Classification of Camellia oleifera Diseases in Complex Environments by Attention and Multi-Dimensional Feature Fusion Neural Network
PLANTS-BASEL(2023)
摘要
The use of neural networks for plant disease identification is a hot topic of current research. However, unlike the classification of ordinary objects, the features of plant diseases frequently vary, resulting in substantial intra-class variation; in addition, the complex environmental noise makes it more challenging for the model to categorize the diseases. In this paper, an attention and multidimensional feature fusion neural network (AMDFNet) is proposed for Camellia oleifera disease classification network based on multidimensional feature fusion and attentional mechanism, which improves the classification ability of the model by fusing features to each layer of the Inception structure and enhancing the fused features with attentional enhancement. The model was compared with the classical convolutional neural networks GoogLeNet, Inception V3, ResNet50, and DenseNet121 and the latest disease image classification network DICNN in a self-built camellia disease dataset. The experimental results show that the recognition accuracy of the new model reaches 86.78% under the same experimental conditions, which is 2.3% higher than that of GoogLeNet with a simple Inception structure, and the number of parameters is reduced to one-fourth compared to large models such as ResNet50. The method proposed in this paper can be run on mobile with higher identification accuracy and a smaller model parameter number.
更多查看译文
关键词
camellia oleifera diseases,feature fusion,classification,multi-dimensional
AI 理解论文
溯源树
样例
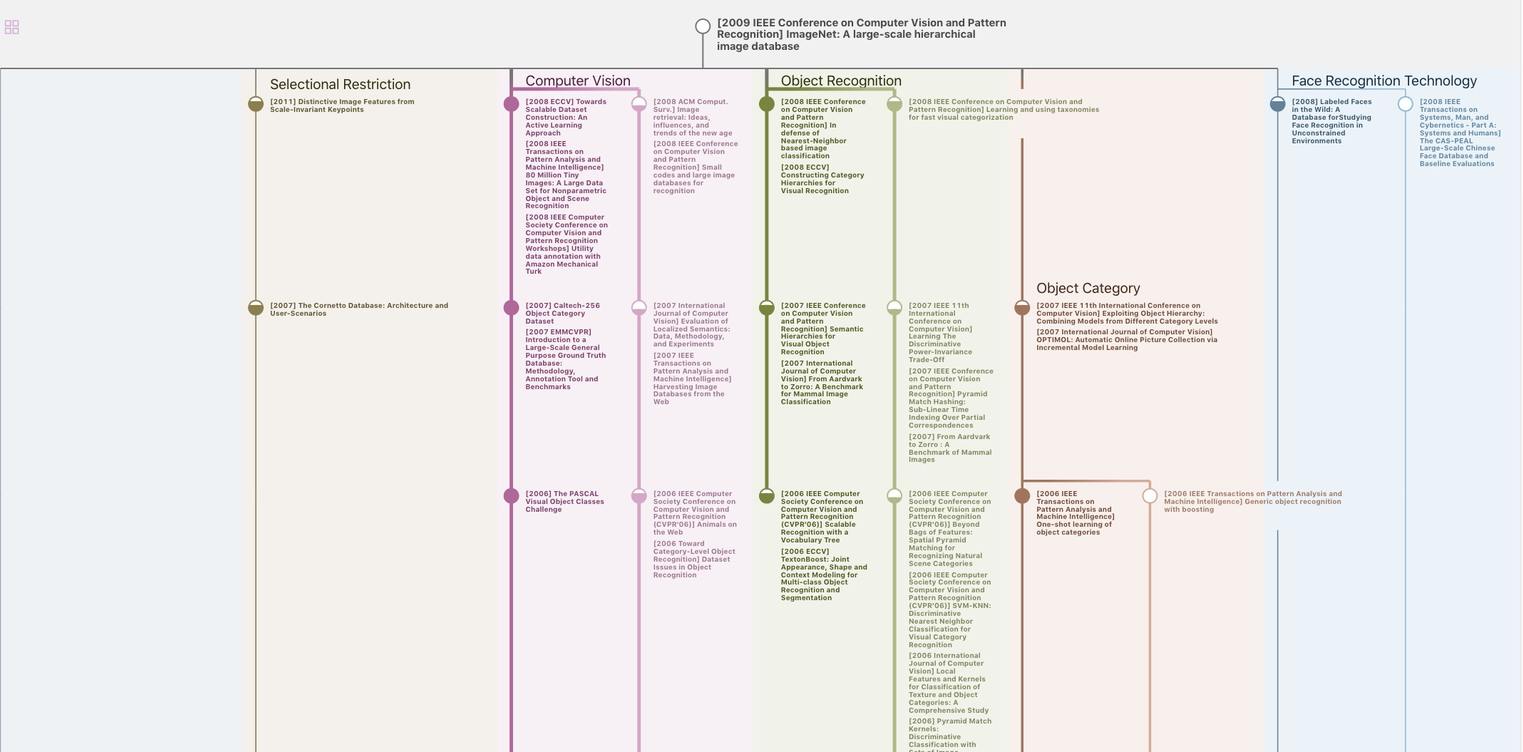
生成溯源树,研究论文发展脉络
Chat Paper
正在生成论文摘要