A Convolutional System Identification Approach Mixing Optimal Parameter Estimation and Deep Learning
INTERNATIONAL JOURNAL OF CONTROL AUTOMATION AND SYSTEMS(2023)
摘要
Experimental identification (EI) methods are often discussed in the literature because of the importance of building reliable dynamical models and control systems. This is especially true when thinking about autonomous vehicle control. An important characteristic of EI approaches is the necessity of a signal with good excitation properties to model the system, which is usually obtained by optimal input design approaches. Although this is a method that reproduces the actual behavior through a mathematical tool, it can ignore unmodeled features such as asymmetry or even constructive addictions. In this way, this work aims to construct a hybrid identification method through a mathematical model estimated in convolution with a deep neural network (DNN). The Gated recurrent unit (GRU) must be able to determine the states of the next instant based on the input and output of the theoretical model. The results are promising and suggest that this association is valid and capable of refining the outputs of a purely mathematical model.
更多查看译文
关键词
Hybrid identification, optimal input design, optimal parameter estimation, recurrent deep learning
AI 理解论文
溯源树
样例
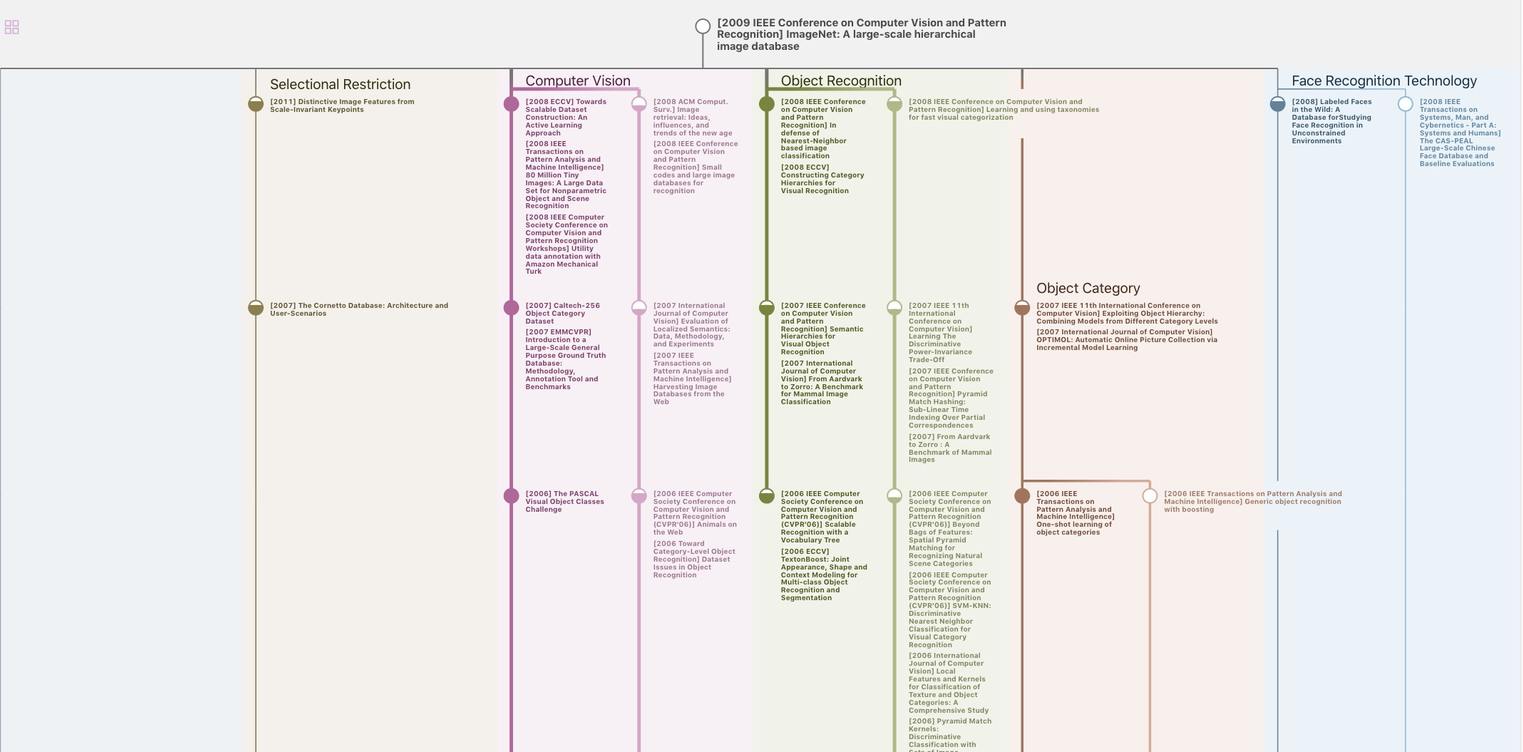
生成溯源树,研究论文发展脉络
Chat Paper
正在生成论文摘要