Parkinson's Disease Detection Using Filter Feature Selection and a Genetic Algorithm with Ensemble Learning.
Diagnostics (Basel, Switzerland)(2023)
摘要
Parkinson's disease (PD) is a neurodegenerative disorder marked by motor and non-motor symptoms that have a severe impact on the quality of life of the affected individuals. This study explores the effect of filter feature selection, followed by ensemble learning methods and genetic selection, on the detection of PD patients from attributes extracted from voice clips from both PD patients and healthy patients. Two distinct datasets were employed in this study. Filter feature selection was carried out by eliminating quasi-constant features. Several classification models were then tested on the filtered data. Decision tree, random forest, and XGBoost classifiers produced remarkable results, especially on Dataset 1, where 100% accuracy was achieved by decision tree and random forest. Ensemble learning methods (voting, stacking, and bagging) were then applied to the best-performing models to see whether the results could be enhanced further. Additionally, genetic selection was applied to the filtered data and evaluated using several classification models for their accuracy and precision. It was found that in most cases, the predictions for PD patients showed more precision than those for healthy individuals. The overall performance was also better on Dataset 1 than on Dataset 2, which had a greater number of features.
更多查看译文
关键词
parkinsons disease,filter feature selection,disease detection
AI 理解论文
溯源树
样例
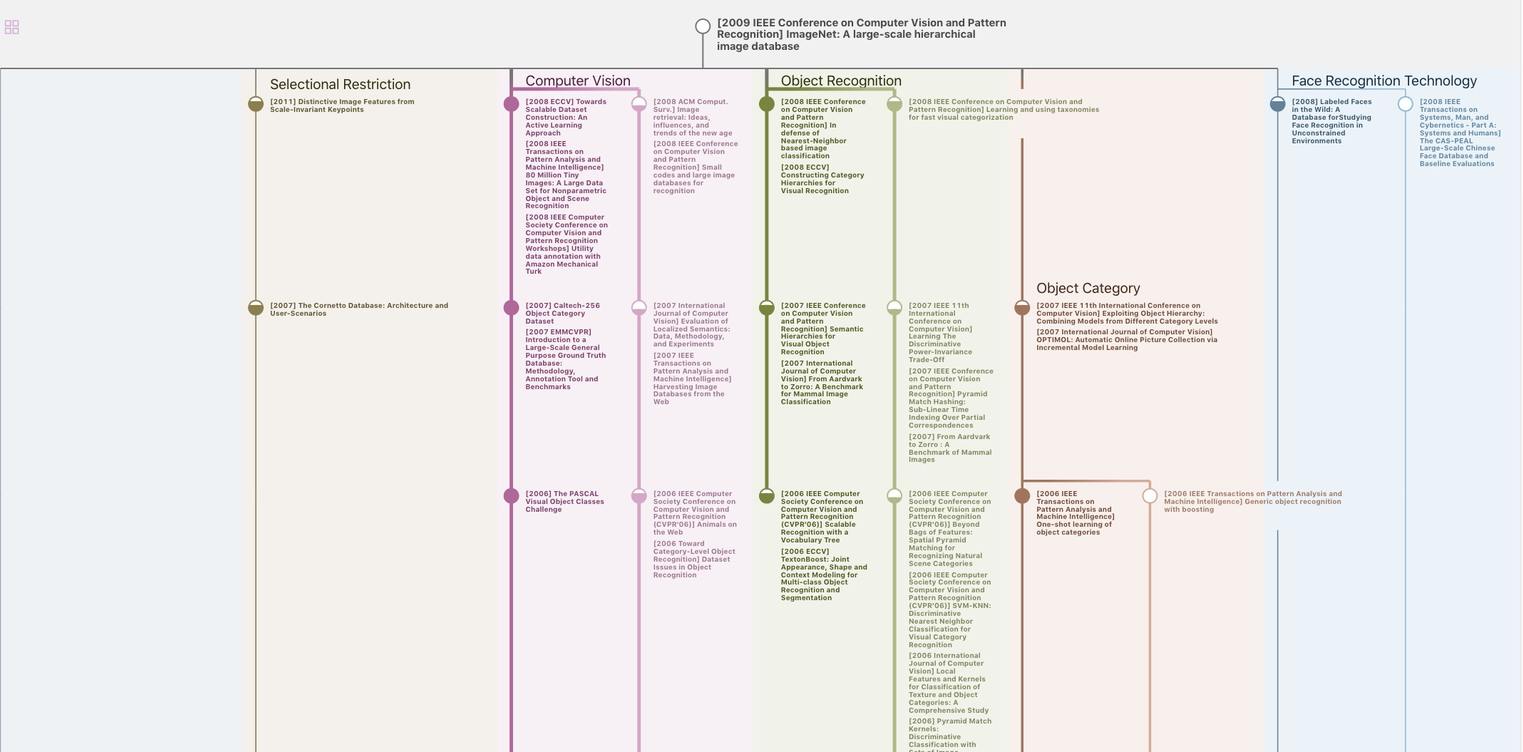
生成溯源树,研究论文发展脉络
Chat Paper
正在生成论文摘要