Data-Based Optimal Microgrid Management for Energy Trading With Integral Q -Learning Scheme
IEEE Internet of Things Journal(2023)
摘要
This article proposes an integral
${Q}$
-learning scheme to study the optimum management strategy of the battery for energy trading in the microgrid. The obtained optimal strategies are managed to minimize the cost of the microgrid, and simultaneously guarantee the better performance of the battery such that service life of battery is extended. First, the microgrid model is constructed, where renewable energy and profiled loads are considered. To satisfy the microgrid demand and the environmental energy consumption, an integral
${Q}$
-learning scheme will be developed for the input policy of the microgrid energy system such that the model dynamics are avoided. Moreover, the minimized cost and the property of the battery are considered in the performance function. Rather than the traditional
${Q}$
-learning iterative scheme, this article proposes a self-learning architecture for the integral-
${Q}$
learning scheme with two neural networks. The first network can learn the optimal
${Q}$
-function. Another network is used to learn the optimal action such that the
${Q}$
-function is minimized and load demand can be satisfied. The network weights are updated with the gradient method and the stability analysis is presented. Finally, the experiment data is used to verify the proposed integral
${Q}$
-learning scheme.
更多查看译文
关键词
Approximate dynamic programming (ADP),energy trading,neural network,Q-learning,smart grid
AI 理解论文
溯源树
样例
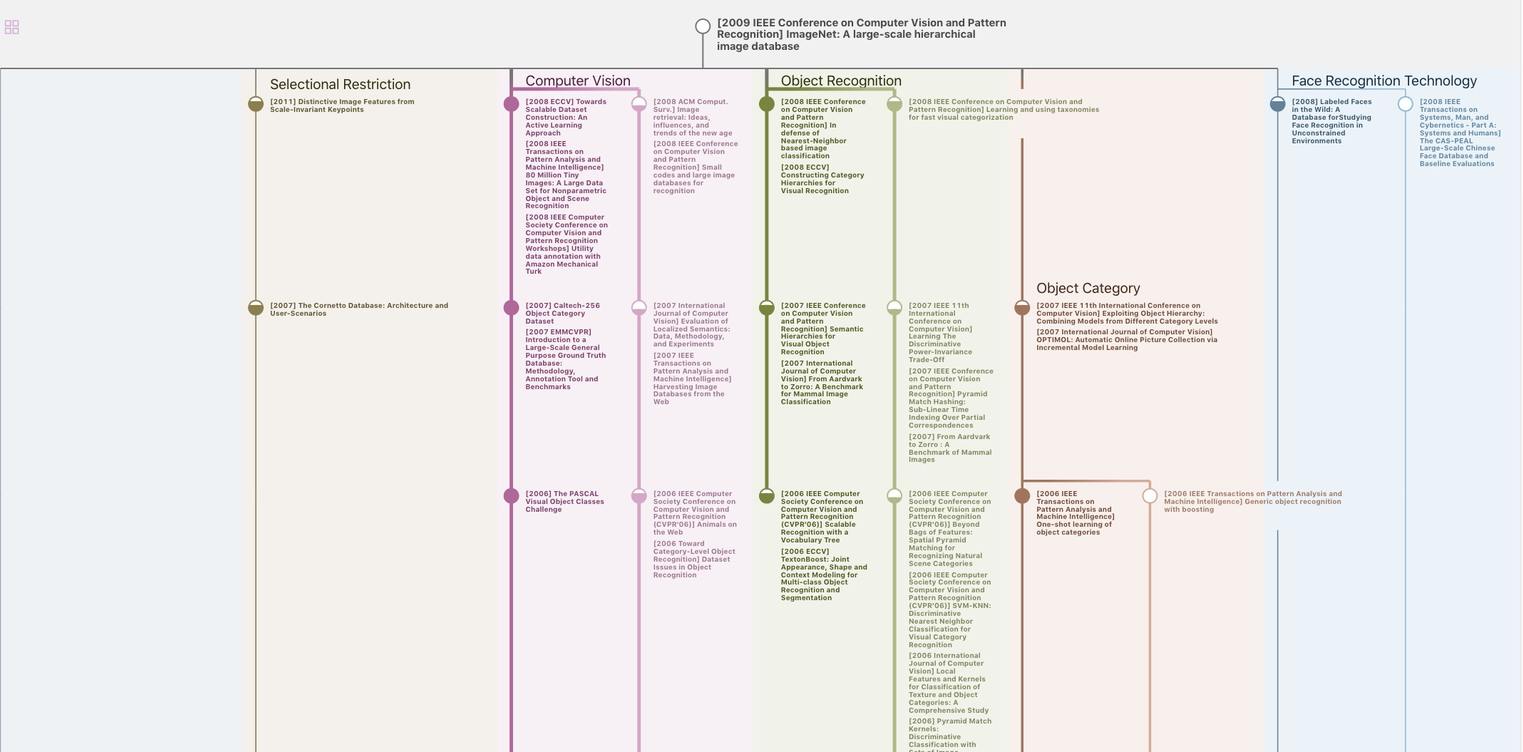
生成溯源树,研究论文发展脉络
Chat Paper
正在生成论文摘要