Statistical inference and distributed implementation for linear multicategory SVM
STAT(2023)
摘要
Support vector machine (SVM) is one of the most prevalent classification techniques due to its excellent performance. The standard binary SVM has been well-studied. However, a large number of multicategory classification problems in the real world are equally worth attention. In this paper, focusing on the computationally efficient multicategory angle-based SVM model, we first study the statistical properties of model coefficient estimation. Notice that the new challenges posed by the widespread presence of distributed data, this paper further develops a distributed smoothed estimation for the multicategory SVM and establishes its theoretical guarantees. Through the derived asymptotic properties, it can be seen that our distributed smoothed estimation can achieve the same statistical efficiency as the global estimation. Numerical studies are performed to demonstrate the highly competitive performance of our proposed distributed smoothed method.
更多查看译文
关键词
asymptotic properties, Bahadur representation, distributed implementation, kernel smoothing, linear multicategory SVM
AI 理解论文
溯源树
样例
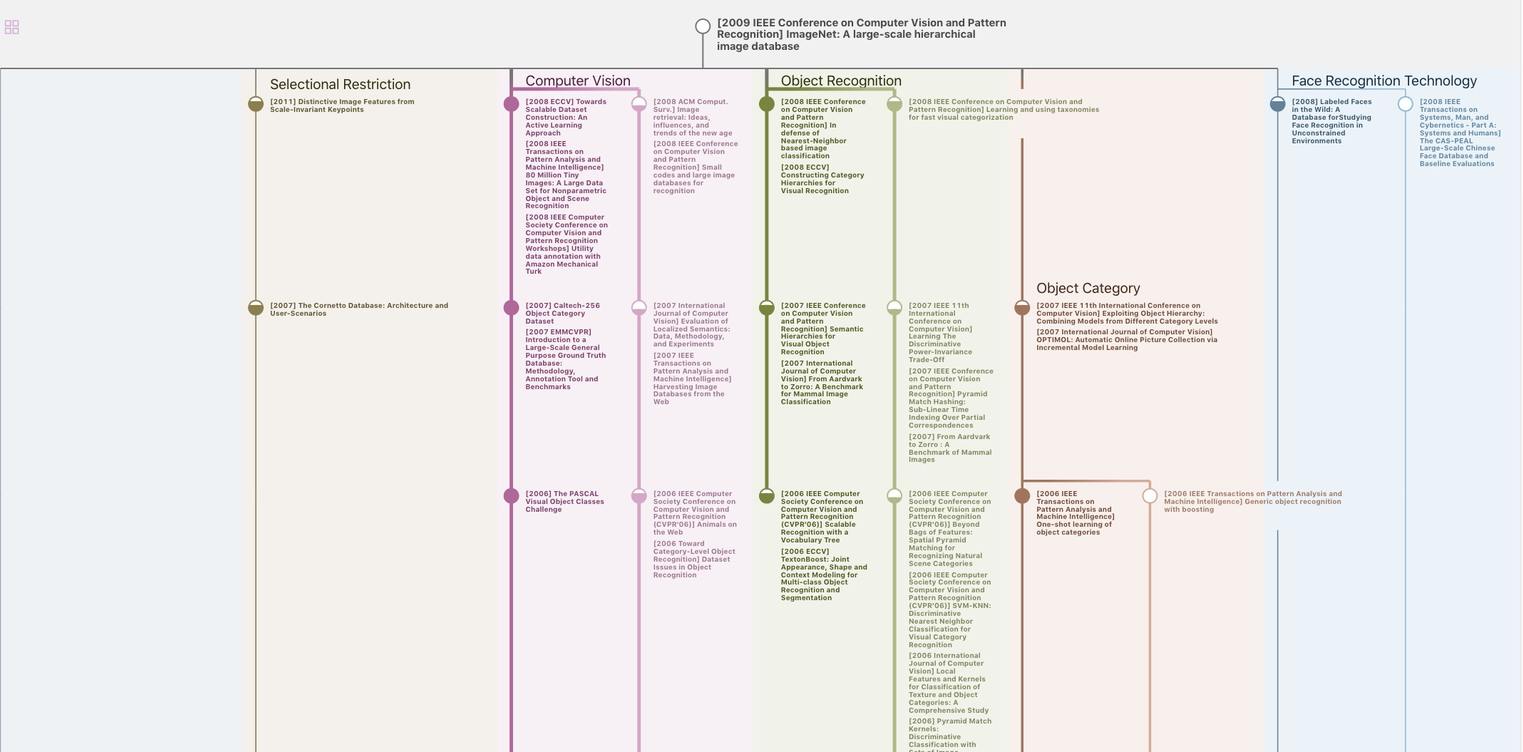
生成溯源树,研究论文发展脉络
Chat Paper
正在生成论文摘要