A CNN Compression Method via Dynamic Channel Ranking Strategy
INTERNATIONAL JOURNAL OF COMPUTATIONAL INTELLIGENCE AND APPLICATIONS(2023)
摘要
In recent years, the rapid development of mobile devices and embedded system raises a demand for intelligent models to address increasingly complicated problems. However, the complexity of the structure and extensive parameters press significantly on efficiency, storage space, and energy consumption. Additionally, the explosive growth of tasks with enormous model structures and parameters makes it impossible to compress models manually. Thus, a standardized and effective model compression solution achieving lightweight neural networks is established as an urgent demand by the industry. Accordingly, Dynamic Channel Ranking Strategy (DCRS) method is proposed to compress deep convolutional neural networks. DCRS selects channels with high contribution of each prunable layer according to compression ratio searched by reinforcement learning agent. Compared with current model compression methods, DCRS efficaciously applies various channel ranking strategies on prunable layers. Experiments indicate with a 50% compression ratio, compressed MobileNet achieved 70.62% top1 and 88.2% top5 accuracy on ImageNet, and compressed ResNet achieved 92.03% accuracy on CIFAR-10. DCRS reduces more FLOPS in these neural networks. The compressed model achieves the best Top-1 and Top-5 accuracy on ResNet50, the best Top-1 accuracy on MobilNetV1.
更多查看译文
AI 理解论文
溯源树
样例
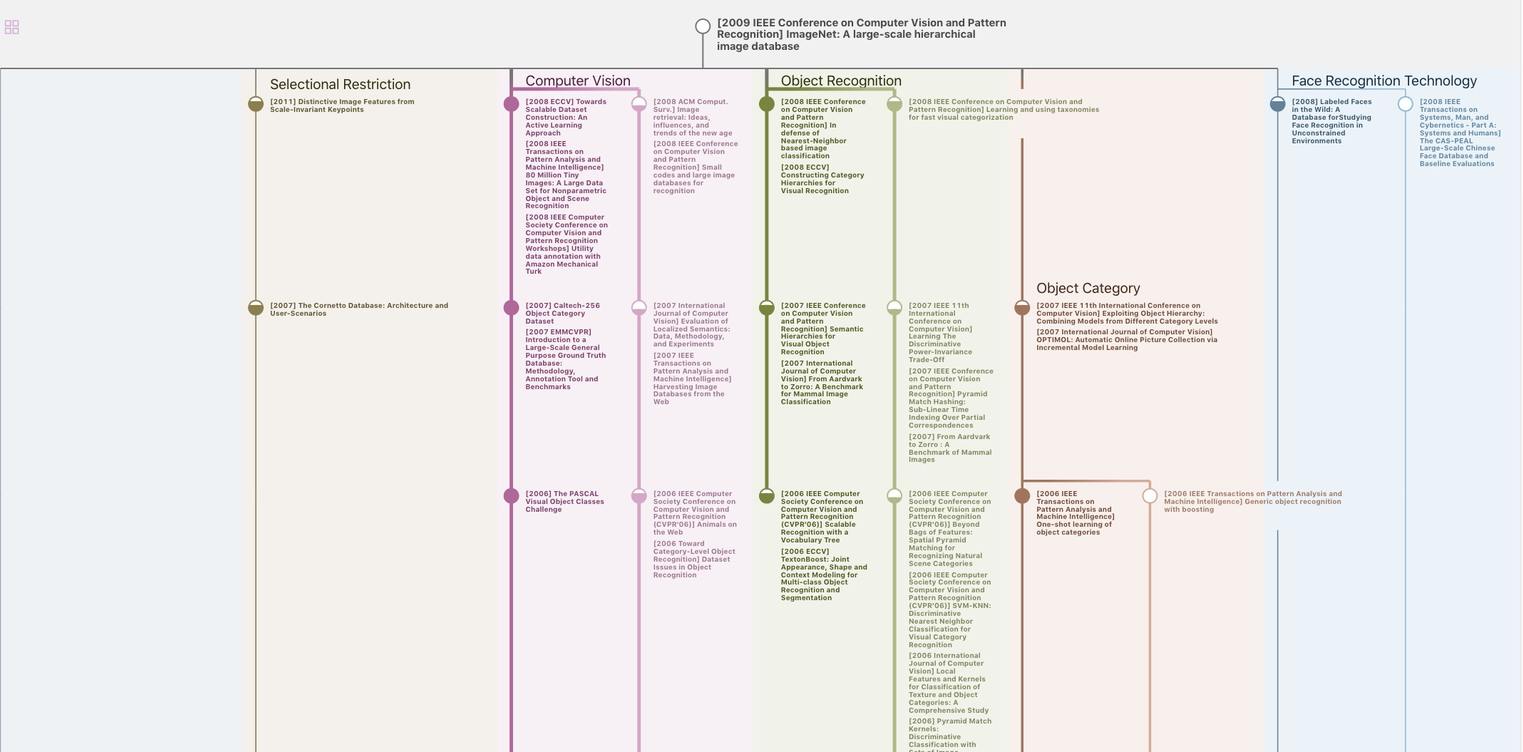
生成溯源树,研究论文发展脉络
Chat Paper
正在生成论文摘要