An active learning method based on result quality evaluation for printed circuit board computed tomography image segmentation
IET IMAGE PROCESSING(2023)
摘要
Element detection is a key step in non-destructive testing of printed circuit board (PCB) based on computed tomography (CT). In recent years, some image segmentation methods based on deep learning have shown great potential in the element segmentation task of PCB CT images, and greatly improved the efficiency and accuracy. However, since image segmentation is based on pixel-level classification, the annotation of training data is difficult and costly. Aiming at this problem, the authors proposed a new active learning method based on the integration of relevant information about segmentation tasks and data variance. In this method, the Result Quality Evaluation Module (RQEM) proposed by us is used to generate task-related information, and an adversarial network is used to generate the difference information between samples and the initial labelled data. Finally, the two parts of information are fused and used as the standard of data selecting. In the PCB CT image element segmentation task, the authors only need to select 12.7% of the whole training set with their proposed method to make Mean Intersection Over Union (MIOU) reach 79.7, which has reached 95% of the optimal performance of 83.7. The in-depth analysis also verifies the effectiveness and stability of the authors' method.
更多查看译文
关键词
computer vision,image segmentation,inverse problems,non-destructive testing
AI 理解论文
溯源树
样例
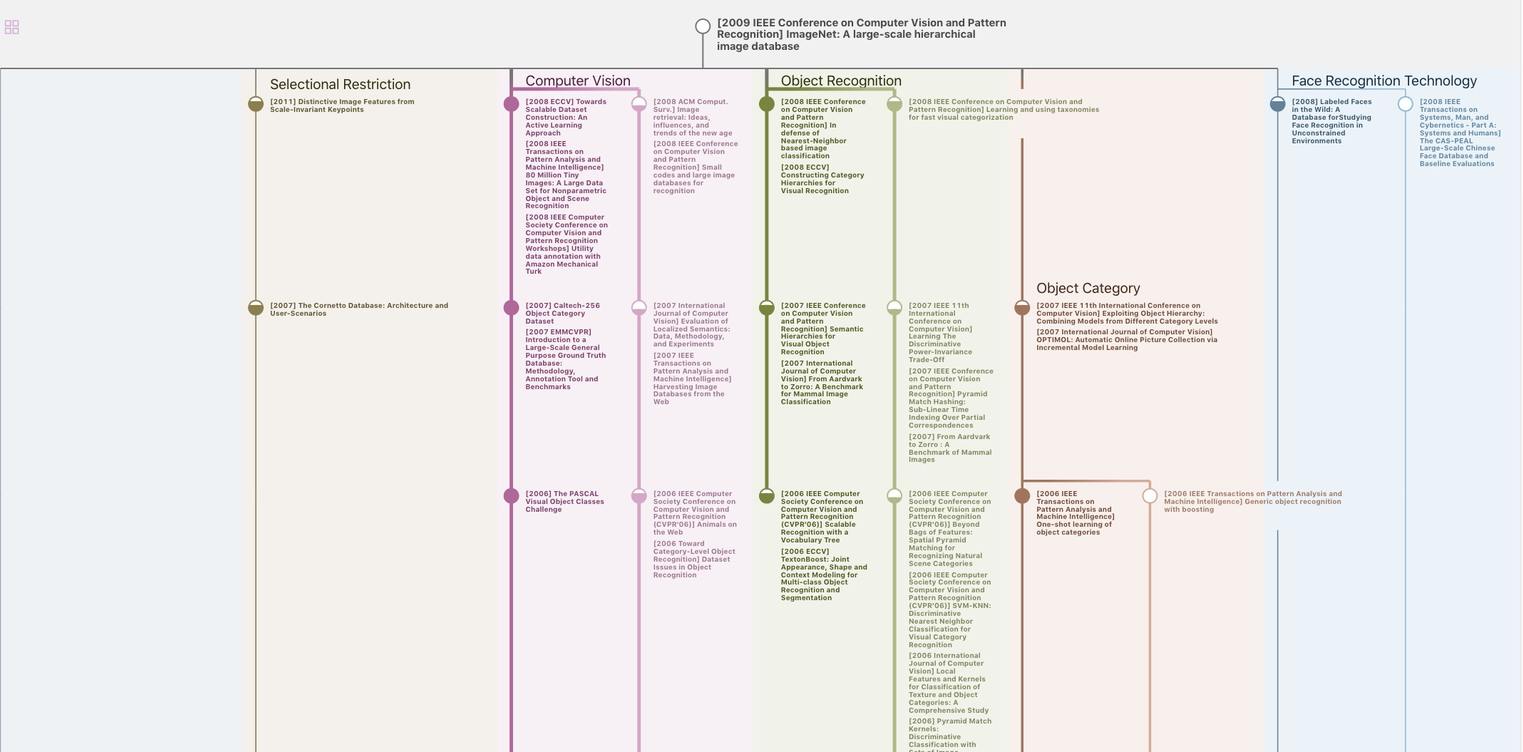
生成溯源树,研究论文发展脉络
Chat Paper
正在生成论文摘要