Predicting secondary school student performance using a double particle swarm optimization-based categorical boosting model
ENGINEERING APPLICATIONS OF ARTIFICIAL INTELLIGENCE(2023)
摘要
Knowing the potential students who will fail the final exam at early stages is very challenging but important for the decision-makers in the educational institutions to take proper actions to prevent them from failure. To accurately predict the secondary school student performance, we propose a double particle swarm optimization (PSO)-based categorical boosting (P2CatBoost) model based on the demographic, school period grades, and social/school related features. Considering the machine learning models are sensitive to their hyper-parameter settings, we introduce the PSO to optimize the fitness function. In addition, the threshold of a standard binary classification task is 0.5, which might not be the optimal value in real-world applications. Thus, we optimize this threshold by the PSO. To evaluate the performance of our proposed model, two datasets downloaded from the University of California, Irvine Repository and the Kaggle, respectively, are used. The experimental results showed that our proposed P2CatBoost has the best performance in terms of all the metrics used. Our proposed P2CatBoost has the best accuracy of 96.62% and 94.45% for the final grade prediction of the Mathematics and Portuguese courses, respectively. In addition, our proposed model outperforms the other models under comparison from 4.5% to 8.3%. The statistical analyses verify that our P2CatBoost can significantly outperform the comparing models. These results confirm the effectiveness of our double PSO for improving the performance of student performance prediction, indicating our proposed model could be a useful tool in educational institutions to improve the quality of education.
更多查看译文
关键词
secondary school student performance,optimization-based
AI 理解论文
溯源树
样例
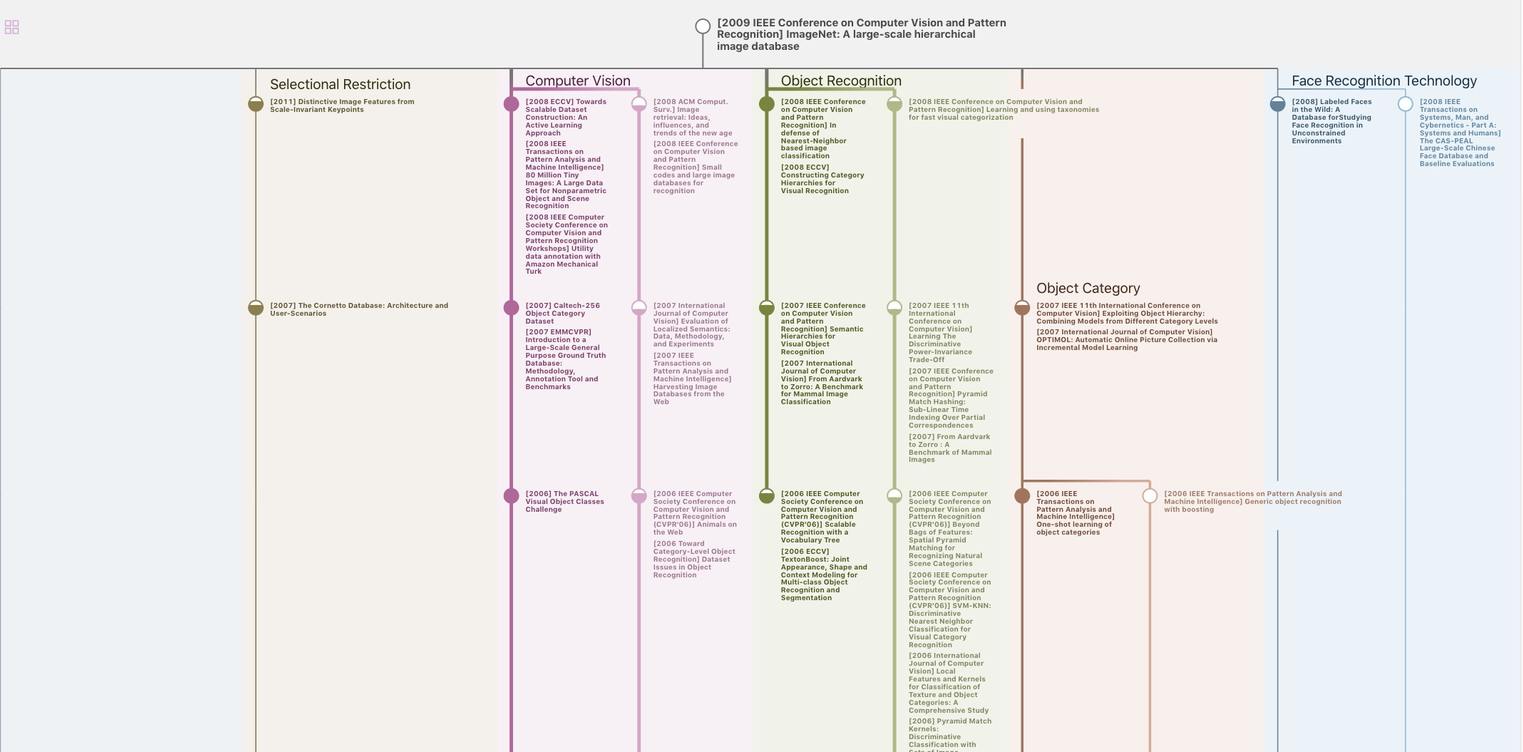
生成溯源树,研究论文发展脉络
Chat Paper
正在生成论文摘要