Learning Objective-Specific Active Learning Strategies with Attentive Neural Processes
MACHINE LEARNING AND KNOWLEDGE DISCOVERY IN DATABASES: RESEARCH TRACK, ECML PKDD 2023, PT I(2023)
摘要
Pool-based active learning (AL) is a promising technology for increasing data-efficiency of machine learning models. However, surveys show that performance of recent AL methods is very sensitive to the choice of dataset and training setting, making them unsuitable for general application. In order to tackle this problem, the field Learning Active Learning (LAL) suggests to learn the active learning strategy itself, allowing it to adapt to the given setting. In this work, we propose a novel LAL method for classification that exploits symmetry and independence properties of the active learning problem with an Attentive Conditional Neural Process model. Our approach is based on learning from a myopic oracle, which gives our model the ability to adapt to non-standard objectives, such as those that do not equally weight the error on all data points. We experimentally verify that our Neural Process model outperforms a variety of baselines in these settings. Finally, our experiments show that our model exhibits a tendency towards improved stability to changing datasets. However, performance is sensitive to choice of classifier and more work is necessary to reduce the performance the gap with the myopic oracle and to improve scalability. We present our work as a proof-of-concept for LAL on nonstandard objectives and hope our analysis and modelling considerations inspire future LAL work.
更多查看译文
关键词
Active Learning,Deep Learning,Neural Process
AI 理解论文
溯源树
样例
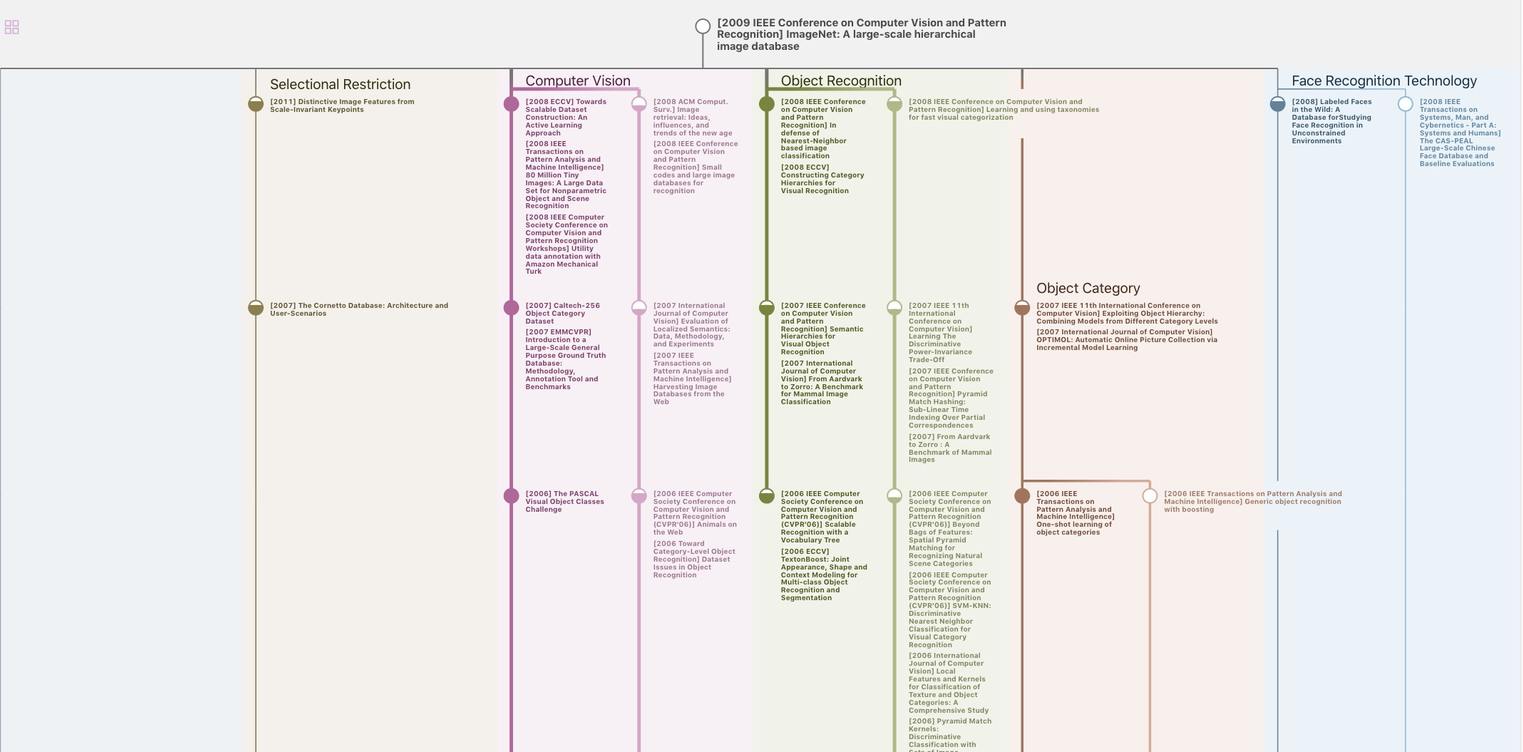
生成溯源树,研究论文发展脉络
Chat Paper
正在生成论文摘要