An Appearance-Motion Network for Vision-Based Crash Detection: Improving the Accuracy in Congested Traffic
IEEE TRANSACTIONS ON INTELLIGENT TRANSPORTATION SYSTEMS(2023)
摘要
Crash detection is of great significance for traffic emergency management. Video-based approaches can effectively save the manpower monitoring cost and have achieved promising results in recent studies. However, they sometimes fail to correctly identify crashes in congested traffic. To fill the gap, this paper proposes a novel appearance-motion network for improving video-based crash detection performance in congested traffic. The appearance-motion network utilizes two paralleled convolutional networks (i.e., an appearance network and a motion network) to extract both appearance features and motion features of crashes. To learn discriminative appearance features for differentiating crashes in congested traffic scene (CCT) with non-crashes in congested traffic scene (NCCT), an auxiliary network combined with a triplet loss are introduced to train the appearance network. To better capture crash motion features in congested traffic, an optical flow learner is built in the motion network and trained to extract more fine-grained motion information. Moreover, a temporal attention module is applied to enable the motion network to focus on valuable frames. Experimental results show that the proposed network achieves a state-of-the-art result on crash detection and the introduction of the three components (i.e., the auxiliary network, the optical flow learner and the temporal attention module) effectively reduces false alarm rate by 28.07% and miss rate by 27.08% on crash detection in congested traffic. Our dataset will be available at https://github.com/vvgoder/Dataset_for_crashdetection.
更多查看译文
关键词
Vision-based crash detection, congested traffic, appearance-motion network, optical flow learner, temporal attention module
AI 理解论文
溯源树
样例
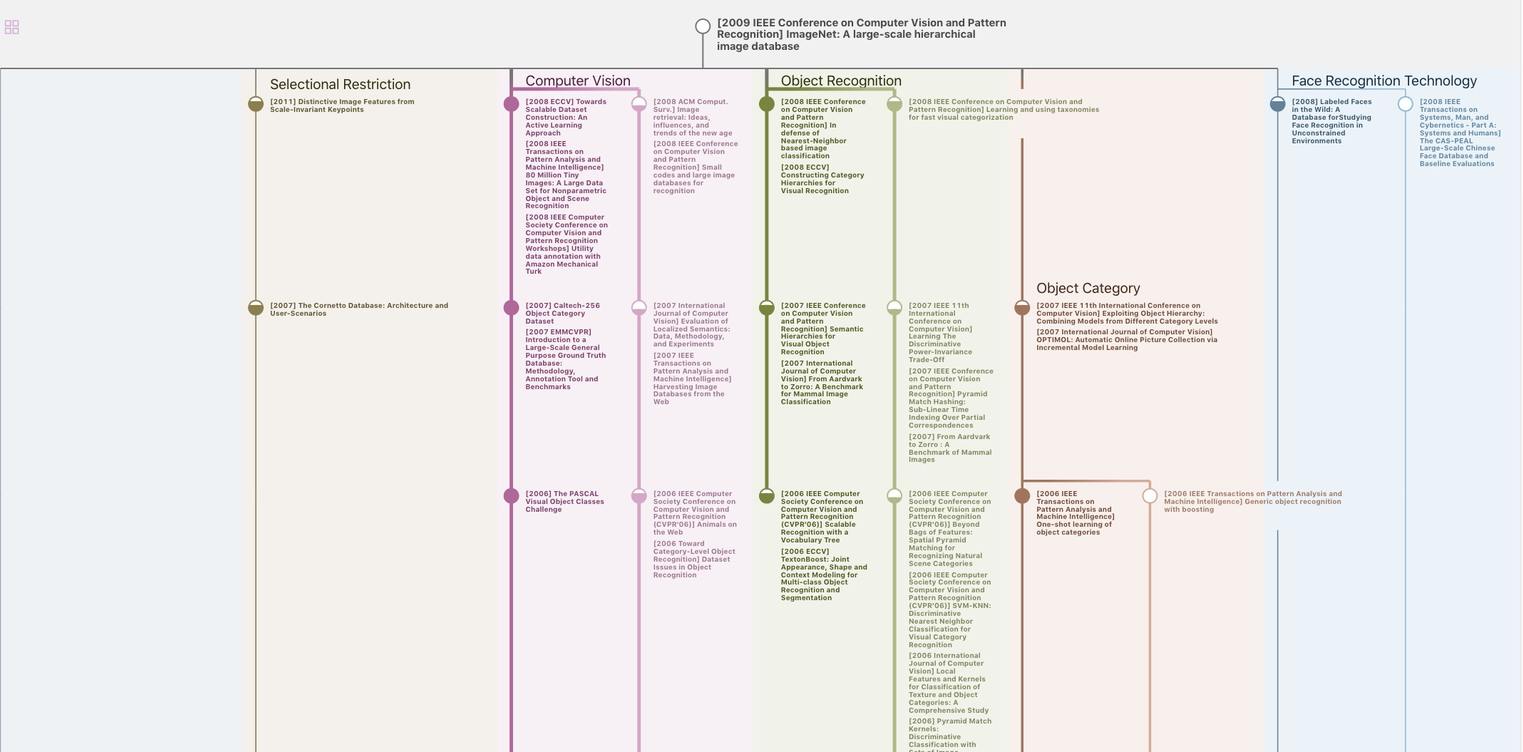
生成溯源树,研究论文发展脉络
Chat Paper
正在生成论文摘要