Efficient Baselines for Motion Prediction in Autonomous Driving
IEEE TRANSACTIONS ON INTELLIGENT TRANSPORTATION SYSTEMS(2023)
摘要
Motion Prediction (MP) of multiple surroundings agents is a crucial task in arbitrarily complex environments, from simple robots to Autonomous Driving Stacks (ADS). Current techniques tackle this problem using end-to-end pipelines, where the input data is usually a rendered top-view of the physical information and the past trajectories of the most relevant agents; leveraging this information is a must to obtain optimal performance. In that sense, a reliable ADS must produce reasonable predictions on time. However, despite many approaches use simple ConvNets and LSTMs to obtain the social latent features, State-of-the-Art (SOTA) models might be too complex for real-time applications when using both sources of information (map and past trajectories) as well as little interpretable, specifically considering the physical information. Moreover, the performance of such models highly depends on the number of available inputs for each particular traffic scenario, which are expensive to obtain, particularly, annotated High-Definition (HD) maps. In this work, we propose several efficient baselines for the well-known Argoverse 1 Motion Forecasting Benchmark. We aim to develop compact models using SOTA techniques for MP, including attention mechanisms and GNNs. Our lightweight models use standard social information and interpretable map information such as points from the driveable area and plausible centerlines by means of a novel physics-based heuristic step based on kinematic constraints, in opposition to black-box CNN-based or too-complex graphs methods for map encoding, to generate plausible multi-modal trajectories achieving up-to-pair accuracy with less operations and parameters than other SOTA methods. Our code is publicly available at https://github.com/Cram3r95/mapfe4mp.
更多查看译文
关键词
Trajectory,Roads,History,Task analysis,Predictive models,Autonomous vehicles,Pipelines,Autonomous driving,Argoverse,trajectory forecasting,motion prediction,multi-agent,deep learning
AI 理解论文
溯源树
样例
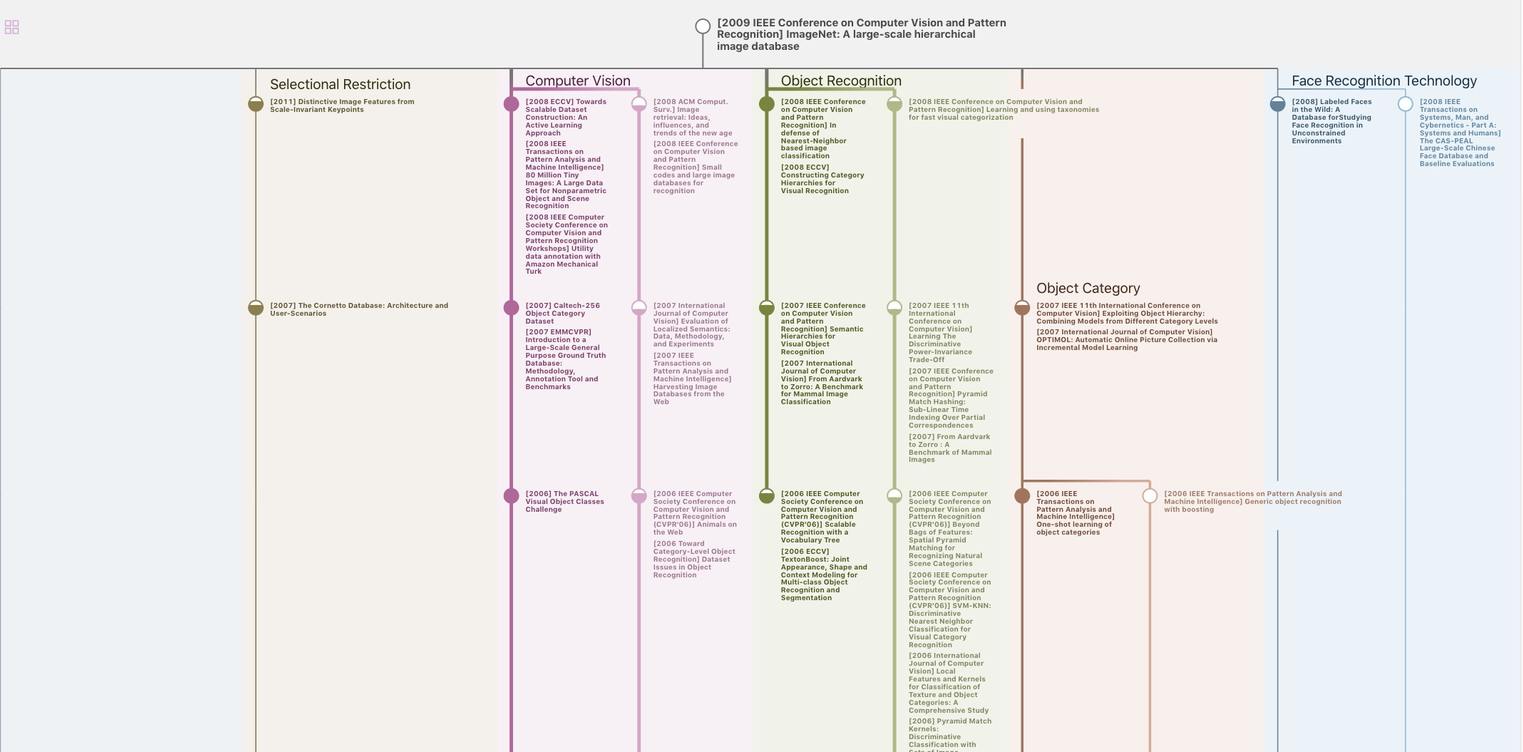
生成溯源树,研究论文发展脉络
Chat Paper
正在生成论文摘要