An improved multi-task least squares twin support vector machine
ANNALS OF MATHEMATICS AND ARTIFICIAL INTELLIGENCE(2023)
摘要
In recent years, multi-task learning (MTL) has become a popular field in machine learning and has a key role in various domains. Sharing knowledge across tasks in MTL can improve the performance of learning algorithms and enhance their generalization capability. A new approach called the multi-task least squares twin support vector machine (MTLS-TSVM) was recently proposed as a least squares variant of the direct multi-task twin support vector machine (DMTSVM). Unlike DMTSVM, which solves two quadratic programming problems, MTLS-TSVM solves two linear systems of equations, resulting in a reduced computational time. In this paper, we propose an enhanced version of MTLS-TSVM called the improved multi-task least squares twin support vector machine (IMTLS-TSVM). IMTLS-TSVM offers a significant advantage over MTLS-TSVM by operating based on the empirical risk minimization principle, which allows for better generalization performance. The model achieves this by including regularization terms in its objective function, which helps control the model’s complexity and prevent overfitting. We demonstrate the effectiveness of IMTLS-TSVM by comparing it to several single-task and multi-task learning algorithms on various real-world data sets. Our results highlight the superior performance of IMTLS-TSVM in addressing multi-task learning problems.
更多查看译文
关键词
Least squares, Multi-task learning, Twin support vector machine, Multi-task twin support vector machine, Quadratic programming problems
AI 理解论文
溯源树
样例
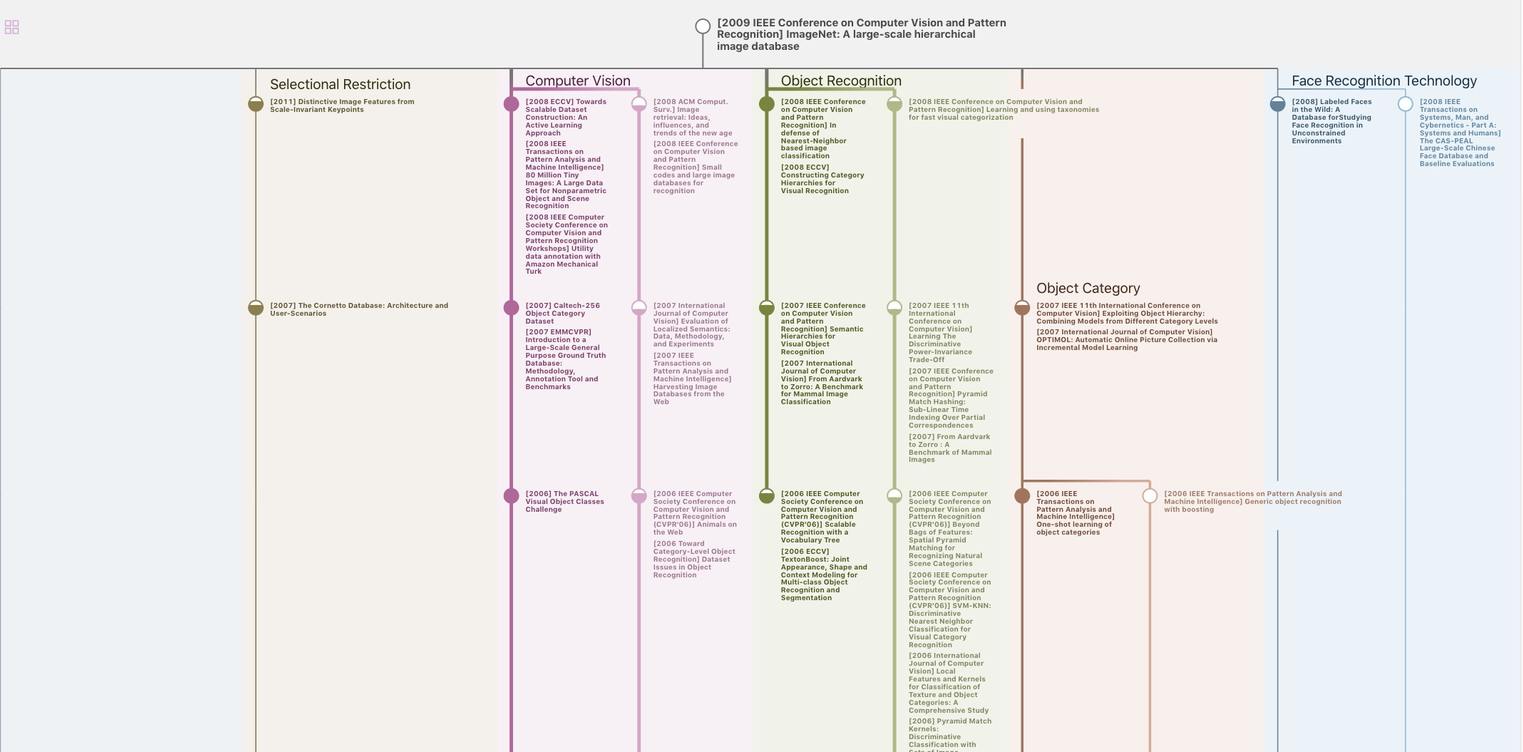
生成溯源树,研究论文发展脉络
Chat Paper
正在生成论文摘要