SyNIG: Synthetic Network Traffic Generation through Time Series Imaging.
IEEE Conference on Local Computer Networks(2023)
摘要
Immense growth of network usage and the associated proliferation of network, traffic, traffic classes, and diverse QoS requirements pose numerous challenges for network operators. Though data-driven approaches can provide better solutions for these challenges, limited data has been a barrier to developing those methods with high resiliency. In this work, we propose SyNIG (Synthetic Network Traffic Generation through Time Series Imaging), which utilizes Generative Adversarial Networks (GANs) for network traffic synthesis by converting time series data to a specific image format called GASF (Gramian Angular Summation Field). With GASF images we encode correlation between samples in 1D signals on a single 2D pixel map. Taking three types of network traffic; video streaming, accessing websites and IoT, we synthesize over 200,000 traces using over 40,000 original traces generalizing our method for different network traffic. We validate our method by demonstrating the fidelity of the synthetic data and applying them to several network related use cases showing improved performance.
更多查看译文
AI 理解论文
溯源树
样例
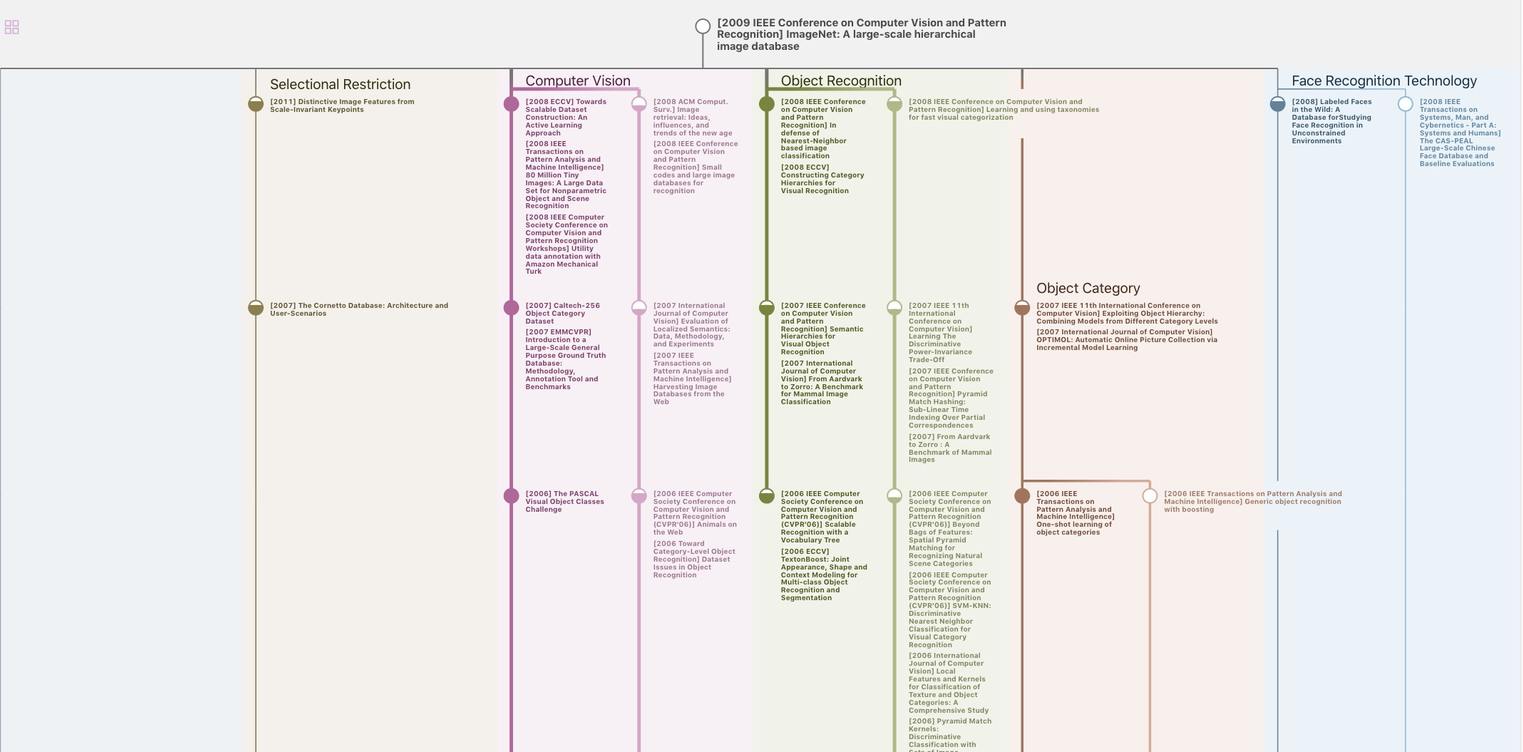
生成溯源树,研究论文发展脉络
Chat Paper
正在生成论文摘要