LJIR: Learning Joint-Action Intrinsic Reward in cooperative multi-agent reinforcement learning.
Neural networks : the official journal of the International Neural Network Society(2023)
摘要
Effective exploration is the key to achieving high returns for reinforcement learning. Agents must explore jointly in multi-agent systems to find the optimal joint policy. Due to the exploration problem and the shared reward, the policy-based multi-agent reinforcement learning algorithms face policy overfitting, which may lead to the joint policy falling into a local optimum. This paper introduces a novel general framework called Learning Joint-Action Intrinsic Reward (LJIR) for improving multi-agent reinforcement learners' joint exploration ability and performance. LJIR observes agents' state and joint actions to learn to construct an intrinsic reward online that can guide effective joint exploration. With the novel combination of Transformer and random network distillation, LJIR selects the novel states to give more intrinsic rewards, which help agents find the best joint actions. LJIR can dynamically adjust the weight of exploration and exploitation during training and keep the policy invariance finally. To ensure LJIR seamlessly adopts existing MARL algorithms, we also provide a flexible combination method for intrinsic and external rewards. Empirical results on the SMAC benchmark show that the proposed method achieves state-of-the-art performance in challenging tasks.
更多查看译文
关键词
Reinforcement learning,Multi-agent system,Intrinsic reward,Curiosity-driven exploration,Transformer
AI 理解论文
溯源树
样例
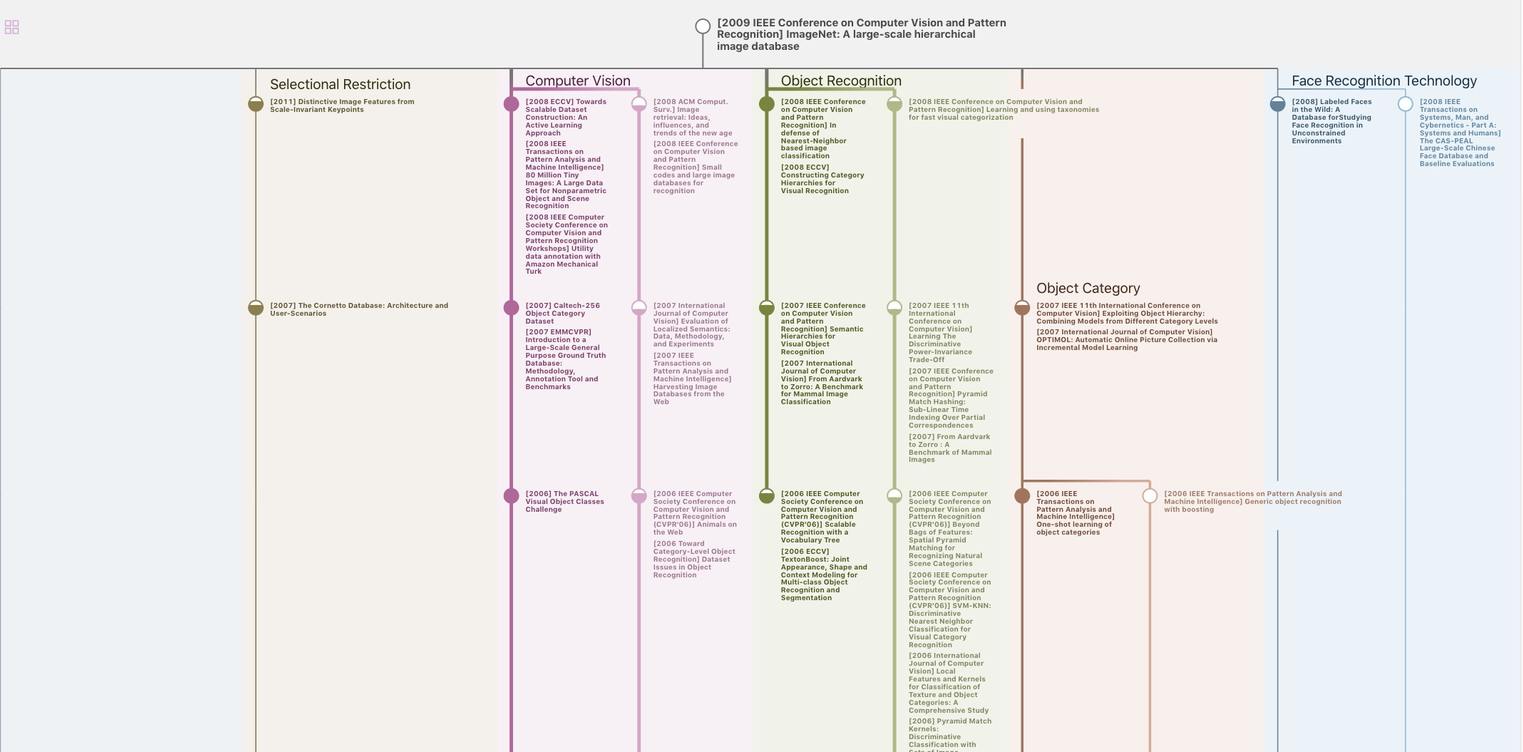
生成溯源树,研究论文发展脉络
Chat Paper
正在生成论文摘要