Semllldsnet: semi-supervised learning based limited labeled data deep segmentation network for covid-19 ct images
JOURNAL OF NONLINEAR AND CONVEX ANALYSIS(2023)
摘要
The accurate segmentation of lesions in coronavirus disease 2019(CO VID-19) computed tomography (CT) images can help doctors detect disease infection and control the condition. Hence, this study proposed a deep learning segmentation network with limited labeled data (LLDSNet). The problem of lesion segmentation in COVID-19 poses a major challenge due to the different morphology of lesions in different CT images, lesions with dispersion, small lesion area, background area imbalance, blurring of the boundary of lesions and normal areas, and so forth. The contextual feature-aware module can effectively extract the overall and local features, and the shape edge detection module can accurately find the edges of the lesion area to segment the lesions in this area. The hybrid loss function is used to avoid the class imbalance problem and improve the overall network performance. It was demonstrated that LLDSNet achieved a Dice coefficient of 0.696 for a small number of data set. We propose a semi-supervised segmentation network Semi1,LDSNet due to the shortage of labeled data.. The experiments were performed on the created Semi-CCCT dataset. The results indicated a significant enhancement in the performance of semi-supervised segmentation in comparison to fully supervised segmentation, with a Dice of 0.717. The proposed Semi_LLDSNet outperformed the five other segmentation networks tested.
更多查看译文
关键词
Computer-aided, COVID-19, segmentation, deep learning, feature-aware, semi-supervised, edge detection
AI 理解论文
溯源树
样例
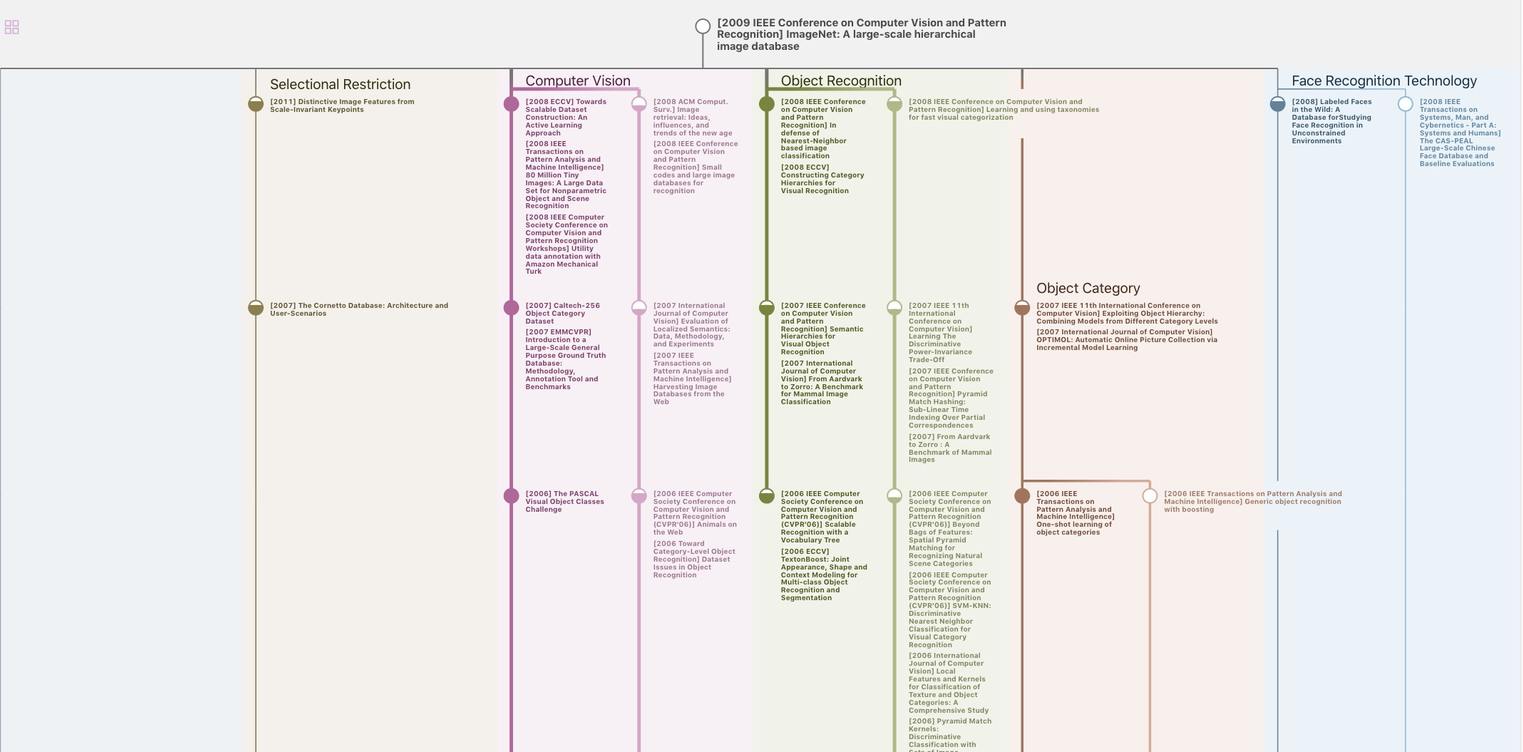
生成溯源树,研究论文发展脉络
Chat Paper
正在生成论文摘要