PL-Net: towards deep learning-based localization for underwater terrain
NEURAL COMPUTING & APPLICATIONS(2023)
摘要
Underwater terrain matching localization is a method for achieving precise localization by using the features of submarine topography to perform relevant matching operations. Existing underwater terrain matching algorithms suffer from issues such as limited application range, poor real-time performance, and low localization accuracy because of the variable carrier motion state, insignificant undulatory features of underwater terrain, and low underwater terrain data measurement stability. To solve the above problems, we present PL-Net, a novel learning-based underwater terrain matching localization method. First, we extract the terrain’s keypoints to avoid the complexity of large-scale computation and improve the algorithm’s real-time performance. Then, the pretrained deep neural network automatically extracts the high-level terrain features, avoiding the manual setting of feature extraction patterns, reducing the probability of localization failure caused by environmental and equipment factors, and improving the algorithm’s robustness. Finally, we improve the localization accuracy by adding a self-attention mechanism that enables the network to adjust the weights of each component and pay more attention to the regions with significant features. We conducted a series of tests to validate the proposed method. The results indicate that our method significantly outperforms other methods, indicating that our method can achieve accurate and real-time localization in a wide range of underwater environments.
更多查看译文
关键词
Underwater localization,Terrain matching,3D point cloud,Deep learning
AI 理解论文
溯源树
样例
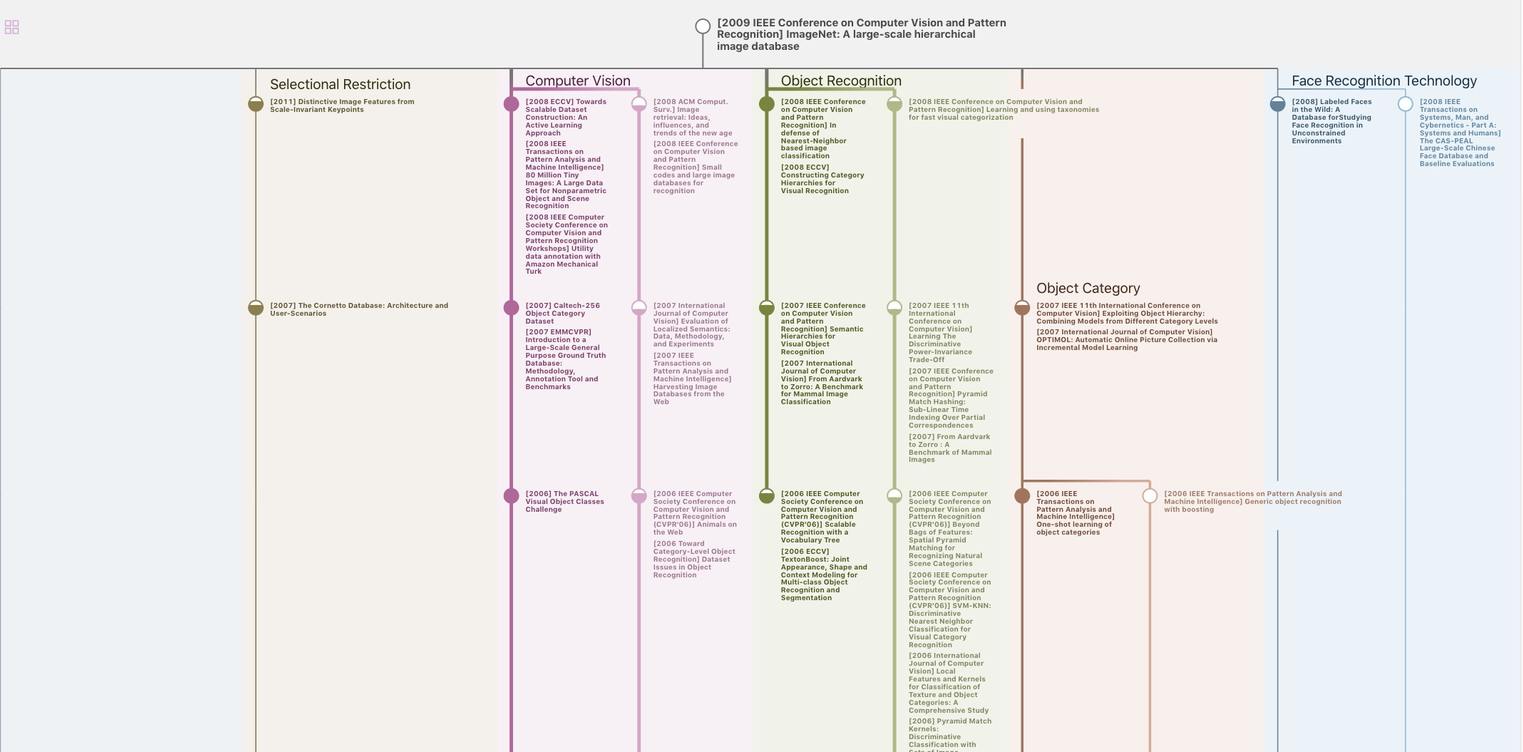
生成溯源树,研究论文发展脉络
Chat Paper
正在生成论文摘要