Causal Analysis of the Factors Influencing Vehicle Following Distance Using Immersive Virtual Environments
INTERNATIONAL CONFERENCE ON TRANSPORTATION AND DEVELOPMENT 2023: TRANSPORTATION SAFETY AND EMERGING TECHNOLOGIES(2023)
摘要
Identification of factors affecting the vehicle following-distance behavior in the context of improving safety and reducing crashes is of utmost importance. However, earlier research on the factors influencing following-distance behavior has primarily relied on correlation/associationbased techniques, which miss the underlying causal relations between the influencing factors and are therefore unable to identify the actual root causes. Therefore, the aim of this study is to create a causal model by using a framework for causal inference to identify the factors that contribute to participants' following-distance behavior and to quantitatively analyze their impacts. To realize this aim, we performed human-subject experiments in immersive virtual environments (IVE) integrated with a driving simulator to elicit participants' following-distance behavior data. IVEs provide users with a fully immersive simulation experience and allow researchers to easily design and manipulate contextual factors and observe the outcomes. As such, incorporating IVEs with causal inference into the following-distance behavior analysis helps to advance a more customizable research tool for investigating causal relationships in safety and traffic capacity studies. Six experimental scenarios involving several road-related factors and human-related factors were performed with 24 participants. Using the PC-stable algorithm and the elicitation method, a causal model was learnt from the data that visualized the causal relationships between the factors. The findings of this analysis suggest that the traffic conditions (i.e., normal, medium, and heavy traffic) have a causal effect on the participants' following-distance behavior, thus providing an extension to the previous studies where only correlations/associations were observed between those factors.
更多查看译文
AI 理解论文
溯源树
样例
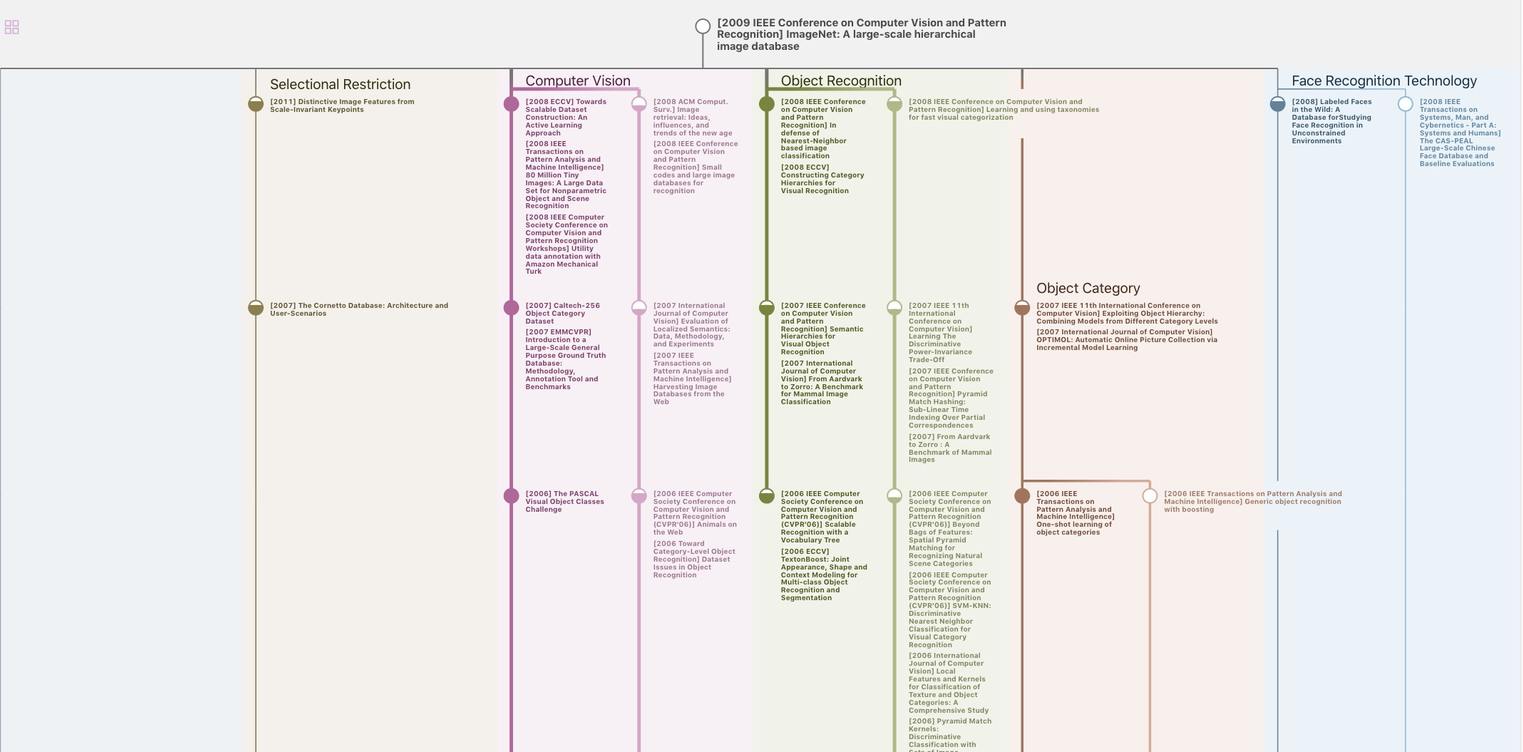
生成溯源树,研究论文发展脉络
Chat Paper
正在生成论文摘要