Self-supervised knowledge mining from unlabeled data for bearing fault diagnosis under limited annotations
MEASUREMENT(2023)
摘要
Deep learning has become a popular approach for fault diagnosis due to its powerful feature extraction and adaptability. However, its reliance on extensive annotations poses challenges in real-world applications. To confront this issue, this article proposes the CLTrans, a contrastive learning-based knowledge transfer method for semi-supervised fault diagnosis. CLTrans utilizes large-scale unlabeled data to benefit downstream tasks by simply performing unsupervised similarity matching. A feature encoder pre-trained by CLTrans can extract discriminative representations of vibration signals and can efficiently adapt to various tasks, even with data under different distributions. Experimental results of inner-dataset and inter-dataset knowledge transfer demonstrate that CLTrans outperforms conventional deep learning and state-of-the-art semi-supervised fault diagnosis approaches in terms of accuracy and domain adaptability, especially under limited labels. The capability of unsupervised knowledge mining and transfer allows for reducing the burden of data collection and annotation.
更多查看译文
关键词
Contrastive learning,Fault diagnosis,Knowledge transfer,Semi-supervised learning
AI 理解论文
溯源树
样例
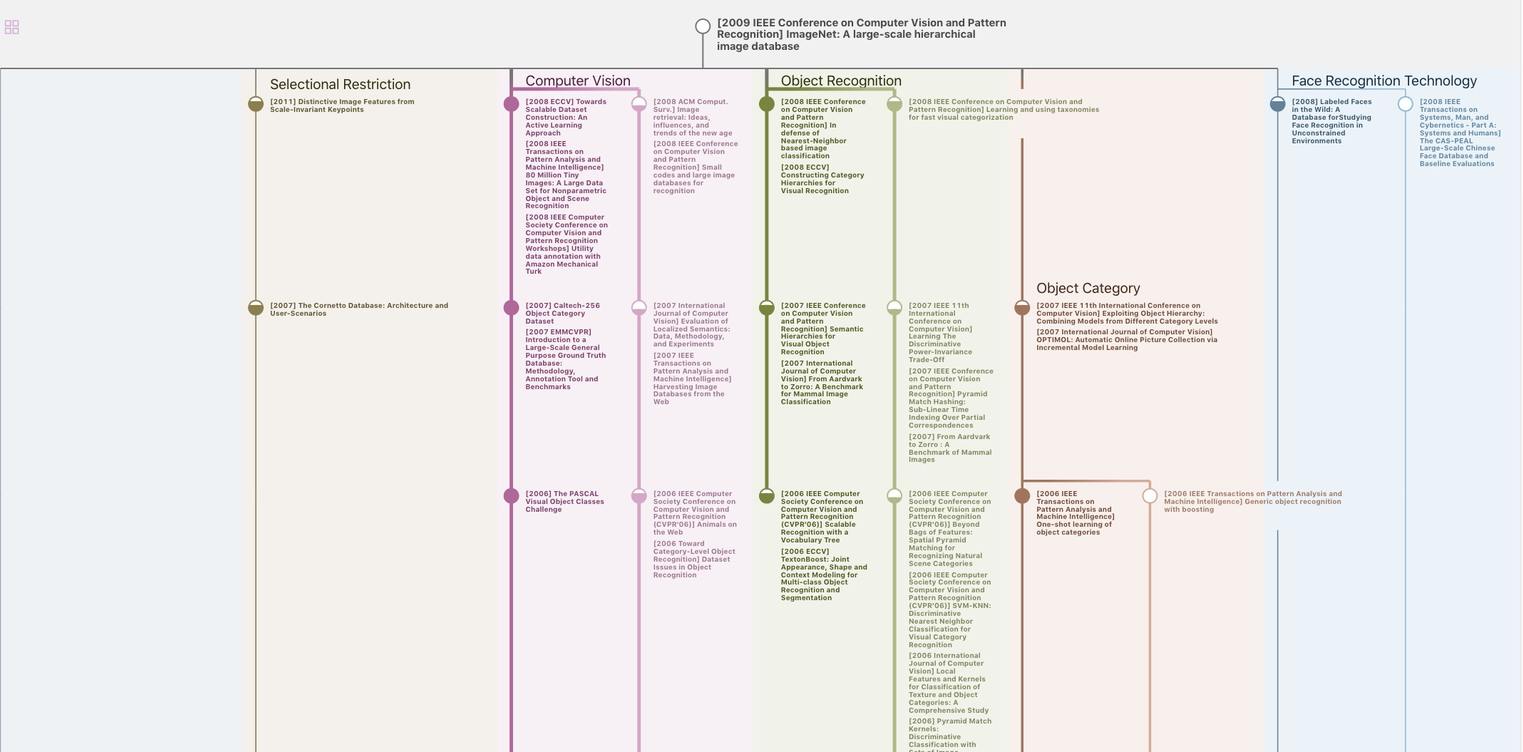
生成溯源树,研究论文发展脉络
Chat Paper
正在生成论文摘要