Joint estimation for SOC and capacity after current measurement offset redress with two-stage forgetting factor recursive least square method
JOURNAL OF POWER ELECTRONICS(2023)
摘要
To ensure the safe operation of electric vehicles (EVs), it is essential to estimate the internal status of lithium-ion batteries online. When current sensors are faulty, current measurement offset (CMO) interference occurs, and traditional state estimation algorithms become invalid due to incorrect current data. In this paper, a two-stage forgetting factor recursive least squares (FFRLS) algorithm is proposed for online identification of battery parameters and estimation of the CMO. Afterwards, a joint estimation framework is established to obtain the state of charge (SOC) and capacity with adaptive extended Kalman filter (AEKF) and iterative reweighted least squares (IRLS) algorithms, respectively. The open-source dataset of the CALCE Battery Research Group is used to verify the accuracy and robustness of the algorithm. The results show that the mean absolute error (MAE) of the CMO online estimation is less than 2.5 mA, the mean absolute percentage error (MAPE) of the SOC estimation is less than 2%, and the error in estimating the usable capacity is less than 2.5%.
更多查看译文
关键词
current measurement,joint estimation,recursive least square method,two-stage
AI 理解论文
溯源树
样例
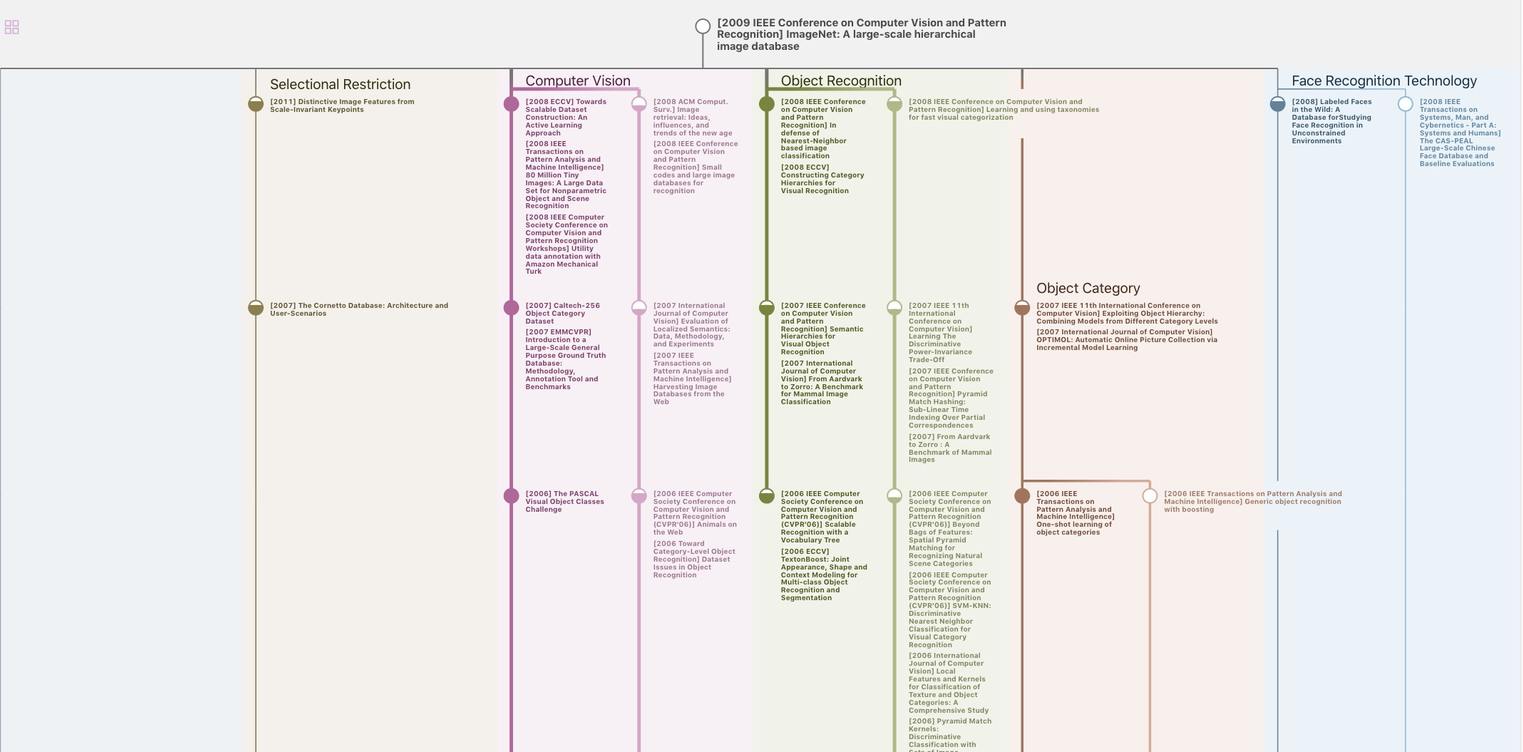
生成溯源树,研究论文发展脉络
Chat Paper
正在生成论文摘要