Local-global contrast for learning voice-face representations
2023 IEEE INTERNATIONAL CONFERENCE ON IMAGE PROCESSING, ICIP(2023)
摘要
Leveraging deep learning to explore the associations between voices and faces has attracted extensive research interest. Usually, this research is formalized by cross-modal verification, matching, and retrieval tasks. Most works rely on a single local or global optimization objective for learning, ignoring that testing scenarios may prefer different optimization objectives. For example, local objectives are more helpful for verification and matching tasks, while global objectives contribute more to retrieval. In this study, we proposed a learning framework based on local and global objectives to improve the generalizability of the learned representations. Firstly, we explored two ways of applying supervised contrastive loss (SCL) to learn voice-face representations. Secondly, we designed a contrastive-form global optimization objective, which shows better performance and training efficiency. Experiments on the VoxCeleb dataset demonstrate the effectiveness of our framework.
更多查看译文
关键词
voice-face association,supervised contrastive learning,cross-modal retrieval,multi-modal learning
AI 理解论文
溯源树
样例
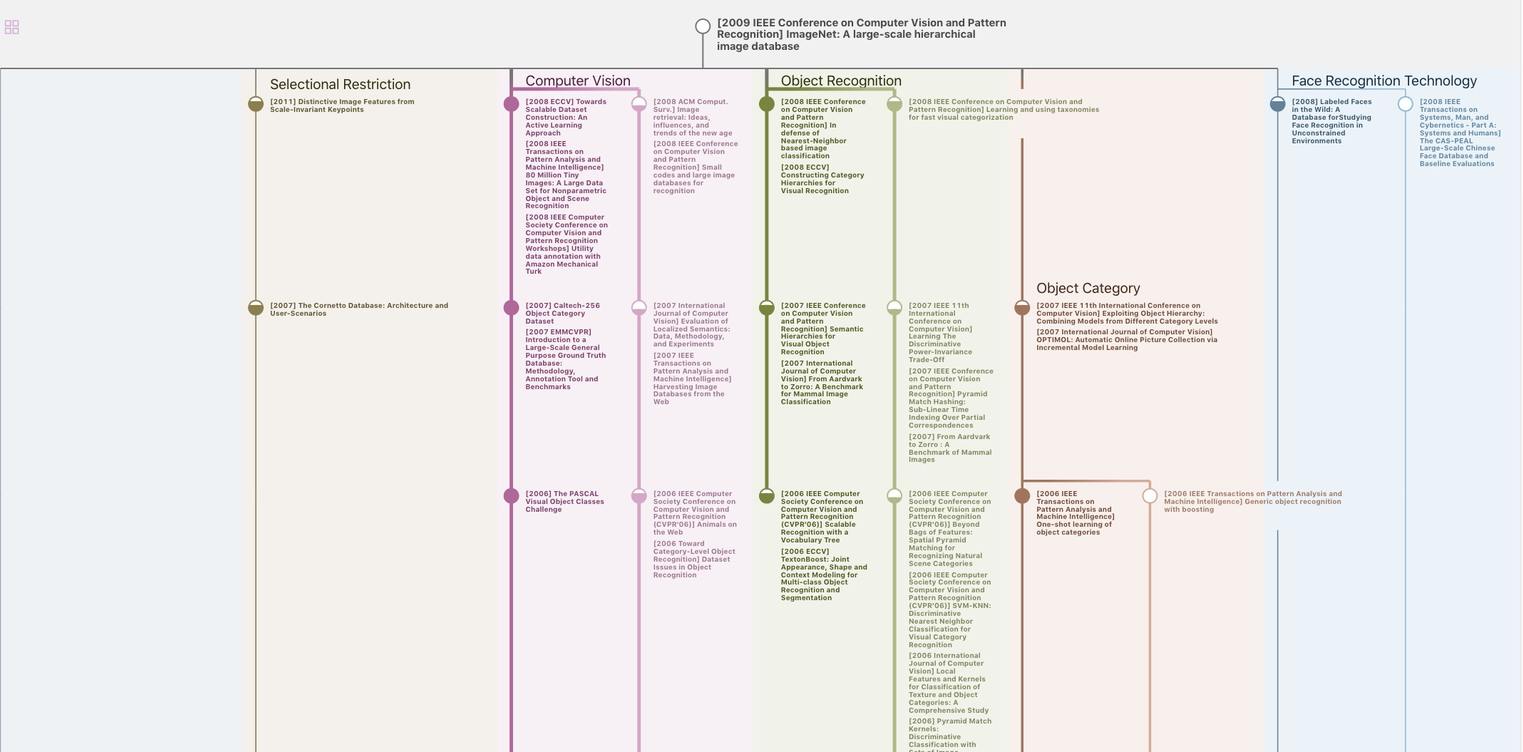
生成溯源树,研究论文发展脉络
Chat Paper
正在生成论文摘要