Multiscale Representation of Radar Echo Data Retrieved through Deep Learning from Numerical Model Simulations and Satellite Images
REMOTE SENSING(2023)
摘要
Radar reflectivity data snapshot fine-grained atmospheric variations that cannot be represented well by numerical weather prediction models or satellites, which poses a limit for nowcasts based on model-data fusion techniques. Here, we reveal a multiscale representation (MSR) of the atmosphere by reconstructing the radar echoes from the Weather Research and Forecasting (WRF) model simulations and the Himawari-8 satellite products using U-Net deep networks. Our reconstructions generated the echoes well in terms of patterns, locations, and intensities with a root mean square error (RMSE) of 5.38 dBZ. We find stratified features in this MSR, with small-scale patterns such as echo intensities sensitive to the WRF-simulated dynamic and thermodynamic variables and with larger-scale information about shapes and locations mainly captured from satellite images. Such MSRs with physical interpretations may inspire innovative model-data fusion methods that could overcome the conventional limits of nowcasting.
更多查看译文
关键词
deep learning, multiscale representation, model-data fusion
AI 理解论文
溯源树
样例
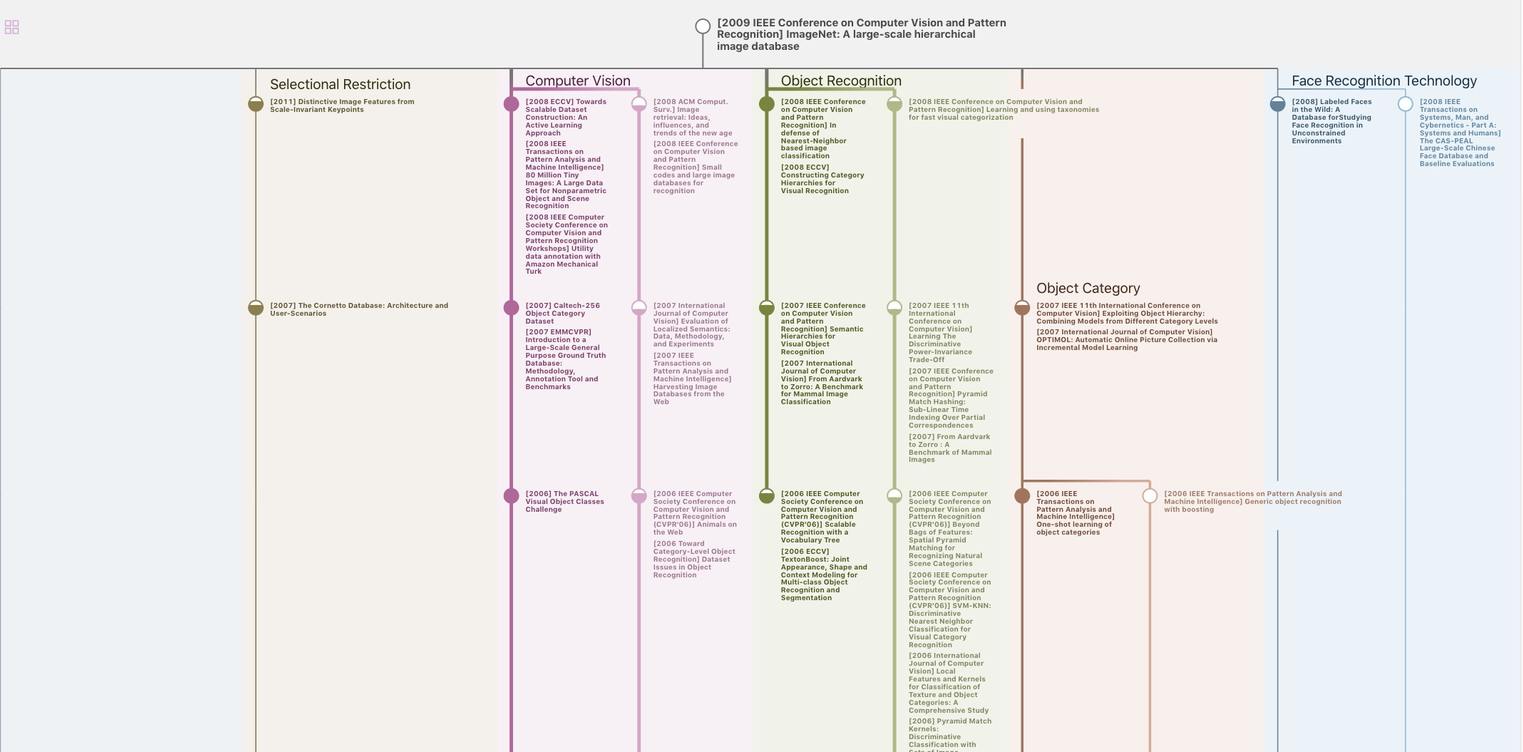
生成溯源树,研究论文发展脉络
Chat Paper
正在生成论文摘要