AI pitfalls and what not to do: mitigating bias in AI
BRITISH JOURNAL OF RADIOLOGY(2023)
摘要
Various forms of artificial intelligence (AI) applications are being deployed and used in many healthcare systems. As the use of these applications increases, we are learning the failures of these models and how they can perpetuate bias. With these new lessons, we need to prioritize bias evaluation and mitigation for radiology applications; all the while not ignoring the impact of changes in the larger enterprise AI deployment which may have downstream impact on perfor-mance of AI models. In this paper, we provide an updated review of known pitfalls causing AI bias and discuss strategies for mitigating these biases within the context of AI deployment in the larger healthcare enterprise. We describe these pitfalls by framing them in the larger AI lifecycle from problem definition, data set selection and curation, model training and deployment emphasizing that bias exists across a spectrum and is a sequela of a combination of both human and machine factors.
更多查看译文
关键词
ai pitfalls,bias
AI 理解论文
溯源树
样例
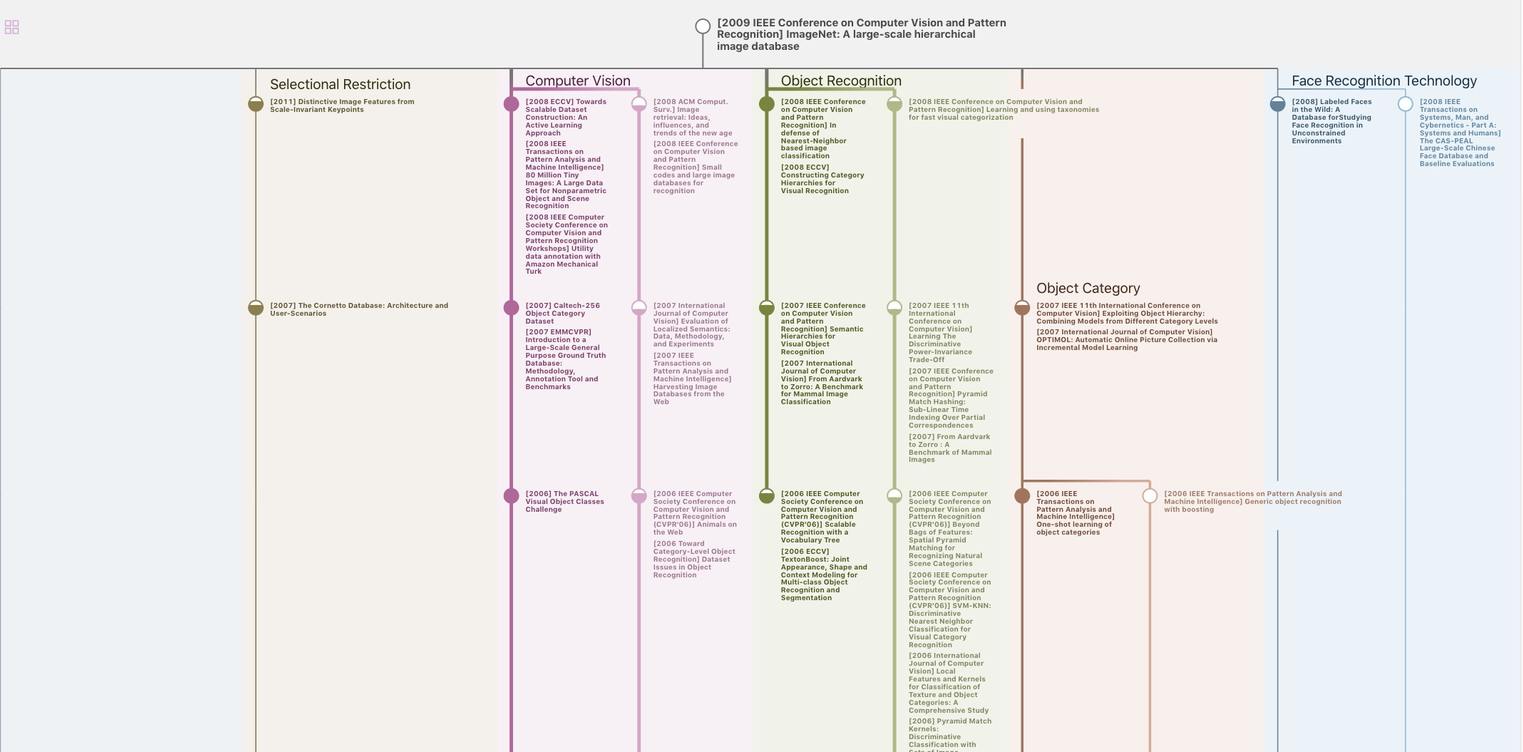
生成溯源树,研究论文发展脉络
Chat Paper
正在生成论文摘要