EFC-YOLO: An Efficient Surface-Defect-Detection Algorithm for Steel Strips.
Sensors (Basel, Switzerland)(2023)
摘要
The pursuit of higher recognition accuracy and speed with smaller model sizes has been a major research topic in the detection of surface defects in steel. In this paper, we propose an improved high-speed and high-precision Efficient Fusion Coordination network (EFC-YOLO) without increasing the model's size. Since modifications to enhance feature extraction in shallow networks tend to affect the speed of model inference, in order to simultaneously ensure the accuracy and speed of detection, we add the improved Fusion-Faster module to the backbone network of YOLOv7. Partial Convolution (PConv) serves as the basic operator of the module, which strengthens the feature-extraction ability of shallow networks while maintaining speed. Additionally, we incorporate the Shortcut Coordinate Attention (SCA) mechanism to better capture the location information dependency, considering both lightweight design and accuracy. The de-weighted Bi-directional Feature Pyramid Network (BiFPN) structure used in the neck part of the network improves the original Path Aggregation Network (PANet)-like structure by adding step branches and reducing computations, achieving better feature fusion. In the experiments conducted on the NEU-DET dataset, the final model achieved an 85.9% mAP and decreased the GFLOPs by 60%, effectively balancing the model's size with the accuracy and speed of detection.
更多查看译文
关键词
surface defect detection, YOLOv7, deep learning, feature extraction
AI 理解论文
溯源树
样例
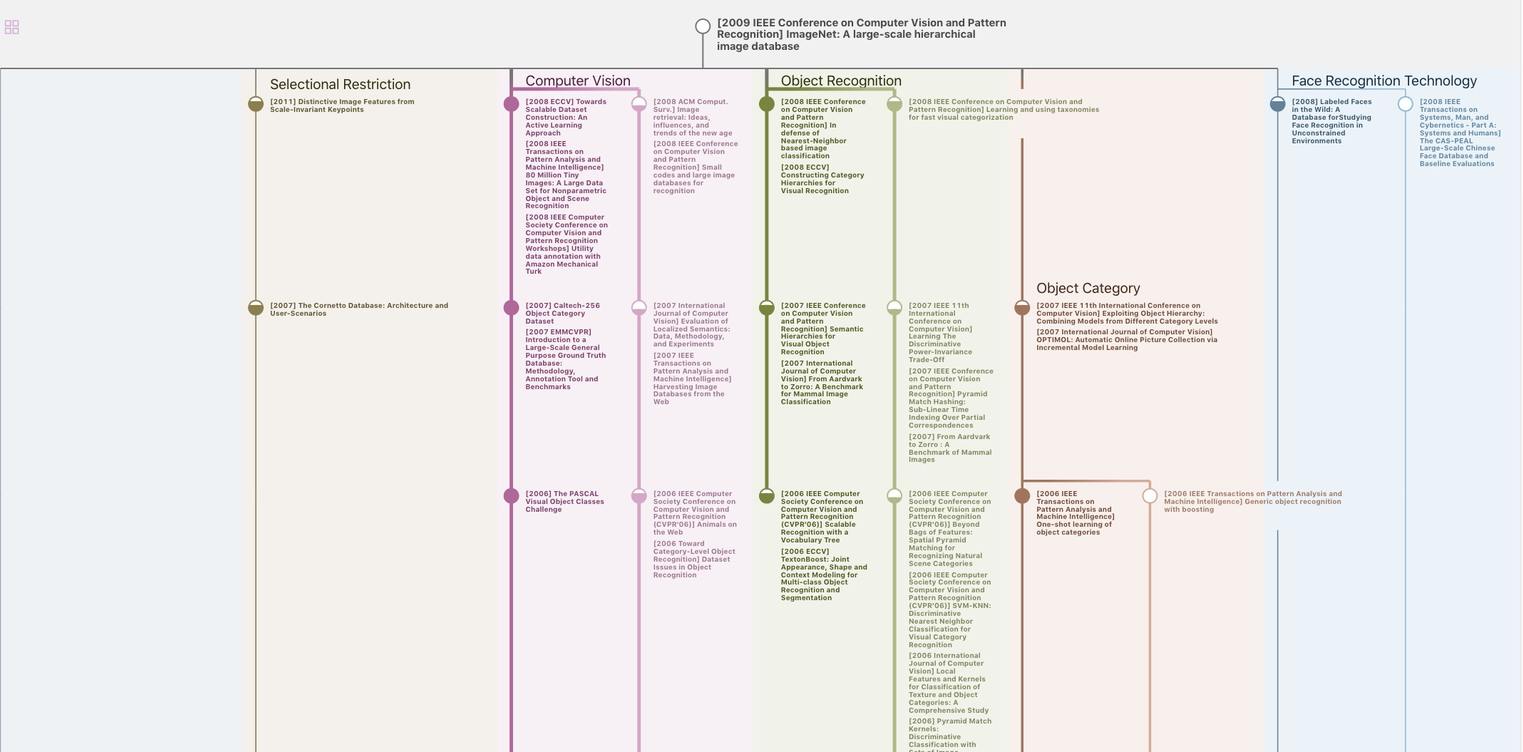
生成溯源树,研究论文发展脉络
Chat Paper
正在生成论文摘要