Determining the twist angle of stacked MoS2 layers using machine learning-assisted low-frequency interlayer Raman fingerprints
JOURNAL OF RAMAN SPECTROSCOPY(2023)
摘要
The investigation of twisted stacked few-layer MoS2 has revealed novel electronic, optical, and vibrational properties over an extended period. For the successful integration of twisted stacked few-layer MoS2 into a wide range of applications, it is crucial to employ a noninvasive, versatile technique for characterizing the layered architecture of these complex structures. In this work, we introduce a machine learning-assisted low-frequency Raman spectroscopy method to characterize the twist angle of few-layer stacked MoS2 samples. A feedforward neural network (FNN) is utilized to analyze the low-frequency breathing mode as a function of the twist angle. Moreover, using finite difference method (FDM) and density functional theory (DFT) calculations, we show that the low-frequency Raman spectra of MoS2 are mainly influenced by the effect of the nearest and second nearest layers. A new improved linear chain model (TA-LCM) with taking the twist angle into the consideration is developed to understand the interlayer breathing modes of stacked few-layer MoS2. This approach can be extended to other 2D materials systems and provides an intelligent way to investigate naturally stacked and twisted interlayer interactions.
更多查看译文
关键词
DFT,FDM,linear chain model,low-frequency Raman spectroscopy,twisted few-layer MoS2
AI 理解论文
溯源树
样例
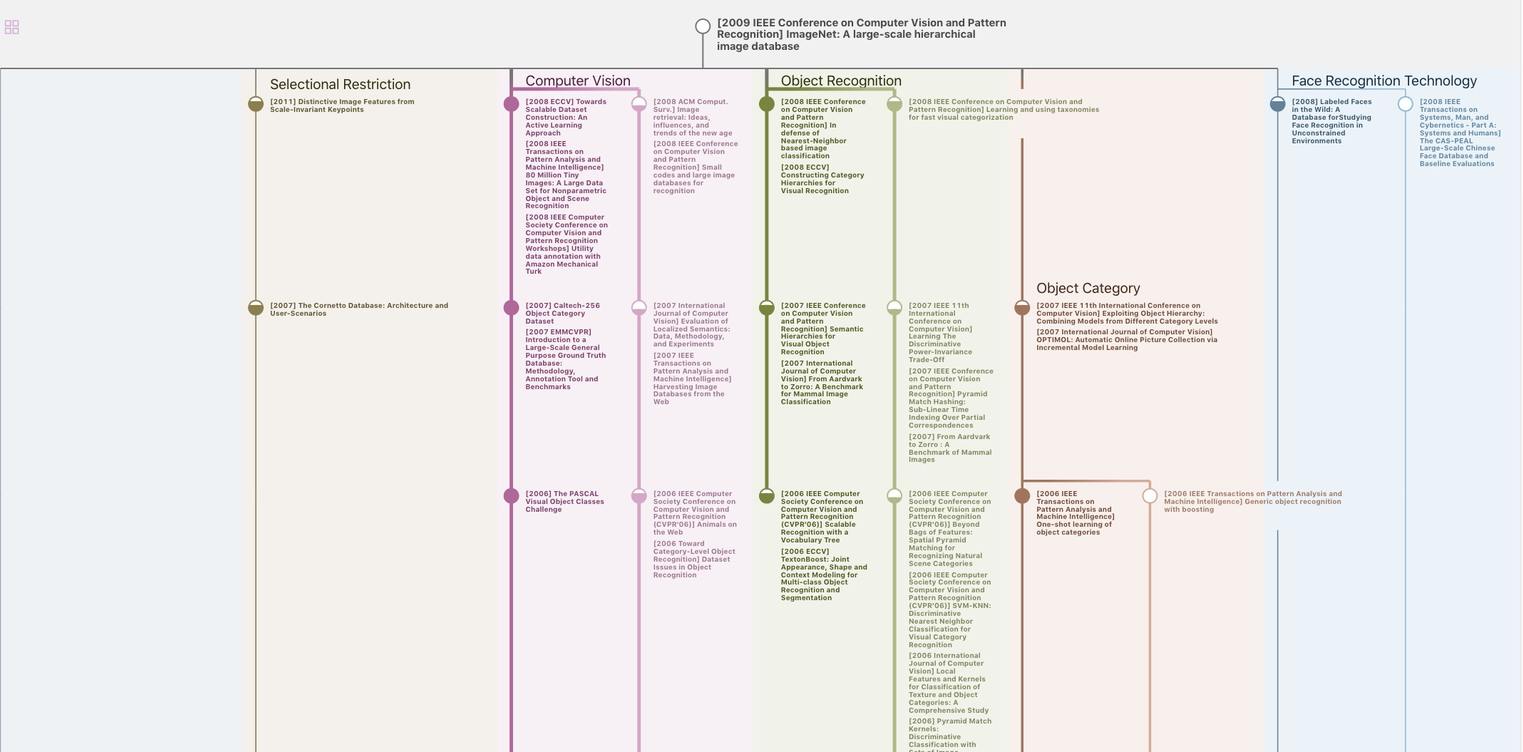
生成溯源树,研究论文发展脉络
Chat Paper
正在生成论文摘要