A machine learning approach targeting parameter estimation for plantfunctional type coexistence modeling using ELM-FATES (v2.0)
GEOSCIENTIFIC MODEL DEVELOPMENT(2023)
摘要
Tropical forest dynamics play a crucial role in the global carbon, water,and energy cycles. However, realistically simulating the dynamics ofcompetition and coexistence between different plant functional types (PFTs)in tropical forests remains a significant challenge. This study aims toimprove the modeling of PFT coexistence in the Functionally AssembledTerrestrial Ecosystem Simulator (FATES), a vegetation demography modelimplemented in the Energy Exascale Earth System Model (E3SM) land model(ELM), ELM-FATES. Specifically, we explore (1) whether plant traitrelationships established from field measurements can constrain ELM-FATESsimulations and (2) whether machine learning (ML)-based surrogate modelscan emulate the complex ELM-FATES model and optimize parameter selections toimprove PFT coexistence modeling. We conducted three ensembles of ELM-FATESexperiments at a tropical forest site near Manaus, Brazil. By comparing theensemble experiments without (Exp-CTR) and with (Exp-OBS) consideration ofobserved trait relationships, we found that accounting for theserelationships slightly improves the simulations of water, energy, and carbonvariables when compared to observations but degrades the simulation of PFTcoexistence. Using ML-based surrogate models trained on Exp-CTR, weoptimized the trait parameters in ELM-FATES and conducted another ensembleof experiments (Exp-ML) with these optimized parameters. The proportion ofPFT coexistence experiments significantly increased from 21 % in Exp-CTRto 73 % in Exp-ML. After filtering the experiments that allow for PFTcoexistence to agree with observations (within 15 % tolerance), 33 % ofthe Exp-ML experiments were retained, which is a significant improvementcompared to the 1.4 % in Exp-CTR. Exp-ML also accurately reproduces the annualmeans and seasonal variations in water, energy, and carbon fluxes and thefield inventory of aboveground biomass. This study represents areproducible method that utilizes machine learning to identify parametervalues that improve model fidelity against observations and PFT coexistencein vegetation demography models for diverse ecosystems. Our study alsosuggests the need for new mechanisms to enhance the robust simulation ofcoexisting plants in ELM-FATES and has significant implications formodeling the response and feedbacks of ecosystem dynamics to climate change.
更多查看译文
AI 理解论文
溯源树
样例
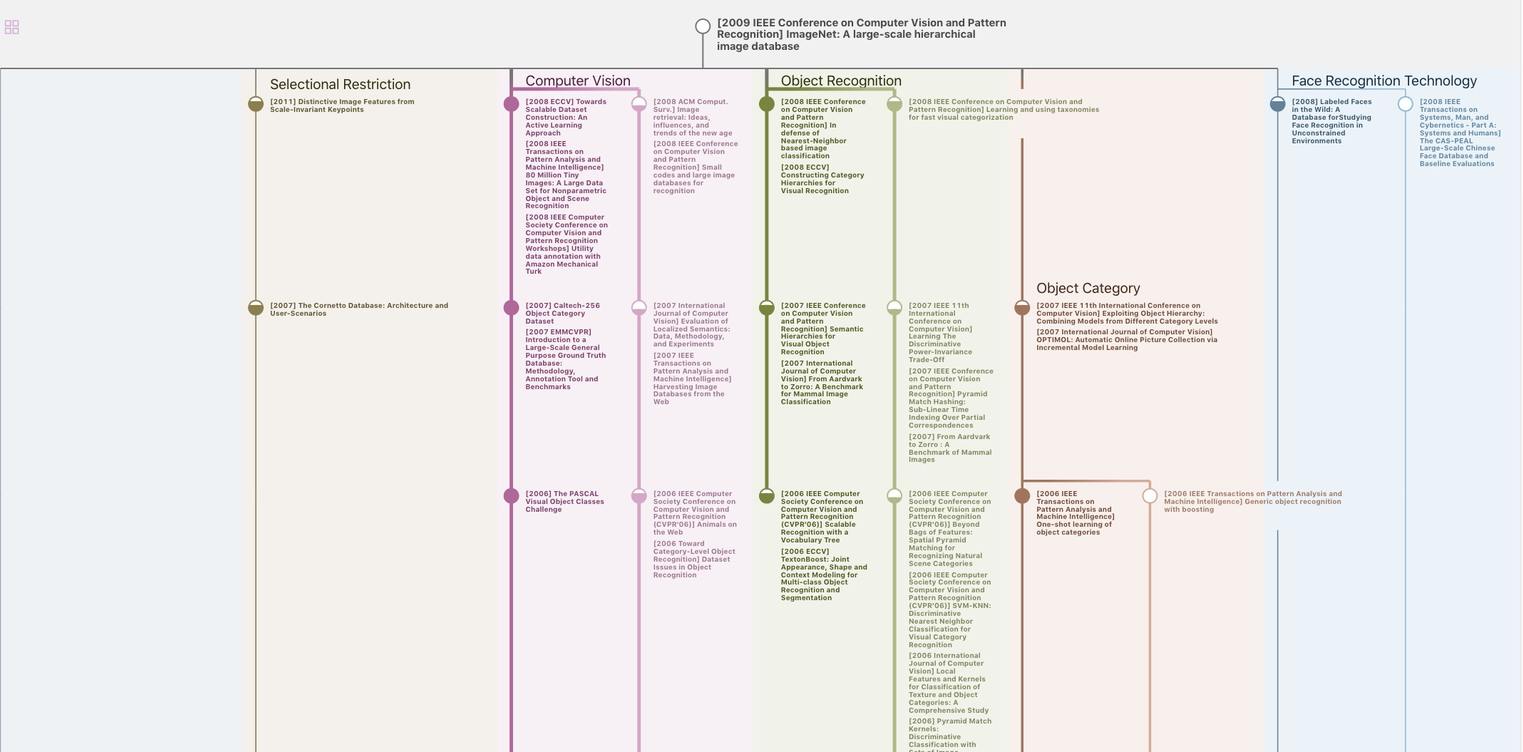
生成溯源树,研究论文发展脉络
Chat Paper
正在生成论文摘要