Morphing one dataset into another with maximum likelihood estimation
PHYSICAL REVIEW D(2023)
摘要
Many components of data analysis in high-energy physics and beyond require morphing one dataset into another. This is commonly solved via reweighting, but there are advantages of preserving weights and shifting the data points instead. Normalizing flows are machine learning models that have shown impressive precision on a variety of particle physics tasks. Naively, normalizing flows cannot be used for morphing because they require knowledge of the probability density of the starting dataset; in most cases in particle physics, we can generate more examples, but we do not know densities explicitly. We propose a protocol called flows for flows for training normalizing flows to morph one dataset into another even if the underlying probability density of neither dataset is known explicitly. This enables a morphing strategy trained with maximum likelihood estimation, a setup that has been shown to be highly effective in related tasks. We study variations on this protocol to explore how far the data points are moved to statistically match the two datasets. Furthermore, we show how to condition the learned flows on particular features in order to create a morphing function for every value of the conditioning feature. For illustration, we demonstrate flows for flows on toy examples as well as a collider physics example involving dijet events.
更多查看译文
AI 理解论文
溯源树
样例
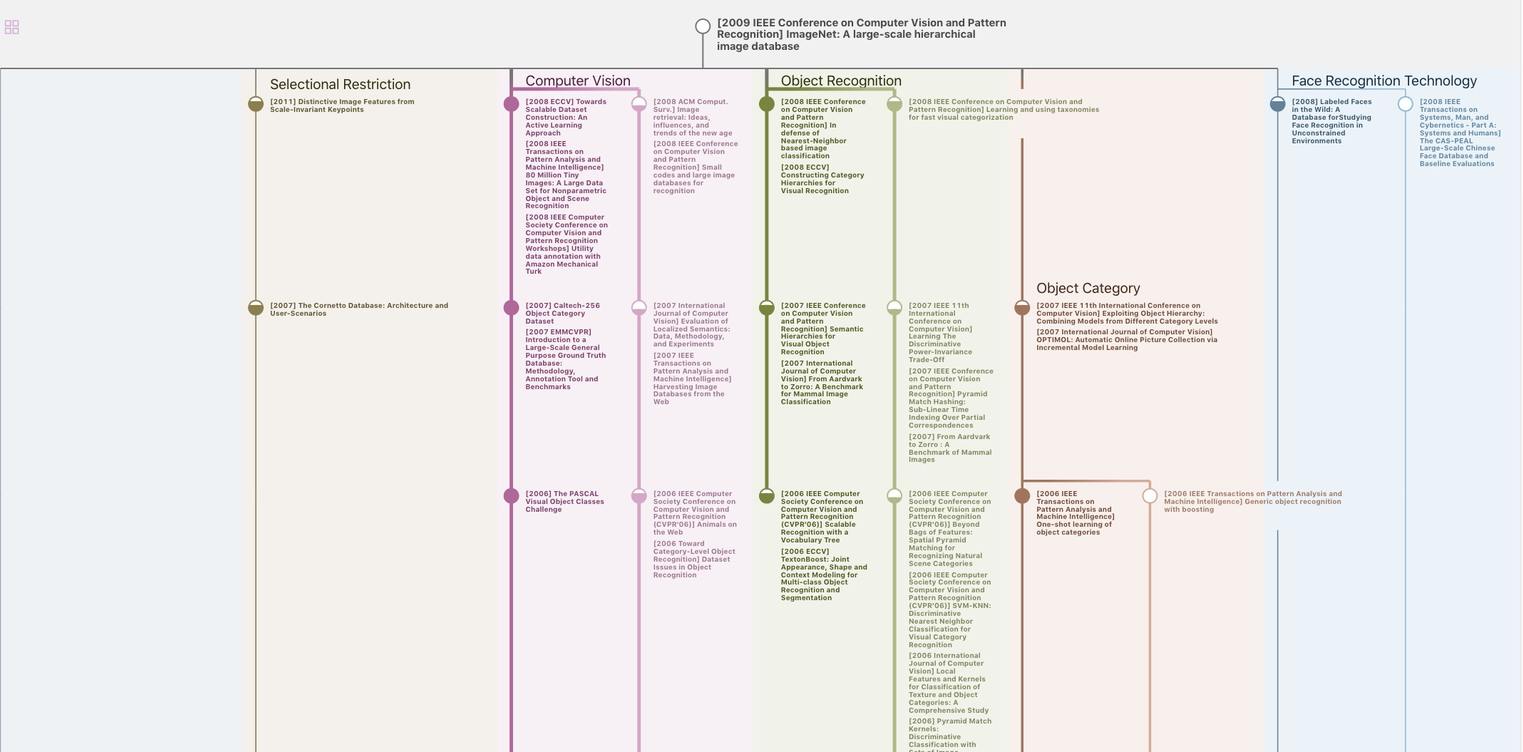
生成溯源树,研究论文发展脉络
Chat Paper
正在生成论文摘要