Learning Dynamic Dependencies With Graph Evolution Recurrent Unit for Stock Predictions
IEEE TRANSACTIONS ON SYSTEMS MAN CYBERNETICS-SYSTEMS(2023)
摘要
Investment decisions and risk management require understanding the time-varying dependencies between stocks. Graph-based learning systems have emerged as a promising approach for predicting stock prices by leveraging interfirm relationships. However, existing methods rely on a static stock graph predefined from finance domain knowledge and large-scale data engineering, which overlooks the dynamic dependencies between stocks. In this article, we present a novel framework called graph evolution recurrent unit (GERU), which uses a dynamic graph neural network to automatically learn the evolving dependencies from historical stock features, leading to better predictions. Our approach consists of three parts: first, we develop an adaptive dynamic graph learning (ADGL) module to learn latent dynamic dependencies from stock time series. Second, we propose a clustered ADGL (clu-ADGL) to handle large-scale time series by reducing time and memory complexity. Third, we combine the ADGL/clu-ADGL with a graph-gated recurrent unit to model the temporal evolutions of stock networks. Extensive experiments on real-world datasets show that our proposed methods outperform existing methods in predicting stock movements, capturing meaningful dynamic dependencies and temporal evolution patterns from the financial market, and achieving outstanding profitability in portfolio construction.
更多查看译文
关键词
Gated recurrent unit, graph representation learning, learning dynamic dependencies, stock prediction system
AI 理解论文
溯源树
样例
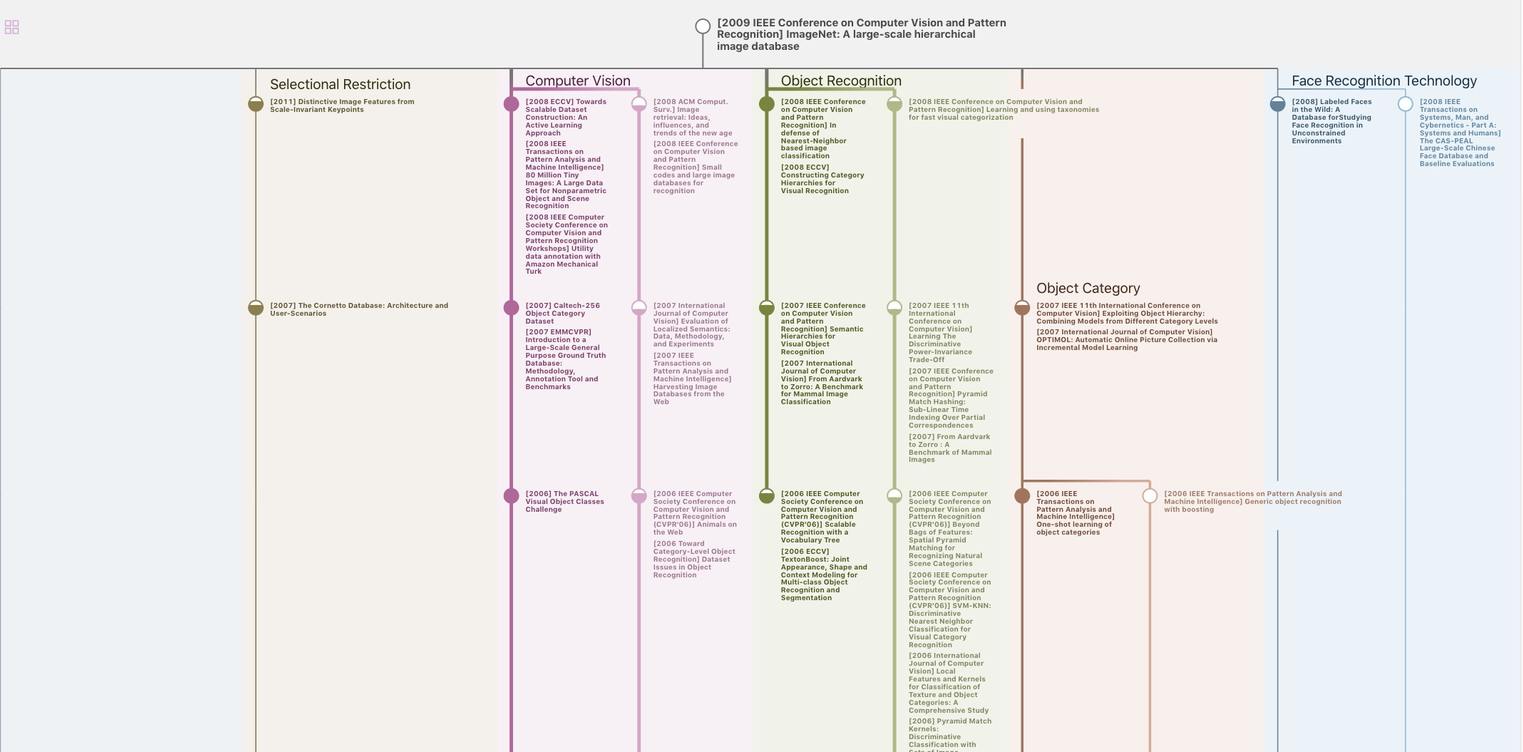
生成溯源树,研究论文发展脉络
Chat Paper
正在生成论文摘要