Feasibility study of super-resolution deep learning-based reconstruction using k-space data in brain diffusion-weighted images
Neuroradiology(2023)
摘要
Purpose The purpose of this study is to evaluate the influence of super-resolution deep learning-based reconstruction (SR-DLR), which utilizes k-space data, on the quality of images and the quantitation of the apparent diffusion coefficient (ADC) for diffusion-weighted images (DWI) in brain magnetic resonance imaging (MRI). Methods A retrospective analysis was performed on 34 patients who had undergone DWI using a 3 T MRI system with SR-DLR reconstruction based on k-space data in August 2022. DWI was reconstructed with SR-DLR (Matrix = 684 × 684) and without SR-DLR (Matrix = 228 × 228). Measurements were made of the signal-to-noise ratio (SNR), contrast-to-noise ratio (CNR) in white matter (WM) and grey matter (GM), and the full width at half maximum (FWHM) of the septum pellucidum. Two radiologists assessed image noise, contrast, artifacts, blur, and the overall quality of three image types using a four-point scale. Quantitative and qualitative scores between images with and without SR-DLR were compared using the Wilcoxon signed-rank test. Results Images with SR-DLR showed significantly higher SNRs and CNRs than those without SR-DLR ( p < 0.001). No statistically significant variances were found in the apparent diffusion coefficients (ADCs) in WM and GM between images with and without SR-DLR (ADC in WM, p = 0.945; ADC in GM, p = 0.235). Moreover, the FWHM without SR-DLR was notably lower compared to that with SR-DLR ( p < 0.001). Conclusion SR-DLR has the potential to augment the quality of DWI in DL MRI scans without significantly impacting ADC quantitation.
更多查看译文
关键词
Retrospective studies,Magnetic resonance imaging,Echo-planar imaging,Diffusion,Deep learning
AI 理解论文
溯源树
样例
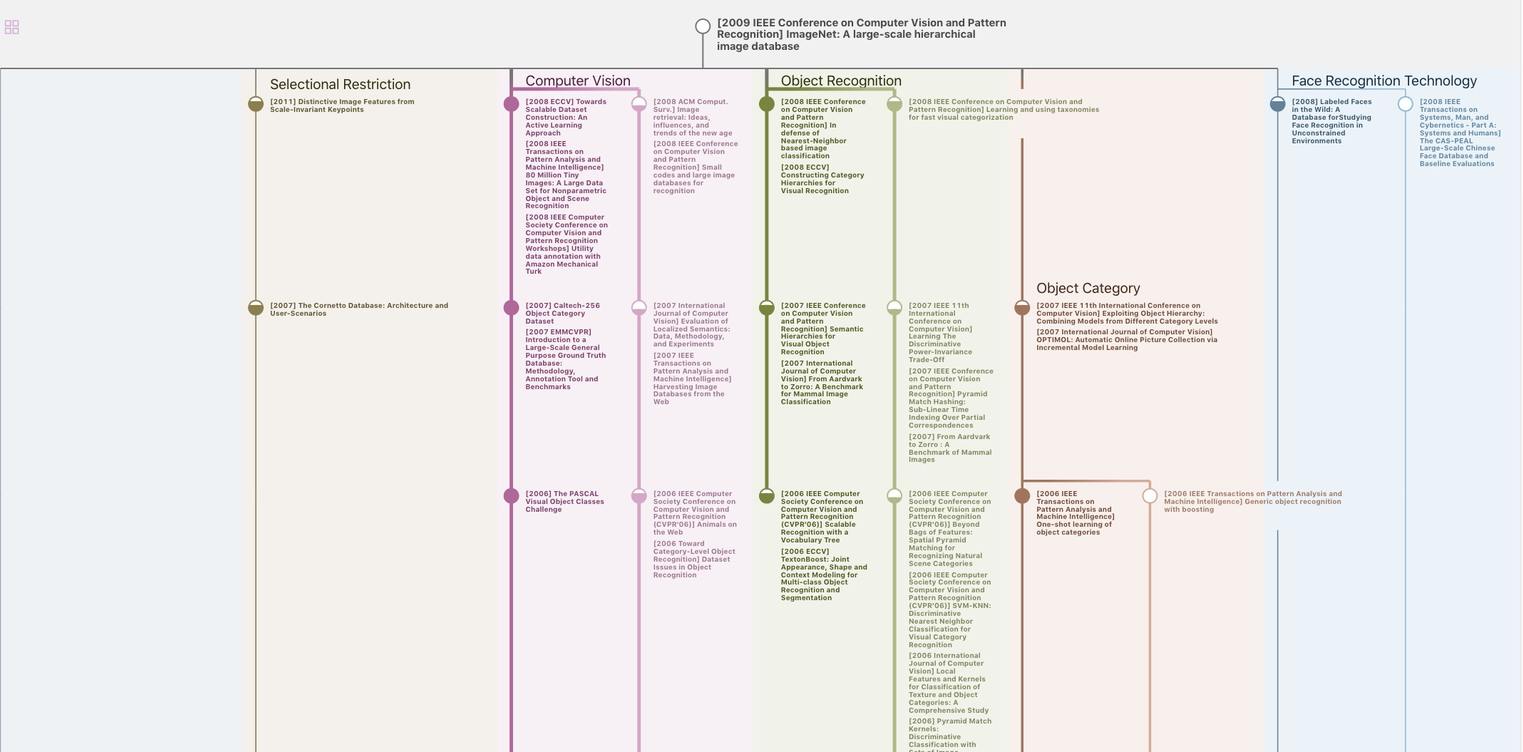
生成溯源树,研究论文发展脉络
Chat Paper
正在生成论文摘要