Connecting Large-Scale Meteorological Patterns to Extratropical Cyclones in CMIP6 Climate Models Using Self-Organizing Maps
EARTHS FUTURE(2023)
摘要
Extratropical cyclones (ETCs) are responsible for the majority of cool-season extreme events in the northeastern United States (NEUS), often leading to high-impact weather conditions that can have wide-ranging socioeconomic impacts. Evaluating the ability of climate models to adequately simulate ETC dynamics is essential for improving model performance and increasing confidence in future projections used by stakeholders and policymakers. ETCs are traditionally studied using techniques such as case studies and synoptic typing, however, these approaches can be time-consuming, require subjective analysis, and do not necessarily identify the coincident large-scale meteorological patterns (LSMPs). Here, we apply self-organizing maps (SOMs) as an automated machine-learning approach to characterize the LSMPs and associated frequency and intensity of discrete ETC events over NEUS. The dominant patterns of geopotential height variability are identified through SOM analysis of five reanalysis products during the last four decades. ETC events are tracked using TempestExtremes and are integrated with SOMs to classify the accumulated cyclone activity (ACA) associated with each pattern. We then evaluate the skill of CMIP6 historical experiments in simulating the LSMPs and ETC events identified in the SOM. Our results identify a robust bias toward more zonal patterns, with models struggling to reproduce the more amplified patterns typically associated with the highest cyclone activity. While model resolution has some impact on simulation credibility, model configuration appears to be more important in LSMP representation. The vast majority of CMIP6 models produce too few ETCs, although model errors are distributed around historical reanalyses when ACA is normalized by storm frequency.
更多查看译文
关键词
extratropical cyclones, CMIP6, self-organizing maps
AI 理解论文
溯源树
样例
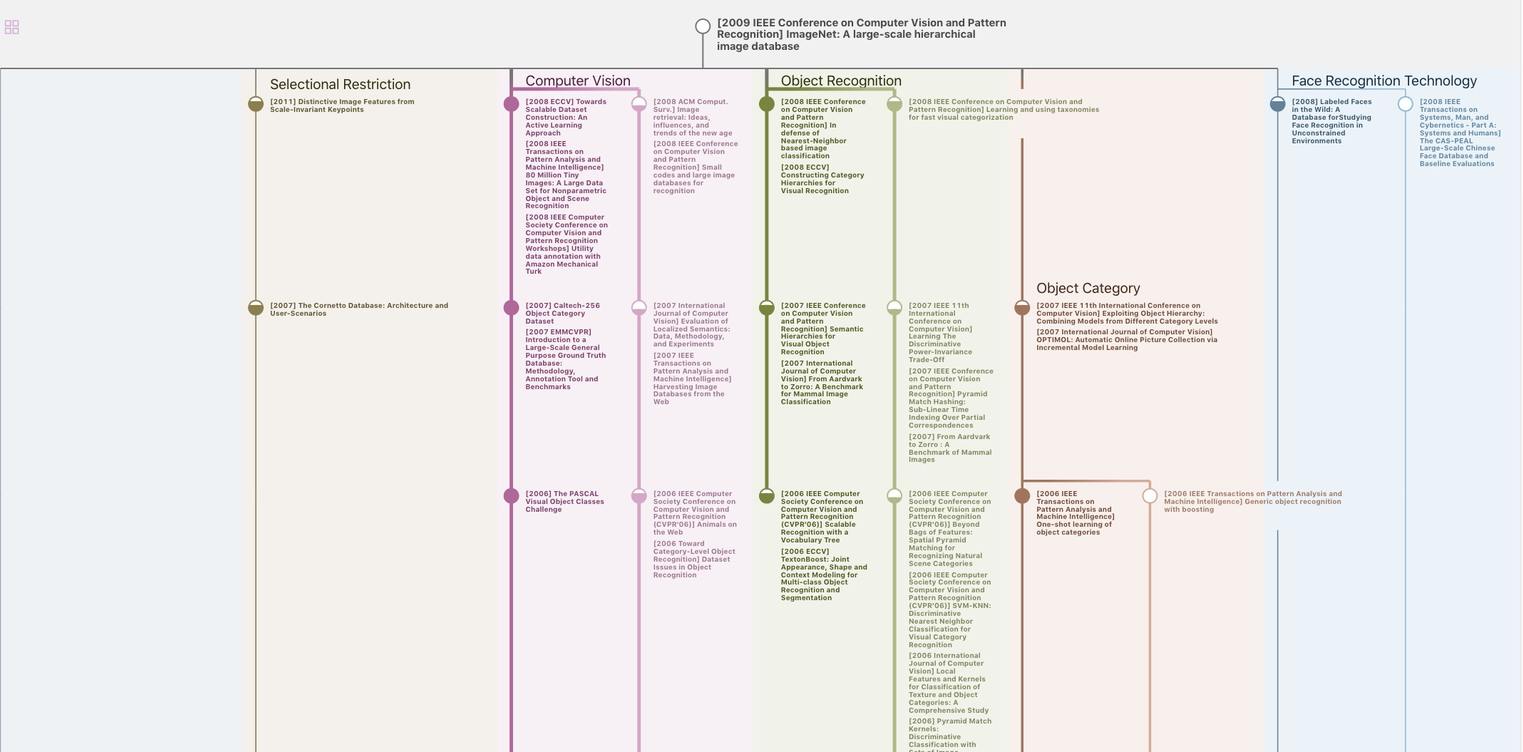
生成溯源树,研究论文发展脉络
Chat Paper
正在生成论文摘要