Edge Video Analytics With Adaptive Information Gathering: A Deep Reinforcement Learning Approach
IEEE TRANSACTIONS ON WIRELESS COMMUNICATIONS(2023)
摘要
With growing popularity of enormous public safety and transportation infrastructure cameras, there are increasing demands for automatic mobile video analytics. The emerging multi-access edge computing (MEC) technology has been recently applied to improve the accuracy-latency tradeoff of mobile video analytics. In this paper, we study an MEC-enabled multi-device video analytics system and formulate the problem as a Markov decision process (MDP) to meet two practical challenges: i) the absence of ground truth in real-time and ii) the content-varying degradation-accuracy relation. In particular, we aim to design an online joint frame degradation and bandwidth allocation algorithm with the time-varying function and limited feedback from each device. Thanks to the MDP formulation and n-step return technique, the long-term goal offers adaptive information gathering and thus improves the average accuracy and latency. For sample efficiency, we decompose the MDP problem into discrete degradation adaptation subproblems and continuous bandwidth allocation subproblems. Based on the decomposition, we propose a deep reinforcement learning (DRL) based framework, referred to as DBAG, to solve the decomposed subproblems. DBAG integrates model-based optimization and model-free DRL to solve the MDP problem with a discrete-continuous hybrid action space. Under various network setups and public datasets, DBAG greatly improves the accuracy-latency tradeoff.
更多查看译文
关键词
Multi-access edge computing,resource allocation,deep reinforcement learning,video analytics,markove decision process
AI 理解论文
溯源树
样例
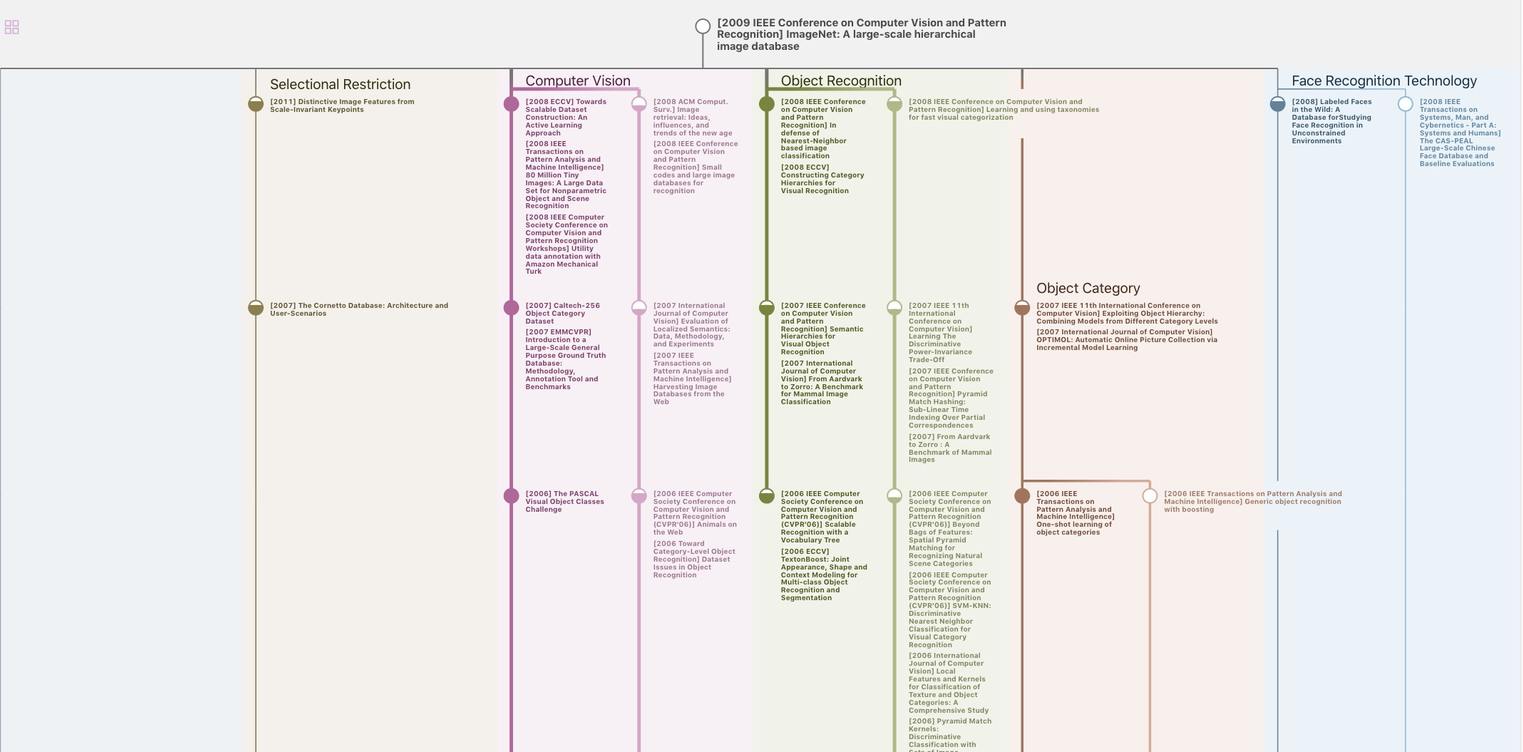
生成溯源树,研究论文发展脉络
Chat Paper
正在生成论文摘要