SIM-Sync: From Certifiably Optimal Synchronization Over the 3D Similarity Group to Scene Reconstruction With Learned Depth
IEEE ROBOTICS AND AUTOMATION LETTERS(2024)
摘要
We present SIM-Sync, a certifiably optimal algorithm that estimates camera trajectory and 3D scene structure directly from multiview image keypoints. The key enabler of SIM-Sync is a pretrained depth prediction network. Given a graph with nodes representing monocular images taken at unknown camera poses and edges containing pairwise image keypoint correspondences, SIM-Sync first uses a pretrained depth prediction network to lift the 2D keypoints into 3D scaled point clouds, where the scaling of the per-image point cloud is unknown due to the scale ambiguity in monocular depth prediction. SIM-Sync then seeks to synchronize jointly the unknown camera poses and scaling factors (i.e., over the 3D similarity group) by minimizing the sum of the Euclidean distances between edge-wise scaled point clouds. The SIM-Sync formulation, despite being nonconvex, allows for the design of an efficient, certifiably optimal solver that is almost identical to the SE-Sync algorithm. Particularly, after solving the translations in closed-form, the remaining optimization over the rotations and scales can be written as a quadratically constrained quadratic program, for which we apply Shor's semidefinite relaxation. We demonstrate the empirical tightness and practical usefulness of SIM-Sync in both simulated and real experiments, and investigate the impact of graph structure and sparsity.
更多查看译文
关键词
Global optimization,robot learning,robot vision systems,state estimation
AI 理解论文
溯源树
样例
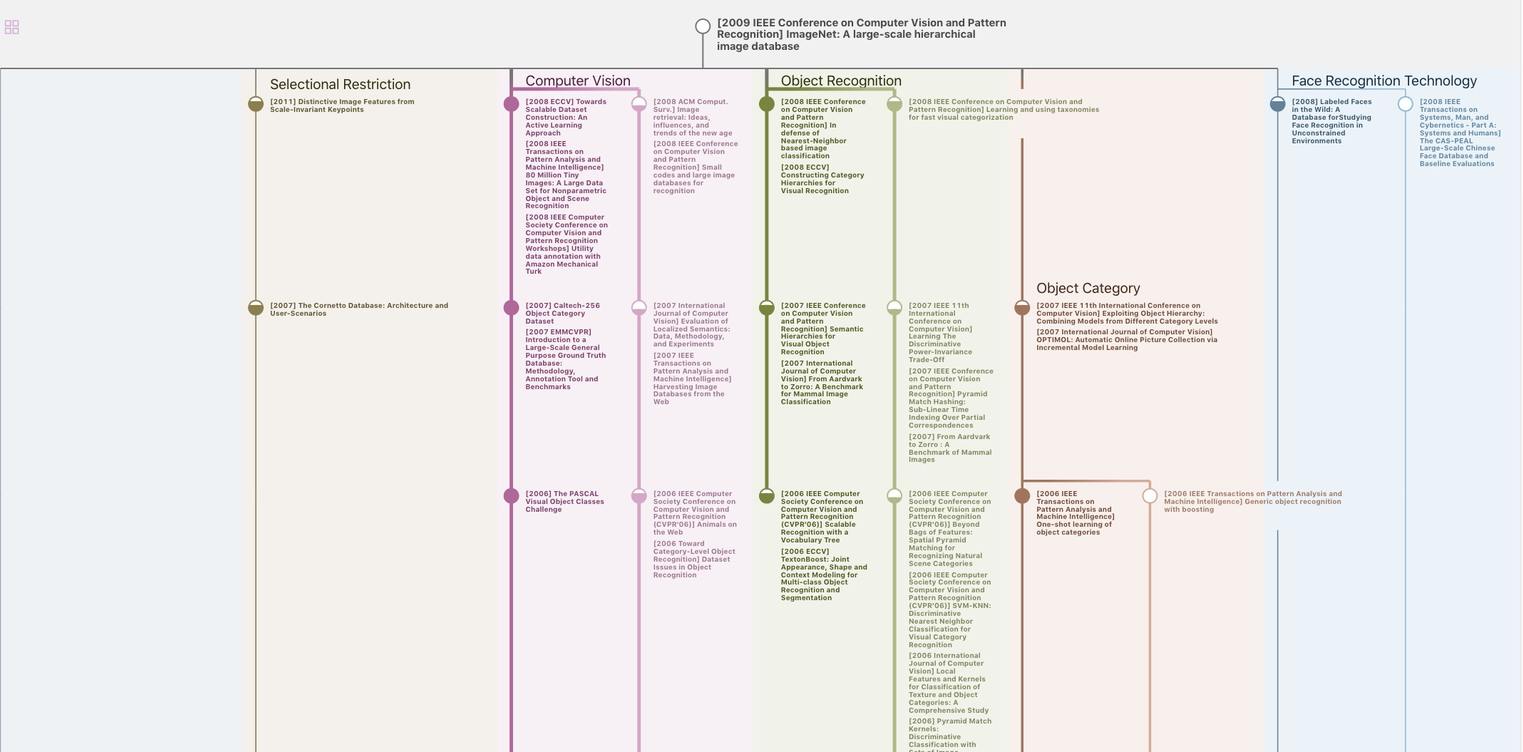
生成溯源树,研究论文发展脉络
Chat Paper
正在生成论文摘要