Identifying Behavioral Factors Leading to Differential Polarization Effects of Adversarial Botnets
APPLIED COMPUTING REVIEW(2023)
摘要
In this paper, we utilize a Twitter dataset collected between December 8, 2021 and February 18, 2022, during the lead-up to the 2022 Russian invasion of Ukraine. Our aim is to design a data processing pipeline featuring a high-accuracy Graph Convolutional Network (GCN) based political camp classifier, a botnet detection algorithm, and a robust measure of botnet effects. Our experiments reveal that while the pro-Russian botnet contributes significantly to network polarization, the pro-Ukrainian botnet contributes with moderating effects. To understand the factors leading to these different effects, we analyze the interactions between the botnets and the users, distinguishing between barrier-crossing users, who navigate across different political camps, and barrier-bound users, who remain within their own camps. We observe that the pro-Russian botnet amplifies the barrier-bound partisan users within their own camp most of the time. In contrast, the pro-Ukrainian botnet amplifies the barrier-crossing users on their own camp alongside themselves for the majority of the time.
更多查看译文
关键词
Social Networks, Botnet Detection, Political Camp Classification, Feature Propagation, Polarization Measures
AI 理解论文
溯源树
样例
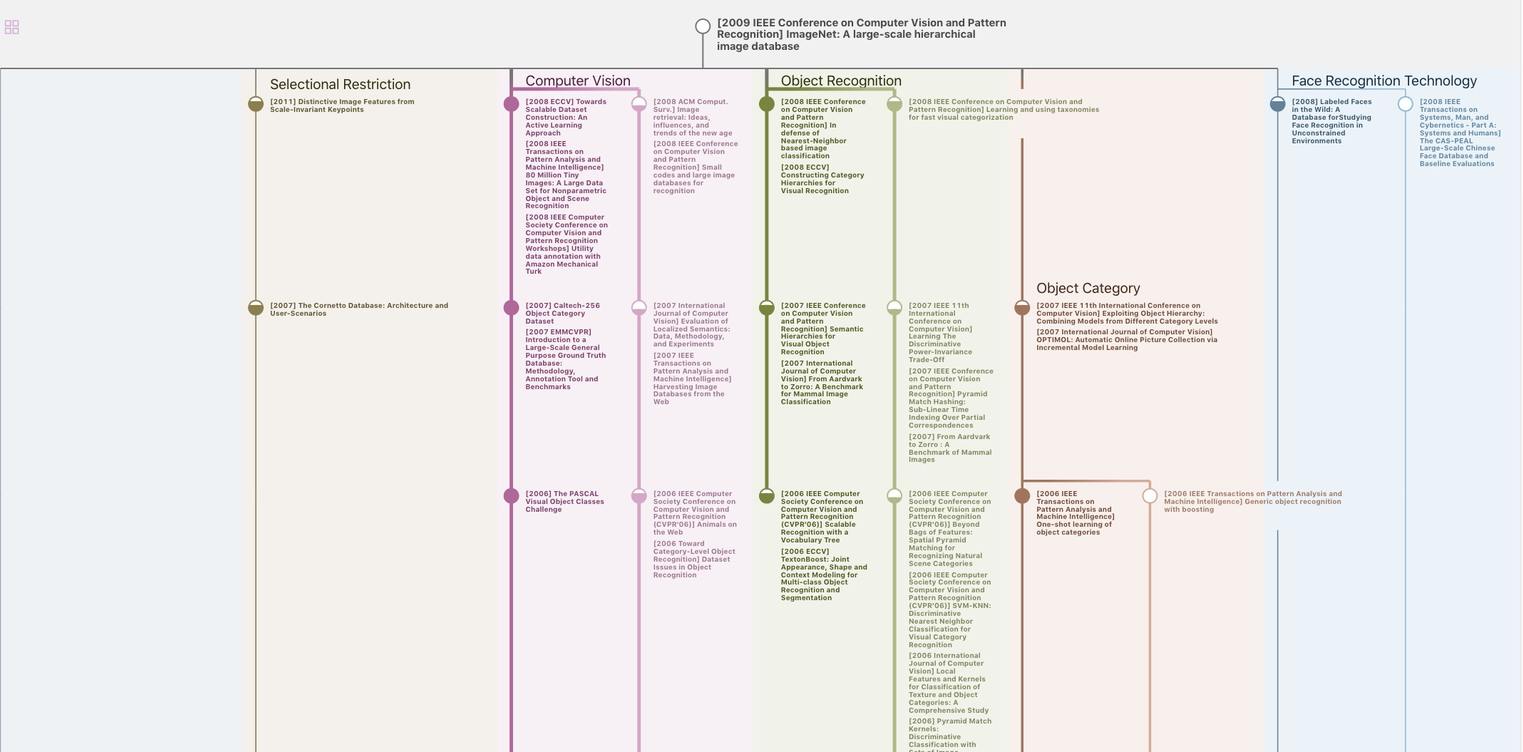
生成溯源树,研究论文发展脉络
Chat Paper
正在生成论文摘要