Role of Stain Normalization in Computational Pathology: Use Case in Metastatic Tissue Classification
MEDICAL IMAGING 2023(2023)
摘要
Machine learning algorithms have made strides in the classification of metastasis in tissue histopathology images. However, one of the major roadblocks faced by these algorithms is the difference in staining of tissue samples taken and scanned at different laboratories. Stain normalization works to standardize the color and intensity of stain patches to a reference image, hence bringing different laboratory stainings to a similar domain. We propose to compare different stain normalization methods in conjunction with color augmentation to evaluate the performance of combinations of techniques for binary classification of metastatic tissue slides taken from lymph nodes. We examine the accuracy, precision, recall, F1 score, and AUROC of a convolutional neural network (CNN) model trained and tested on images that have been normalized using the Macenko and Vahadane methods. Six different configurations that combine color augmentation and stain normalization were analyzed on the PatchCamelyon (PCAM) dataset consisting of over 300K images. Our analysis showed that the Macenko method of stain normalization improves model performance, and similarly, data augmentation shows general improvement, as the increased diversity amongst the data counters overfit in the model. Model accuracy with Macenko and color augmentation improved from baseline by 1.59% and F1 score for Macenko improved from baseline by 2.50%. The best performing combination was color augmentation with the Macenko method.
更多查看译文
关键词
Stain normalization, Image augmentation, Macenko Stain Normalization, Vahadane Stain Normalization, ResNet-18, PatchCameylon
AI 理解论文
溯源树
样例
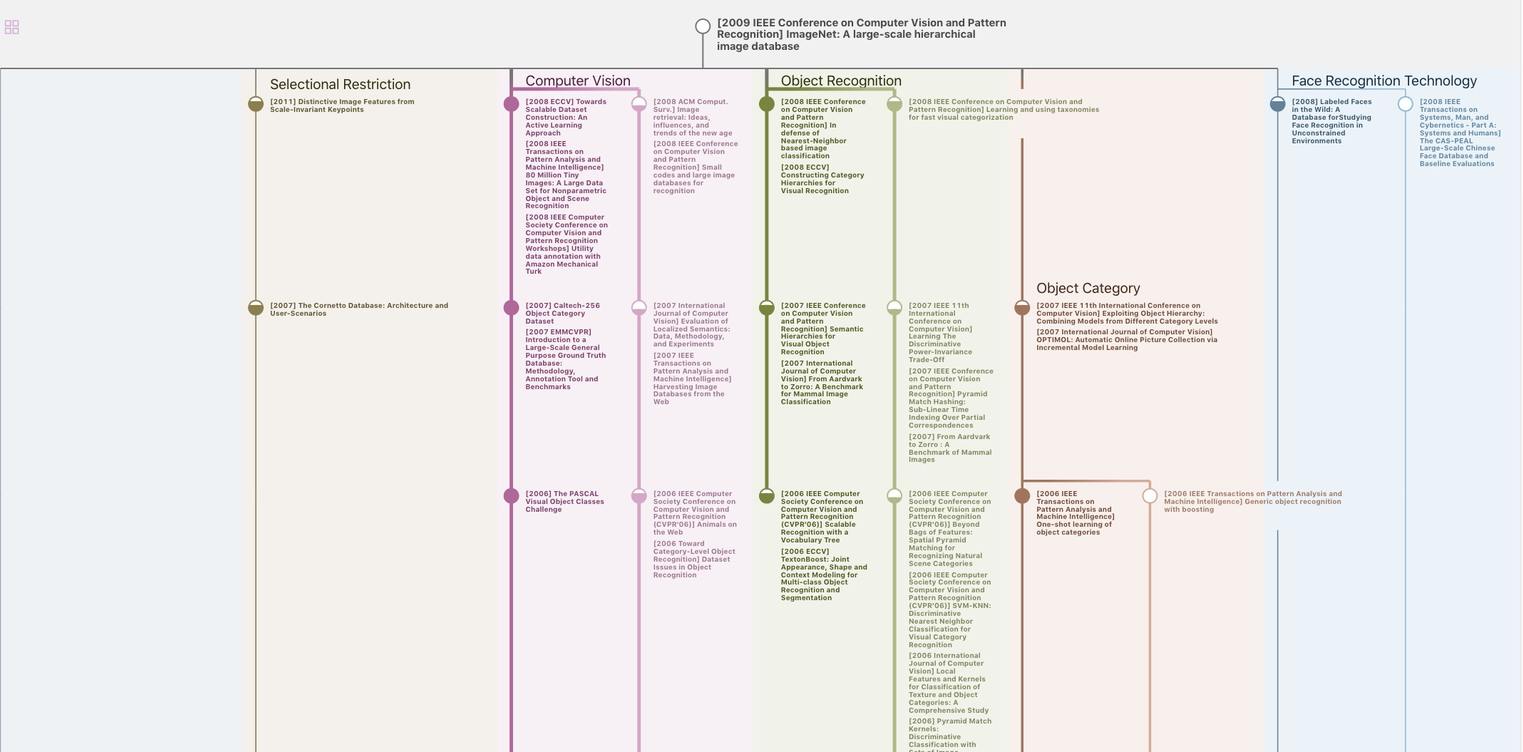
生成溯源树,研究论文发展脉络
Chat Paper
正在生成论文摘要