Qualitative Classification of Proximal Femoral Bone Using Geometric Features and Texture Analysis in Collected MRI Images for Bone Density Evaluation.
Sensors (Basel, Switzerland)(2023)
摘要
The aim of this study was to use geometric features and texture analysis to discriminate between healthy and unhealthy femurs and to identify the most influential features. We scanned proximal femoral bone (PFB) of 284 Iranian cases (21 to 83 years old) using different dual-energy X-ray absorptiometry (DEXA) scanners and magnetic resonance imaging (MRI) machines. Subjects were labeled as "healthy" (T-score > -0.9) and "unhealthy" based on the results of DEXA scans. Based on the geometry and texture of the PFB in MRI, 204 features were retrieved. We used support vector machine (SVM) with different kernels, decision tree, and logistic regression algorithms as classifiers and the Genetic algorithm (GA) to select the best set of features and to maximize accuracy. There were 185 participants classified as healthy and 99 as unhealthy. The SVM with radial basis function kernels had the best performance (89.08%) and the most influential features were geometrical ones. Even though our findings show the high performance of this model, further investigation with more subjects is suggested. To our knowledge, this is the first study that investigates qualitative classification of PFBs based on MRI with reference to DEXA scans using machine learning methods and the GA.
更多查看译文
关键词
osteoporosis, magnetic resonance imaging, dual energy X-ray absorptiometry, machine learning
AI 理解论文
溯源树
样例
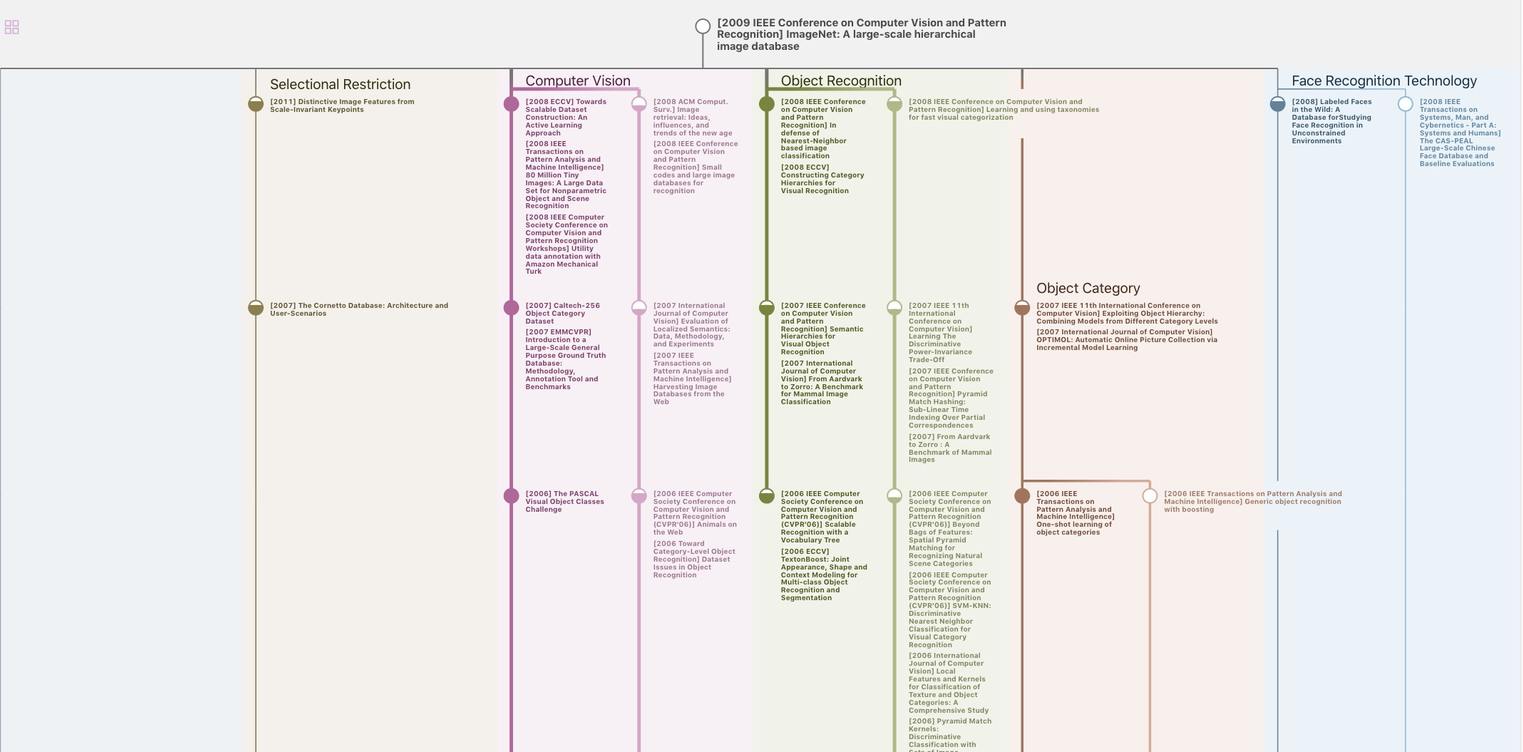
生成溯源树,研究论文发展脉络
Chat Paper
正在生成论文摘要