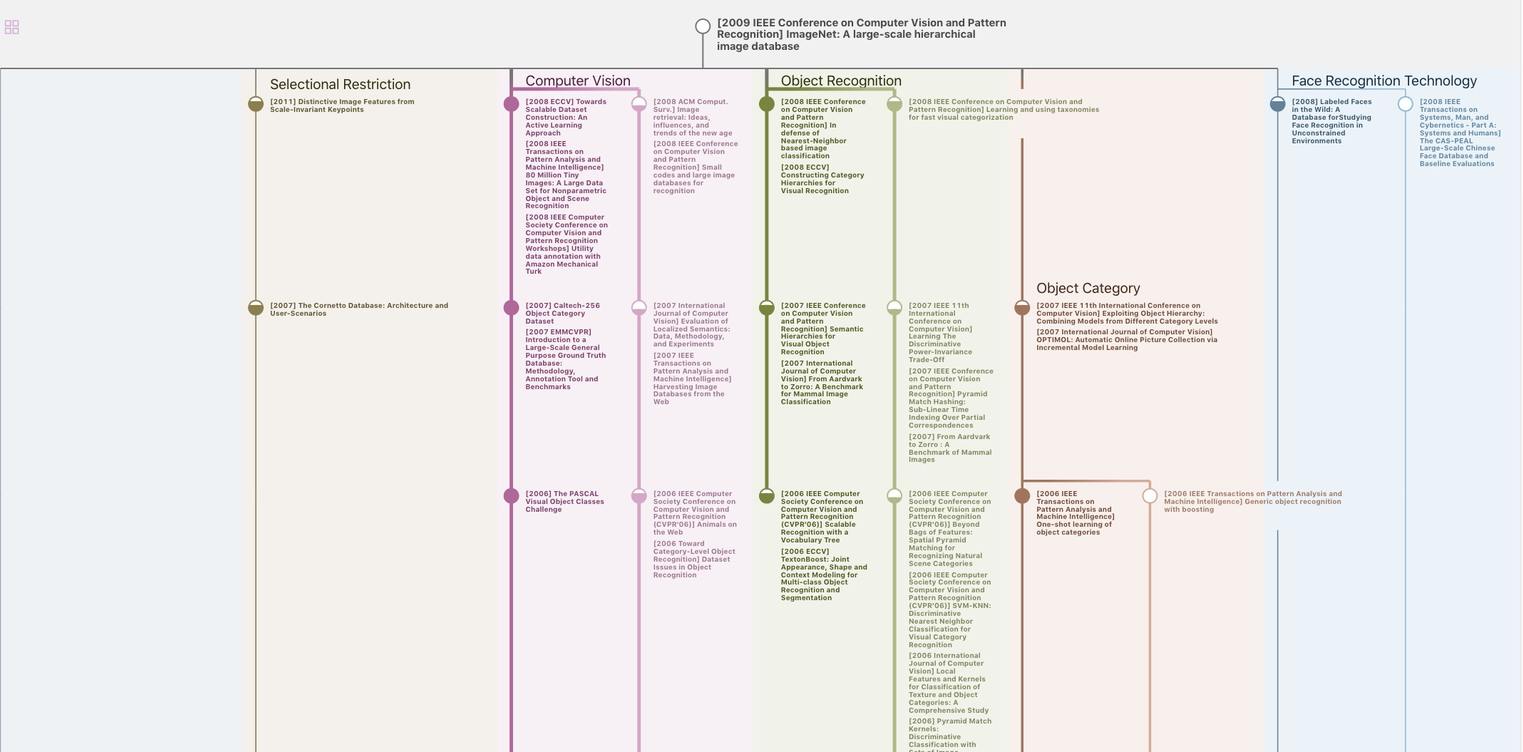
生成溯源树,研究论文发展脉络
Chat Paper
正在生成论文摘要