A Novel Prototype-Assisted Contrastive Adversarial Network for Weak-Shot Learning With Applications: Handling Weakly Labeled Data
IEEE-ASME TRANSACTIONS ON MECHATRONICS(2024)
摘要
This article is concerned with weak-shot learning, a practical yet challenging scenario in transfer learning where only a limited amount of weakly labeled data are available in the target domain. The key insights of weak-shot learning are focused on assigning fine-grained labels to target data and matching class-specific features across domains. In this article, a new prototype-assisted contrastive adversarial (PACA) network for weak-shot learning is proposed to make full use of the deterministic information from well-annotated data and the auxiliary information from weakly annotated data. Specifically, a prototypical pseudolabel learning mechanism is introduced to improve the credibility and robustness of pseudolabel estimation by fully exploiting prototype representations and weakly supervised information. Furthermore, a contrastive adversarial discrepancy strategy is developed to simultaneously reduce domain gaps at the global and local levels, providing compact intraclass features and distinguishable interclass features for weak-shot learning. The prototypical pseudolabel learning and contrastive adversarial discrepancy are designed to be updated alternately to eliminate pseudolabel noise in the target domain, which helps improve the transferability of domain-invariant features. Finally, extensive experiments are conducted on the cross-domain tasks of pipeline fault diagnosis, indicating that the proposed PACA network provides a promising tool for this practical industrial problem.
更多查看译文
关键词
Contrastive adversarial discrepancy,pipeline fault diagnosis,prototype-assisted contrastive adversarial network,prototypical pseudolabel learning,weak-shot learning
AI 理解论文
溯源树
样例
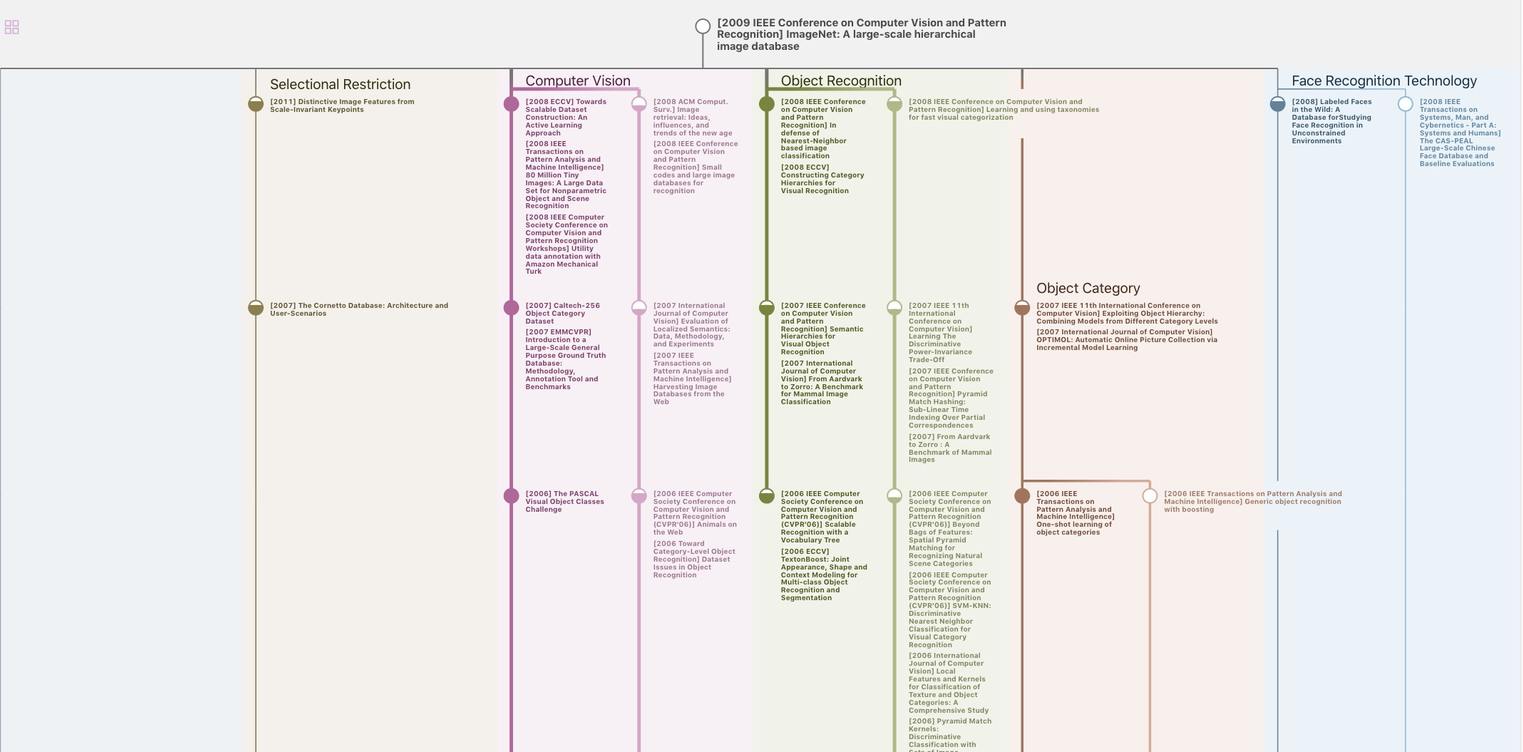
生成溯源树,研究论文发展脉络
Chat Paper
正在生成论文摘要