$L_{2,1}$-Norm Regularized Quaternion Matrix Completion Using Sparse Representation and Quaternion QR Decomposition
arXiv (Cornell University)(2023)
摘要
Color image completion is a challenging problem in computer vision, but recent research has shown that quaternion representations of color images perform well in many areas. These representations consider the entire color image and effectively utilize coupling information between the three color channels. Consequently, low-rank quaternion matrix completion (LRQMC) algorithms have gained significant attention. We propose a method based on quaternion Qatar Riyal decomposition (QQR) and quaternion $L_{2,1}$-norm called QLNM-QQR. This new approach reduces computational complexity by avoiding the need to calculate the QSVD of large quaternion matrices. We also present two improvements to the QLNM-QQR method: an enhanced version called IRQLNM-QQR that uses iteratively reweighted quaternion $L_{2,1}$-norm minimization and a method called QLNM-QQR-SR that integrates sparse regularization. Our experiments on natural color images and color medical images show that IRQLNM-QQR outperforms QLNM-QQR and that the proposed QLNM-QQR-SR method is superior to several state-of-the-art methods.
更多查看译文
关键词
quaternion qr decomposition,sparse
AI 理解论文
溯源树
样例
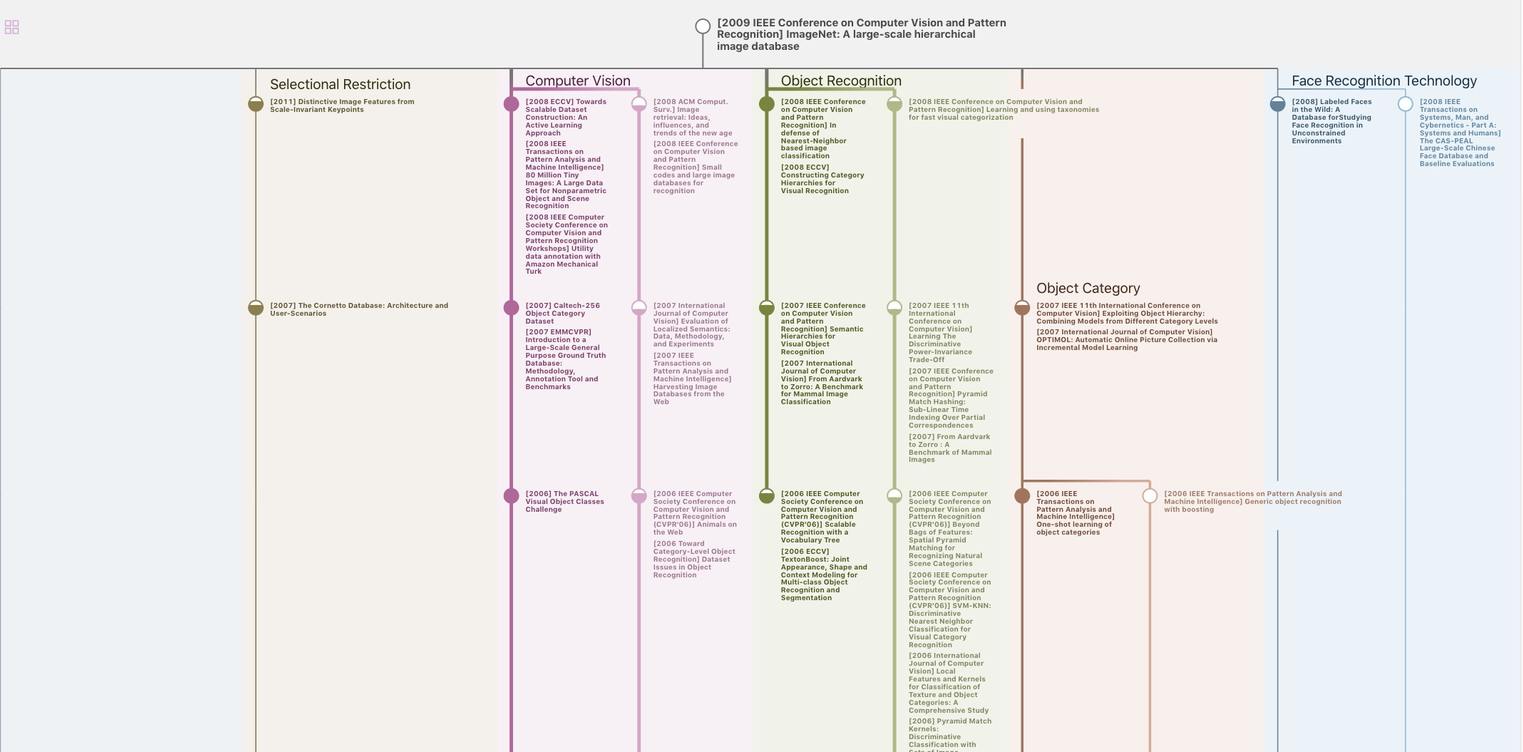
生成溯源树,研究论文发展脉络
Chat Paper
正在生成论文摘要