Sparsity enhanced MRF algorithm for automatic object detection in GPR imagery
MATHEMATICAL BIOSCIENCES AND ENGINEERING(2023)
摘要
This study addressed the problem of automated object detection from ground penetrating radar imaging (GPR), using the concept of sparse representation. The detection task is first formu-lated as a Markov random field (MRF) process. Then, we propose a novel detection algorithm by introducing the sparsity constraint to the standard MRF model. Specifically, the traditional approach finds it difficult to determine the central target due to the influence of different neighbors from the imaging area. As such, we introduce a domain search algorithm to overcome this issue and increase the accuracy of target detection. Additionally, in the standard MRF model, the Gibbs parameters are empirically predetermined and fixed during the detection process, yet those hyperparameters may have a significant effect on the performance of the detection. Accordingly, in this paper, Gibbs parameters are self-adaptive and fine-tuned using an iterative updating strategy followed the concept of sparse rep-resentation. Furthermore, the proposed algorithm has then been proven to have a strong convergence property theoretically. Finally, we verify the proposed method using a real-world dataset, with a set of ground penetrating radar antennas in three different transmitted frequencies (50 MHz, 200 MHz and 300 MHz). Experimental evaluations demonstrate the advantages of utilizing the proposed algorithm to detect objects in ground penetrating radar imagery, in comparison with four traditional detection algorithms.
更多查看译文
关键词
Ground penetrating radar (GPR) imaging, object detection, Markov random field, sparse representation
AI 理解论文
溯源树
样例
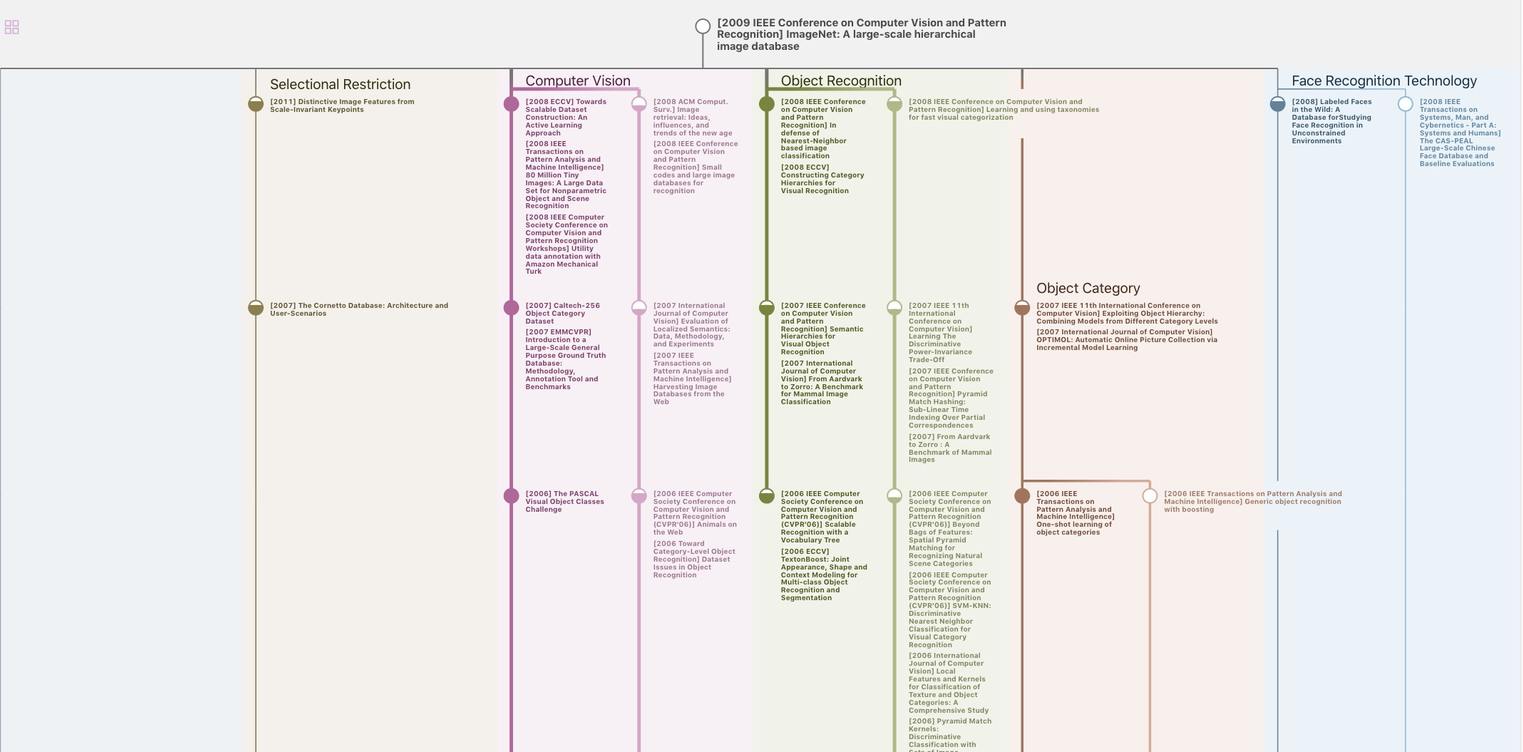
生成溯源树,研究论文发展脉络
Chat Paper
正在生成论文摘要