A Multiview Sparse Dynamic Graph Convolution-Based Region-Attention Feature Fusion Network for Major Depressive Disorder Detection
IEEE TRANSACTIONS ON COMPUTATIONAL SOCIAL SYSTEMS(2023)
摘要
Detecting and diagnosing major depressive disorder (MDD) is greatly crucial for appropriate treatment and support. In recent years, there have been efforts to develop automated methods for depression detection using machine learning techniques, which mainly analyze various data sources such as text, speech, and social media posts. However, the effectiveness and reliability of these methods may vary and more importantly, they fail to provide timely intervention and treatment to MDD patients. To address these challenges, we propose a novel electroencephalogram (EEG)-based MDD detection framework, which is named as multiview sparse dynamic graph convolution-based region-attention feature fusion network (MV-SDGC-RAFFNet). Specifically, we first design a multiview (MV) feature extractor to concurrently characterize EEG signals from temporal, spectral, and time-frequency views, providing rich semantic information on the emotional status of patients. Secondly, we introduce a sparse dynamic graph convolution network (SDGCN) to map the multidomain features into high-level representations, which avoids the limitation of over-smoothing and redundant edges existing in the conventional graph neural networks (GNNs). Finally, to efficiently fuse multidomain features, we propose a region-attention feature fusion network (RAFFNet), which applies different attention weights for brain regions and is greatly beneficial to boost the accuracy (ACC) of MDD detection. We validate the efficacy of the proposed MV-SDGC-RAFFNet framework on two public MDD datasets, and it achieves more promising detection performance against the state-of-the-art methods, indicating that our method has a prospect on clinical MDD detection.
更多查看译文
关键词
Electroencephalogram (EEG),graph neural network (GNN),major depressive disorder (MDD),multidomain feature extraction
AI 理解论文
溯源树
样例
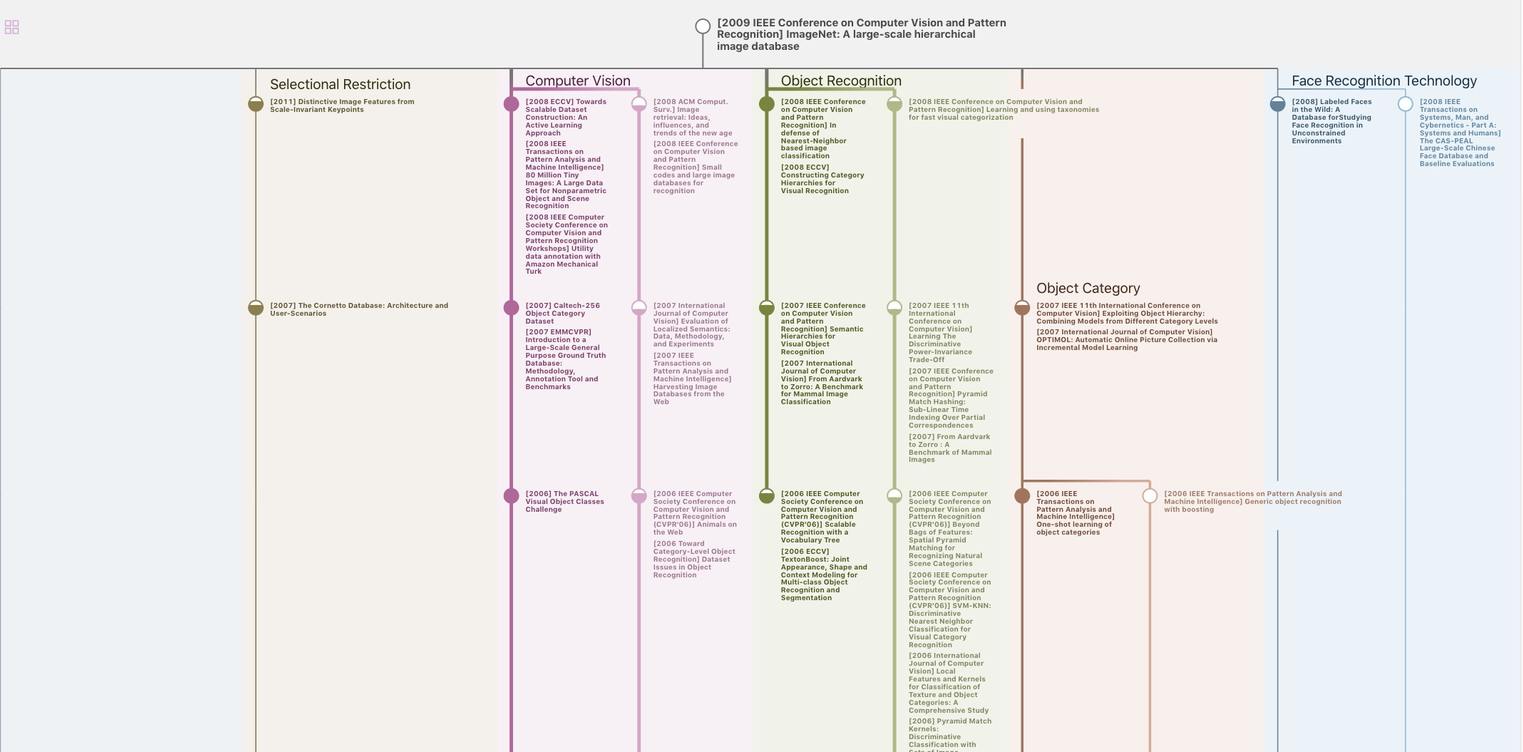
生成溯源树,研究论文发展脉络
Chat Paper
正在生成论文摘要