Dual Plug and Play Multi-Energy Computed Tomography Reconstruction Algorithm
ACTA OPTICA SINICA(2023)
摘要
Objective Multi-energy computed tomography (MECT) technology uses multiple energy X-rays to scan an object, facilitating energy resolution imaging for material attenuation distribution of the object. In addition, the MECT effectively suppresses hardening artifacts and quantitatively analyzes material components, suggesting its broad application prospects in the field of medical diagnosis. Currently, MECT imaging systems have mainly four scanning schemes: multiple scanning systems, fast rapid voltage switching systems, multi-source systems, and photon counting detector systems. However, these approaches are associated with incomplete data and noise caused by physical effects, such as photon scattering, pulse stacking, and charge sharing. Therefore, there is a need to design efficient and feasible reconstruction algorithms to effectively address the above issues. In this regard, this work proposes a dual plug and play (PnP) framework for MECT image reconstruction algorithm. Methods In order to optimize the model design, this work introduces L0 norm regularization in the image gradient domain and tensor weighted kernel norm regularization, which can effectively characterize the priori of MECT images. The regularization of the L0 norm in the image gradient domain expresses the sparsity of the gradient of a single channel image, while the tensor weighted kernel norm characterizes the correlation between multichannel images. Considering the flexibility and efficiency of the PnP framework in various imaging problems, this work proposes an efficient solution method based on the dual PnP framework and the alternating direction multiplier method. To verify the feasibility of the proposed algorithm, this work carried out multichannel photon counting simulation experiments. It also analyzed the performance of the proposed algorithm by adding random noise with different intensities to the projection data obtained under different sampling angles. Results and Discussions Two sets of experiments were conducted on a simulation dataset to verify the performance of the proposed algorithm on incomplete data and noise interference issues. With respect to incomplete data, this work collects projection data from 90 angles within a 360-degree scanning range and adds low-intensity noise to the collected projection data. To address noise interference, this work collects 140 angle projection data within a 360-degree scanning range and adds higher intensity noise to the collected projection data. Considering the noise caused by physical effects, such as photon scattering, pulse stacking, and charge sharing, this work adds random noise to the projection data and sets two different intensities of noise, i. e., 0. 024 and 0. 055, respectively. To further demonstrate the advantages of the dual PnP spectral CT reconstruction algorithm proposed in this paper, several representative reconstruction algorithms were selected for comparative experiments, namely, SART, ASD-POCS, and SISTER. In case of incomplete data, the proposed algorithm obtains higher quality reconstruction results, which can suppress noise while restoring the precise area of the object with high accuracy; whereas in case of noise interference, the proposed algorithm restores most of the details of the image and achieves higher accuracy reconstruction results than other contrast algorithms. Our findings show that the proposed algorithm outperforms the SISTER algorithm under different sampling conditions and noise levels. In addition, this work further examines the key performance indicators of the proposed algorithm from both the iterative characteristics and computational efficiency. Moreover, the computational efficiency of the proposed algorithm is improved by about three times compared with that of the SISTER algorithm. Conclusions In this work, a dual PnP framework MECT image reconstruction algorithm is proposed to reduce radiation dose and improve imaging efficiency. The feasibility of the proposed algorithm was verified using research experiments on incomplete data and noise interference issues. In optimizing the model design, this work introduces L0 norm regularization in the image gradient domain and tensor weighted kernel norm regularization, which can effectively represent a priori of MECT images. The regularization of the L0 norm in the image gradient domain expresses the sparsity of the gradient of a single channel image, while the tensor weighted kernel norm can effectively characterize the correlation between multichannel images. In designing the solution algorithm, this work proposes an efficient solution method based on the dual PnP framework and the alternating direction multiplier method. This method can effectively integrate the tensor weighted kernel norm and the computational method for minimizing the L0 norm in the image gradient domain. Our results show that the proposed method has advantages in imaging quality compared to SART, ASD-POCS, and SISTER algorithms. It also has improved computational efficiency compared to the SISTER algorithm.
更多查看译文
关键词
multi-energy computed tomography,low dose sampling,X-ray imaging,plug and play framework
AI 理解论文
溯源树
样例
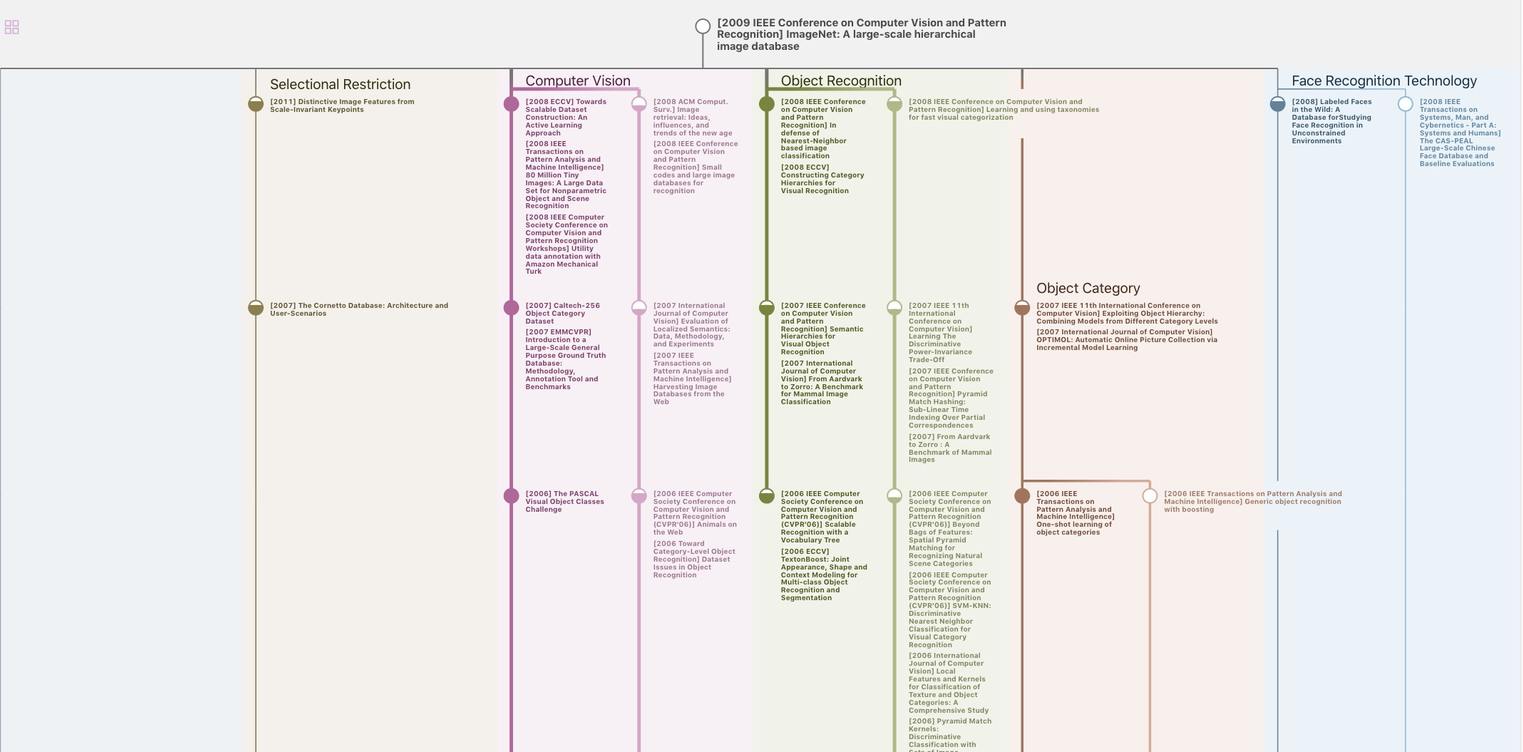
生成溯源树,研究论文发展脉络
Chat Paper
正在生成论文摘要