Rydberg sensors and machine learning assisted design discovery
FERROELECTRICS(2023)
摘要
Recent developments in Machine Learning (ML) models that purport to automate material discovery are explored, with a treatment of the logical extension of this work that we have defined as "Design Discovery". If we use the Rydberg sensor as a representative target for modeling with advanced ML tools and algorithms, the definition of a sufficient feature set to establish an ML model requires the quantum mechanical description of electrical and magnetic interactions that leverage Stark effect observations and Rydberg states of the targeted atom, as well as other forces internal and external to the atomic structure. This provides a feature rich environment from the standpoint of predictive design using Machine Learning models. The paper concludes with a theoretical treatment of a Rydberg sensor and its potential sensitivity to measurement of extremely small electromagnetic phenomena, with an initial consideration of potential variations of existing experiments that can be "discovered" from an appropriately defined ML design discovery model.
更多查看译文
关键词
Machine learning,design discovery,predictive design,Rydberg sensor,quantum model,quantum computing,Variational Quantum Eigensolver (VQE)
AI 理解论文
溯源树
样例
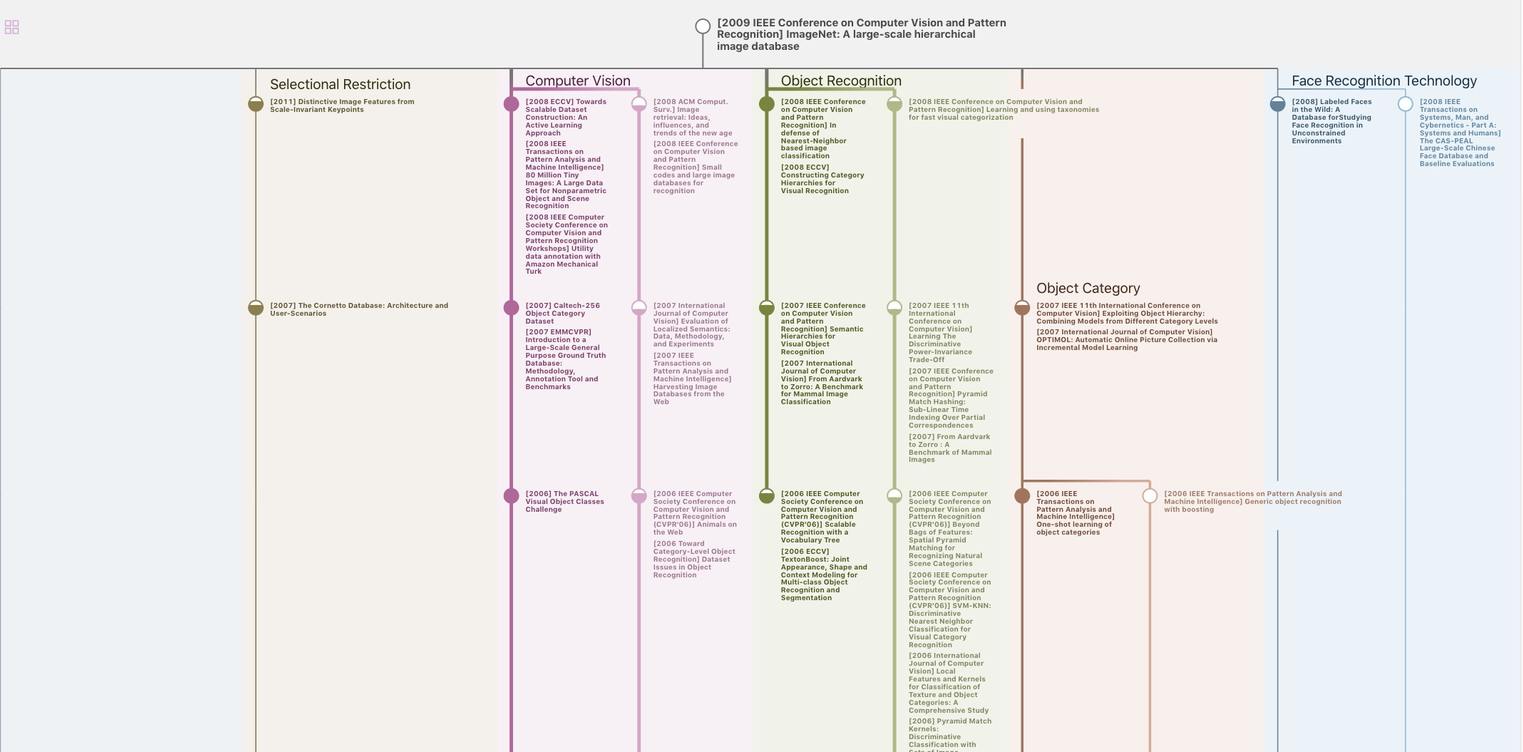
生成溯源树,研究论文发展脉络
Chat Paper
正在生成论文摘要