Boosting deep cross-modal retrieval hashing with adversarially robust training
APPLIED INTELLIGENCE(2023)
摘要
Deep hashing methods effectively enhance the performance of conventional machine learning retrieval models, particularly in visual medium evolving cross-modal retrieval tasks, by relying on the outstanding feature extraction ability of deep neural networks (DNNs). The state-of-the-art deep hashing research focuses on designing prominent models by employing DNNs to discover semantic information from different modalities of data and execute relevant information retrieval tasks. However, the robustness attribute considered essential for reliable DNN model design has limited concerns on deep hashing models. In this article, we present an end-to-end adversarial training framework for cross-modal retrieval. Our framework leverages a projected gradient descent(PGD)-based method to generate adversarial samples, which are then combined with normal samples to achieve robust training. Our approach addresses the vulnerability issues of existing cross-modal retrieval models and fills the gap in retrieval task design. We conduct extensive experiments and compare our model with state-of-the-art cross-modal retrieval models on three benchmark datasets to verify that our model can effectively boost the performance of deep hashing retrieval models on cross-modal retrieval . This work highlights the effectiveness of adversarial training in efficient deep hashing model design.
更多查看译文
关键词
Cross-modal retrieval, Adversarial training, Deep hashing model, Deep neural network
AI 理解论文
溯源树
样例
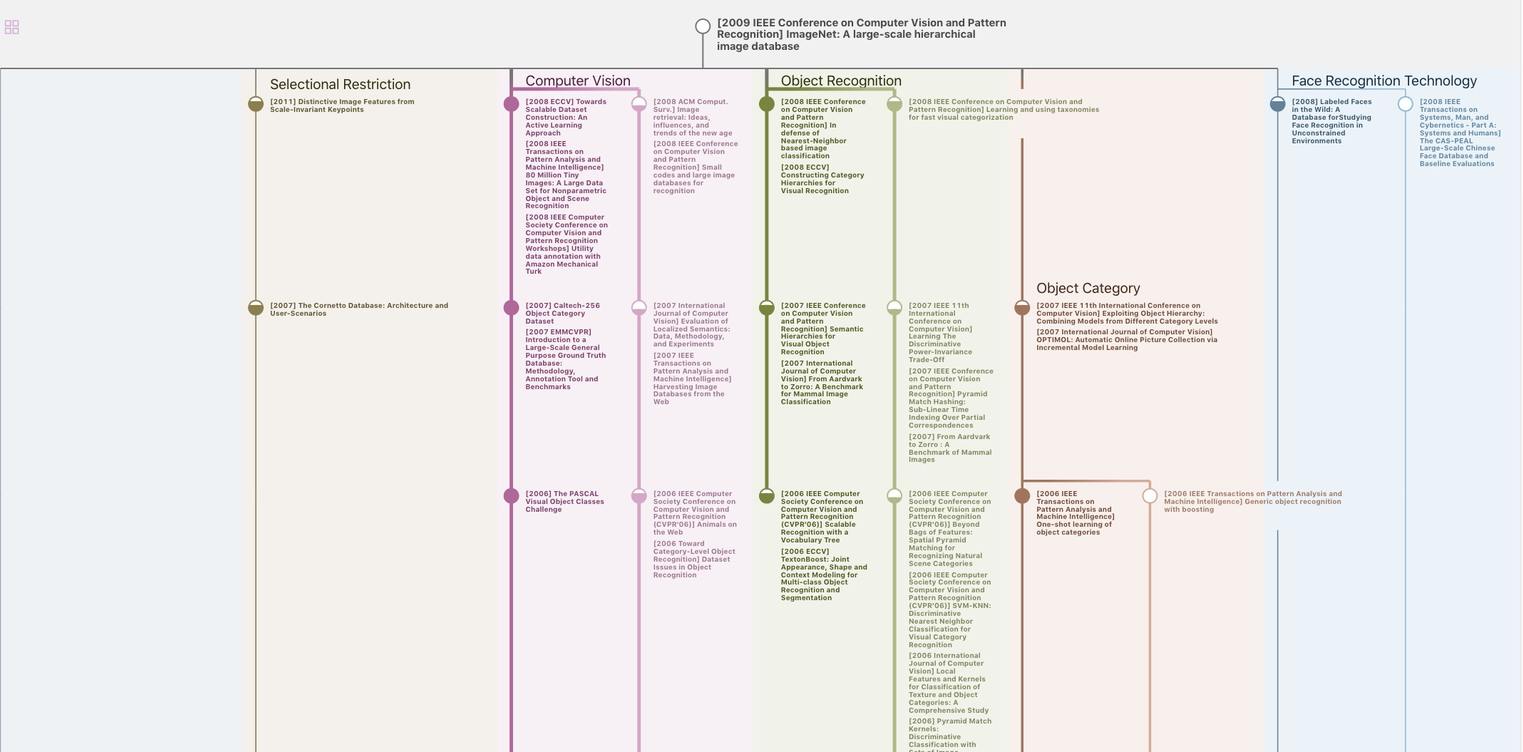
生成溯源树,研究论文发展脉络
Chat Paper
正在生成论文摘要