Resolving Impact of Technical and Biological Sources of Variability on Convolutional Neural Networks: Evaluating Chest X-ray Scans.
MEDICAL IMAGING 2023(2023)
摘要
Chest X-ray scans are subject to variations that can be technical or biological in nature. Technical variations are often on account of differences in type scanner types (e.g. x-ray energy, image pre-processing) whereas biological variations are those associated with differences among populations (e.g. race or gender). Recently, there has been a great deal of interest in the development and application of CNN-based deep learning (DL) algorithms to identify and characterize diseases such as tuberculosis on chest CT scans. However, a detailed assessment of DL networks in terms of resilience to technical and biological sources of variation separately has not been undertaken. These variations could decrease the generalizability of and could lead to bias within specific patient subgroups. In this study, we aimed to disentangle the sources of technical and biological variations in a CNN for the classification of chest X-rays. Therefore, the primary goal of this study was to understand whether, in the context of technical and biological variations, tailored and specific AI models might need to be created (i.e., gender-specific or machine-specific AI models). We evaluated three CNN models to classify technical variations (scanner types, dataset origin) and biological variations (patient sex, and race) using four publicly available Chest x-ray datasets, comprising a total of 871 235 patient scans: MIMIC, Chest-Xray8, CheXpert, and PadChest. Our results indicate that CNNs could distinguish scanner type (i.e. fixed from portable) from x-ray images with an accuracy of 99.9% (N=36 816). Similarly, patient race (e.g. black versus white) was classified with 92% accuracy (N=28 648). Saliency maps showed that the CNN focused on the patient's bones when attempting to predict the patient's race. These findings appear to suggest that the choice of the scanner, race, and gender may have an impact on the CNNs, and thus gender and race-specific models may be needed for diagnostic classification tasks.
更多查看译文
关键词
Deep learning, chest x-ray, self-reported gender classification, scanner type classification, self-reported race classification
AI 理解论文
溯源树
样例
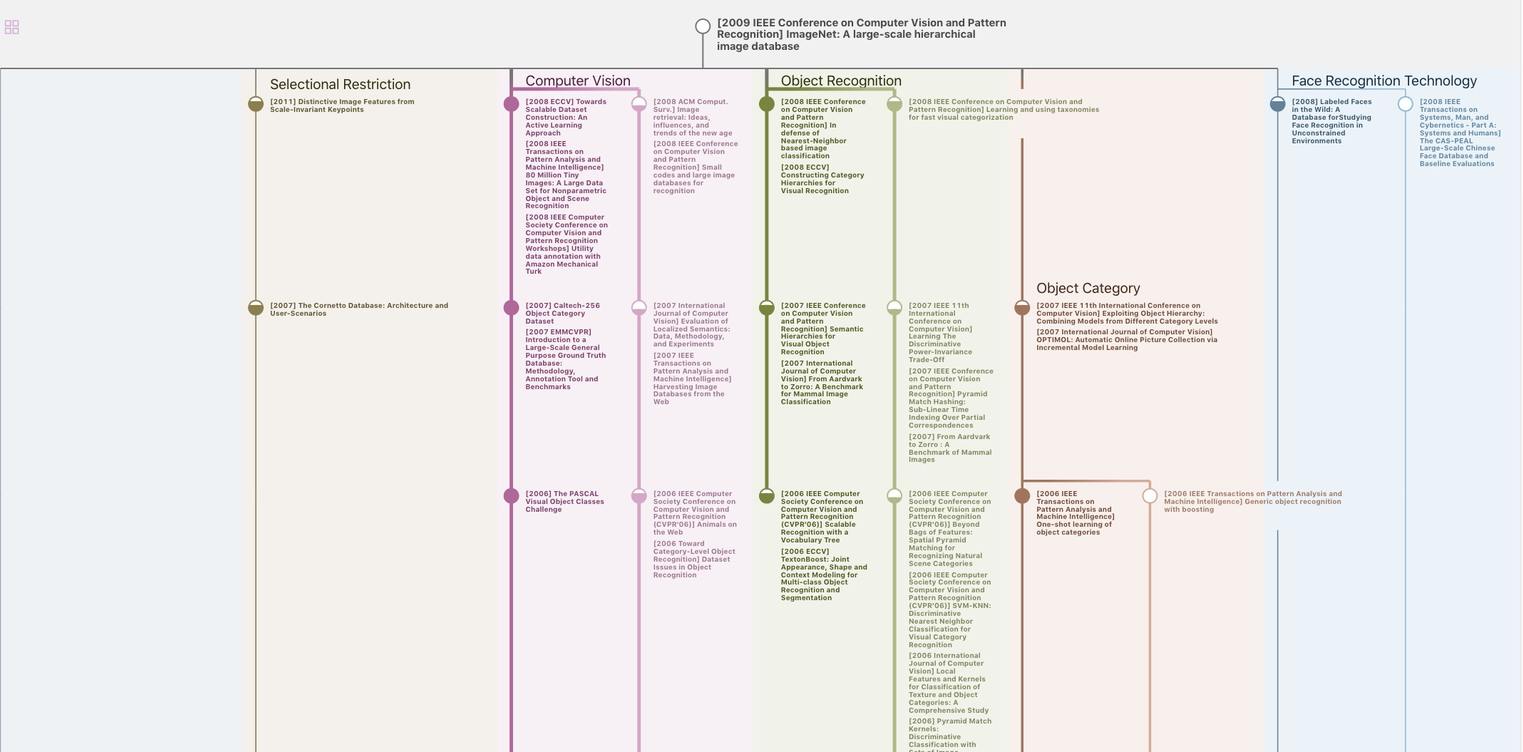
生成溯源树,研究论文发展脉络
Chat Paper
正在生成论文摘要