UWB NLOS Recognition Based on Improved Convolutional Neural Network Assisted by Wavelet Analysis and Gramian Angular Field
IEEE SENSORS JOURNAL(2023)
摘要
In the ultrawideband (UWB)-based indoor wireless positioning scene, nonline-of-sight (NLOS) is a common phenomenon due to the existence of obstacles and it may result in significant positioning errors. So, it is an important research issue to recognize the UWB NLOS signals from the line-of-sight (LOS) signals. As an emerging deep-learning technology, a convolutional neural network (CNN) has been applied successfully to handle this issue. However, the traditional CNN omits the influence of measurement noises and does not consider the mining of the signal's temporal relationship. To solve this problem, a UWB NLOS recognition method, called wavelet Gramian CNN (WGCNN), is proposed by integrating the wavelet analysis and the Gramian angular field (GAF). First, the discrete wavelet transform (DWT) is used to extract the trend curve of the channel impulse response (CIR) signals by eliminating the measurement noises. Furthermore, two GAFs, consisting of Gramian angular summation field (GASF) and Gramian angular difference field (GADF), are introduced to transform the 1-D CIR signal into the 2-D images, which describe the temporal correlation of the signals. Moreover, a GAF image reorganization strategy is designed to capture the key subimages to eliminate the influence of invalid image parts. Finally, the reorganized subimages are fed into the CNN model to build an LOS/NLOS classifier. The experiments are performed on a benchmark dataset provided by the EU "Horizon 2020" program, and the results show that the proposed WGCNN method has higher recognition accuracy than the traditional CNN method.
更多查看译文
关键词
Convolutional neural network (CNN),discrete wavelet transform (DWT),Gramian angular field (GAF),nonline-of-sight (NLOS)
AI 理解论文
溯源树
样例
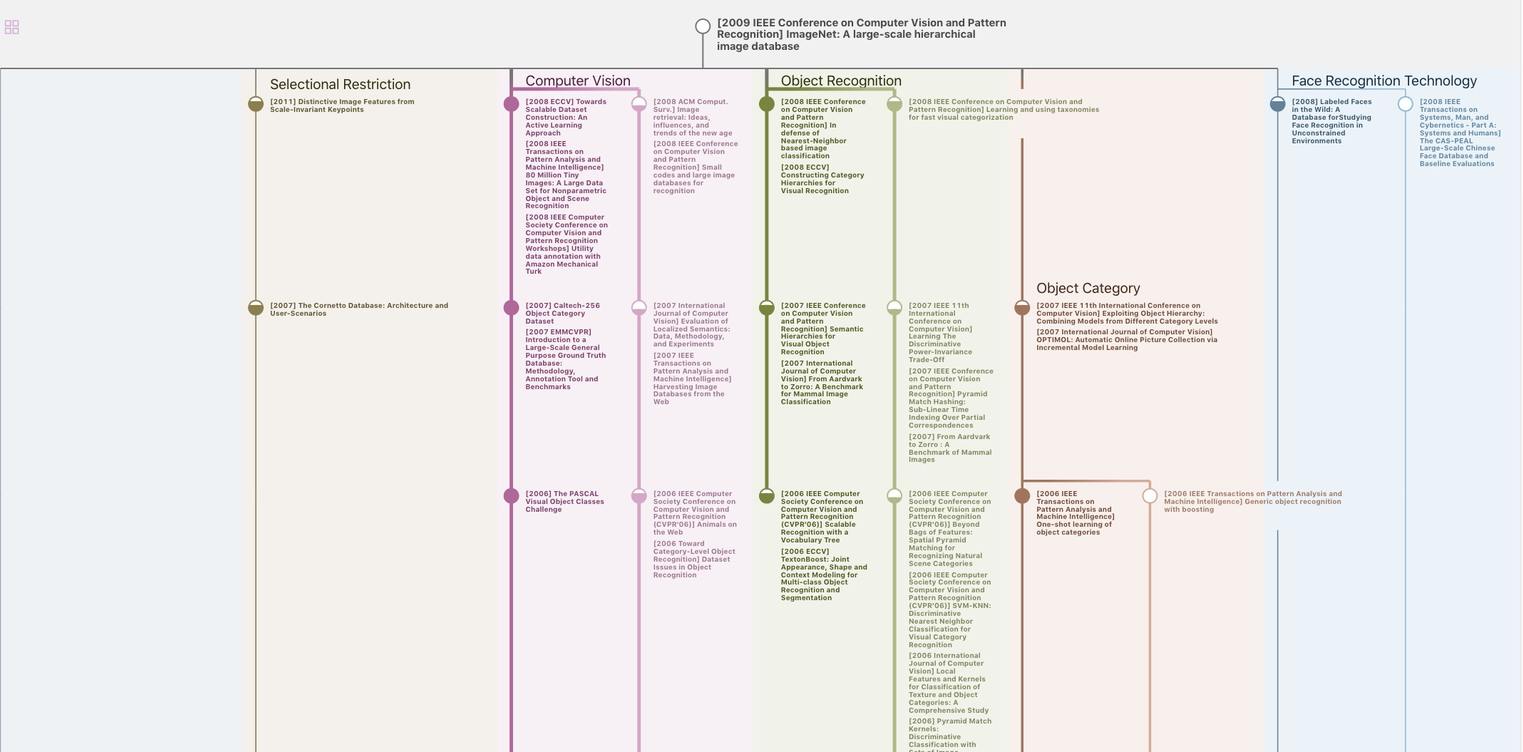
生成溯源树,研究论文发展脉络
Chat Paper
正在生成论文摘要