Deterministic Framework based Structured Learning for Quadrotors
2023 27th International Conference on Methods and Models in Automation and Robotics (MMAR)(2023)
摘要
The design of a continuous learning controller for quadrotors often entails some specific implementations that require significant system knowledge and are prone to experience catastrophic forgetting. To address these challenges, a deterministic approach is trained using a quadrotor on a relatively small amount of automatically generated data. The Twin Delayed Deep Deterministic Policy Gradient (TD3) algorithm is utilized to develop the policy for learning the maneuvers of a quadrotor and controlling it alongside the low-level controller. The algorithm outlined demonstrates proficiency in handling large state spaces and actions that are continuous. It integrates clipped double Q-learning, target policy smoothing, and delayed policy updates, all of which contribute to its effectiveness in training. The proposed control technique’s efficacy is evaluated through numerical simulations conducted on a quadrotor in both standard and windy conditions. The results identified that learning with TD3 reduced the overestimation bias, improved the convergence accuracy, and achieved efficient maneuver with less tracking error by using the dense reward structure.
更多查看译文
关键词
Quadrotor,Reinforcement Learning,Twin Delayed deep deterministic policy gradient,Critic networks,Replay buffer
AI 理解论文
溯源树
样例
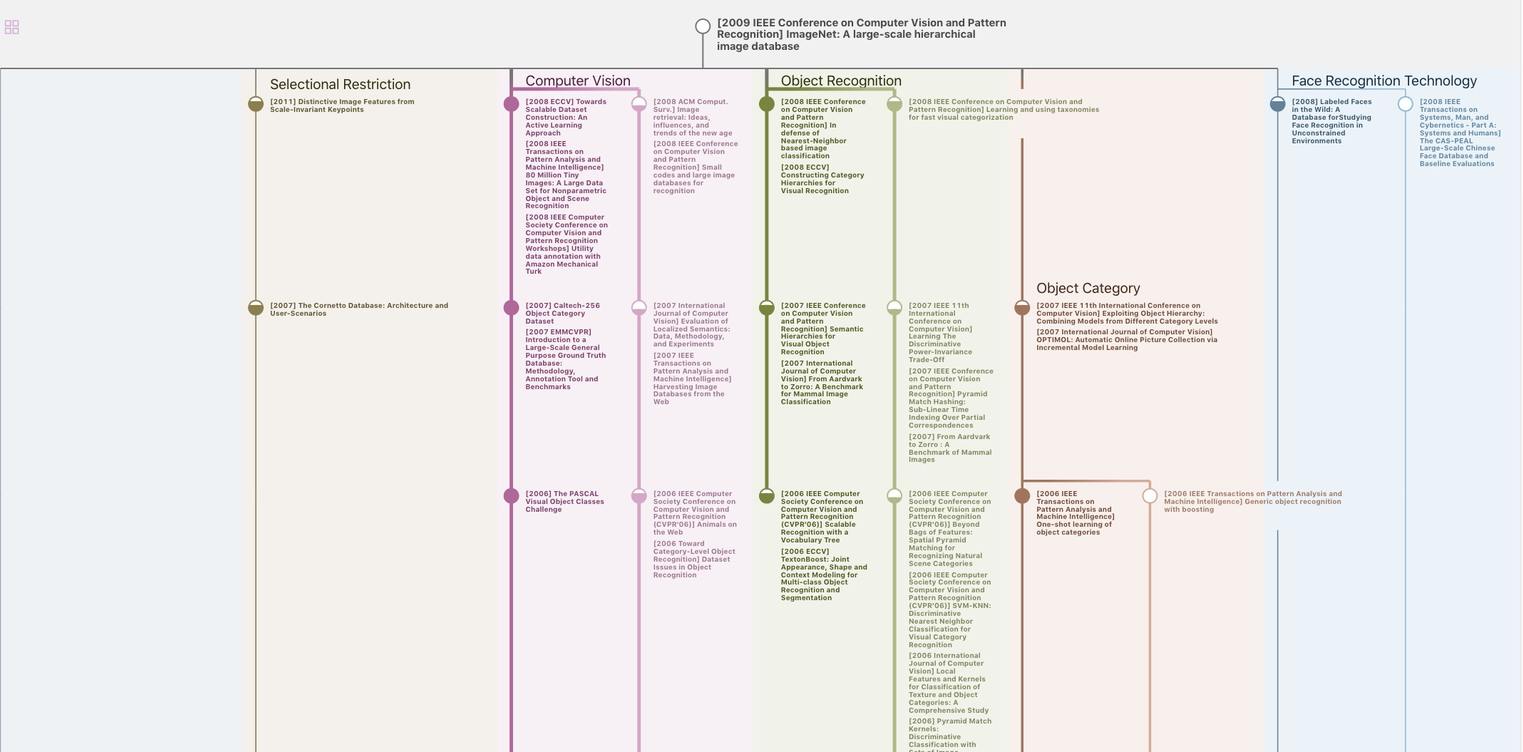
生成溯源树,研究论文发展脉络
Chat Paper
正在生成论文摘要